Placement of Parameter Server in Wide Area Network Topology for Geo-Distributed Machine Learning
JOURNAL OF COMMUNICATIONS AND NETWORKS(2023)
摘要
learning (ML) is extensively used in a wide range of real-world applications that require data all around world to pursue high accuracy of a global model. Unfortunately, it is impossible to transmit all gathered raw data to a central data center for training due to data privacy, data sovereignty and high communication cost. This brings the idea of geodistributed machine learning (Geo-DML), which completes the training of the global ML model across multiple data centers with the bottleneck of high communication cost over the limited wide area networks (WAN) bandwidth. In this paper, we study on the problem of parameter server (PS) placement in PS architecture for communication efficiency of Geo-DML. Our optimization aims to select an appropriate data center as the PS for global training algorithm based on the communication cost. We prove the PS placement problem is NP-hard. Further, we develop an approximation algorithm to solve the problem using the randomized rounding method. In order to validate the performance of our proposed algorithm, we conduct large-scale simulations, and the simulation results on two typical carrier network topologies show that our proposed algorithm can reduce the communication cost up to 61.78% over B4 topology and 21.78% over Internet2 network topology.
更多查看译文
关键词
Geo-distributed machine learning,routing,wide area networks
AI 理解论文
溯源树
样例
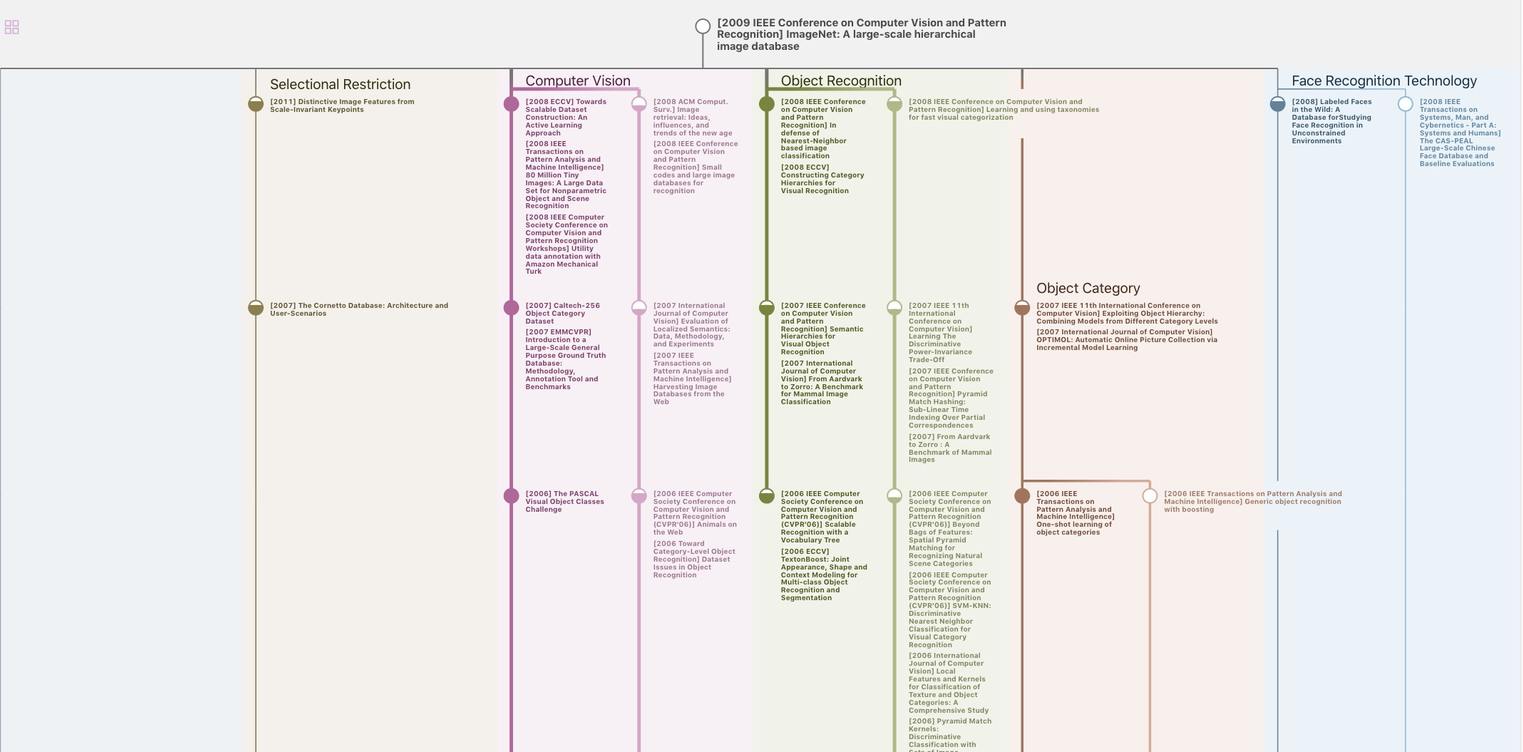
生成溯源树,研究论文发展脉络
Chat Paper
正在生成论文摘要