A Machine Learning Method to Identify the Risk Factors for Liver Fibrosis Progression in Nonalcoholic Steatohepatitis
Digestive diseases and sciences(2023)
摘要
Aim Nonalcoholic fatty liver disease (NAFLD) is a silent epidemy that has become the most common chronic liver disease worldwide. Nonalcoholic steatohepatitis (NASH) is an advanced stage of NAFLD, which is linked to a high risk of cirrhosis and hepatocellular carcinoma. The aim of this study is to develop a predictive model to identify the main risk factors associated with the progression of hepatic fibrosis in patients with NASH. Methods A database from a multicenter retrospective cross-sectional study was analyzed. A total of 215 patients with NASH biopsy-proven diagnosed were collected. NAFLD Activity Score and Kleiner scoring system were used to diagnose and staging these patients. Noninvasive tests (NITs) scores were added to identify which one were more reliable for follow-up and to avoid biopsy. For analysis, different Machine Learning methods were implemented, being the eXtreme Gradient Booster (XGB) system the proposed algorithm to develop the predictive model. Results The most important variable in this predictive model was High-density lipoprotein (HDL) cholesterol, followed by systemic arterial hypertension and triglycerides (TG). NAFLD Fibrosis Score (NFS) was the most reliable NIT. As for the proposed method, XGB obtained higher results than the second method, K-Nearest Neighbors, in terms of accuracy (95.05 vs. 90.42) and Area Under the Curve (0.95 vs. 0.91). Conclusions HDL cholesterol, systemic arterial hypertension, and TG were the most important risk factors for liver fibrosis progression in NASH patients. NFS is recommended for monitoring and decision making.
更多查看译文
关键词
Nonalcoholic fatty liver disease, Nonalcoholic steatohepatitis, Liver fibrosis, Machine learning
AI 理解论文
溯源树
样例
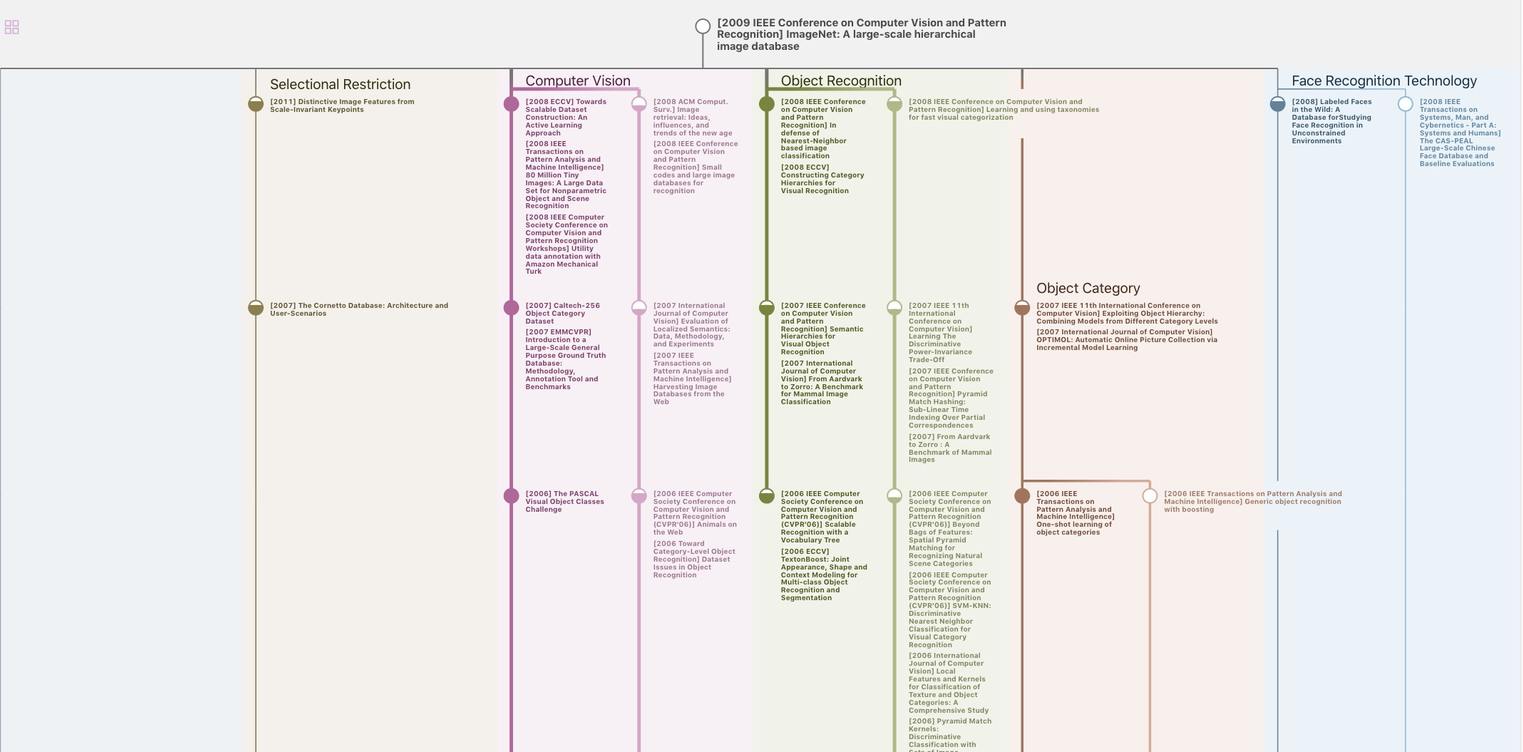
生成溯源树,研究论文发展脉络
Chat Paper
正在生成论文摘要