Remote sensing tree classification with a multilayer perceptron
crossref(2018)
Abstract
To accelerate scientific progress on remote tree classification—as well as biodiversity and ecology sampling—The National Institute of Science and Technology created a community-based competition where scientists were invited to contribute informatics methods for classifying tree species and genus using crown-level images of trees. We predicted tree species and genus at the pixel level using hyperspectral and LIDAR observations. We compared three algorithms that have been implemented extensively across a broad range of research applications: support vector machines, random forests, and multilayer perceptron. At the pixel level, the multilayer perceptron algorithm predicted species or genus with high accuracy (92.7 and 95.9%, respectively) on the training data and performed better than the other algorithms (85.8-93.5%). This indicates promise for the use of the MLP algorithm for tree-species classification and coincides with a growing body of research in which neural network-based algorithms outperform other types of classification algorithms for machine vision. To aggregate patterns across the images, we used an ensemble approach that averages the pixel-level outputs of the MLP algorithm to predict species at the crown level. The accuracy of these predictions on the test set was 68.8% for species.
MoreTranslated text
AI Read Science
Must-Reading Tree
Example
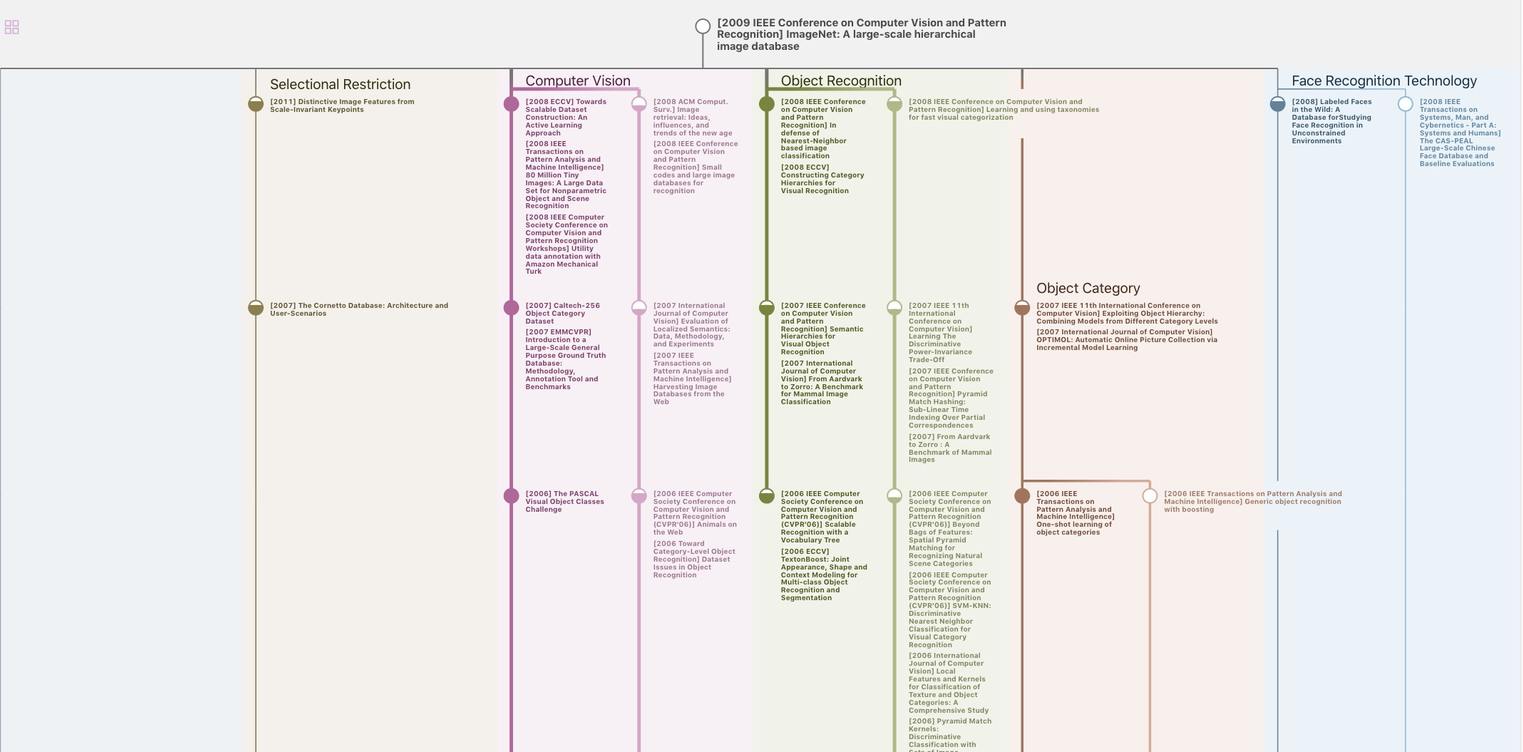
Generate MRT to find the research sequence of this paper
Chat Paper
Summary is being generated by the instructions you defined