Clinical Applications of Machine Learning in the Management of Intraocular Cancers: A Narrative Review
INVESTIGATIVE OPHTHALMOLOGY & VISUAL SCIENCE(2023)
摘要
PURPOSE. There is great promise in use of machine learning (ML) for the diagnosis, prognosis, and treatment of various medical conditions in ophthalmology and beyond. Applications of ML for ocular neoplasms are in early development and this review synthesizes the current state of ML in ocular oncology. METHODS. We queried PubMed and Web of Science and evaluated 804 publications, excluding nonhuman studies. Metrics on ML algorithm performance were collected and the Prediction model study Risk Of Bias ASsessment Tool was used to evaluate bias. We report the results of 63 unique studies. RESULTS. Research regarding ML applications to intraocular cancers has leveraged multiple algorithms and data sources. Convolutional neural networks (CNNs) were one of the most commonly used ML algorithms and most work has focused on uveal melanoma and retinoblastoma. The majority of ML models discussed here were developed for diagnosis and prognosis. Algorithms for diagnosis primarily leveraged imaging (e.g., optical coherence tomography) as inputs, whereas those for prognosis leveraged combinations of gene expression, tumor characteristics, and patient demographics. CONCLUSIONS. ML has the potential to improve the management of intraocular cancers. Published ML models perform well, but were occasionally limited by small sample sizes owing to the low prevalence of intraocular cancers. This could be overcome with synthetic data enhancement and low-shot ML techniques. CNNs can be integrated into existing diagnostic workflows, while non-neural networks perform well in determining prognosis.
更多查看译文
关键词
ocular oncology,machine learning,artificial intelligence,uveal melanoma,retinoblastoma
AI 理解论文
溯源树
样例
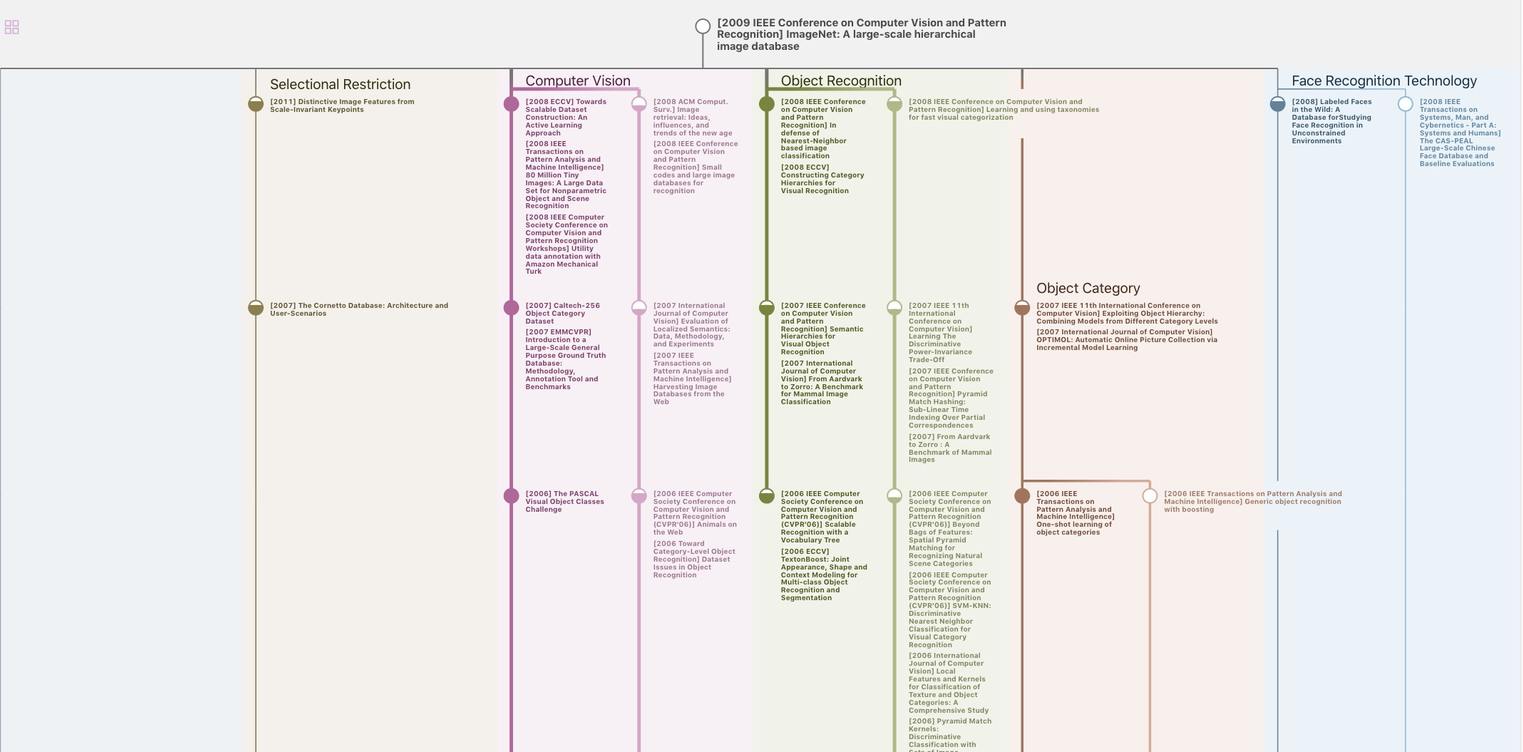
生成溯源树,研究论文发展脉络
Chat Paper
正在生成论文摘要