Risk stratification of gallbladder masses by machine learning-based ultrasound radiomics models: a prospective and multi-institutional study
European radiology(2023)
摘要
Objective This study aimed to evaluate the diagnostic performance of machine learning (ML)–based ultrasound (US) radiomics models for risk stratification of gallbladder (GB) masses. Methods We prospectively examined 640 pathologically confirmed GB masses obtained from 640 patients between August 2019 and October 2022 at four institutions. Radiomics features were extracted from grayscale US images and germane features were selected. Subsequently, 11 ML algorithms were separately used with the selected features to construct optimum US radiomics models for risk stratification of the GB masses. Furthermore, we compared the diagnostic performance of these models with the conventional US and contrast-enhanced US (CEUS) models. Results The optimal XGBoost-based US radiomics model for discriminating neoplastic from non-neoplastic GB lesions showed higher diagnostic performance in terms of areas under the curves (AUCs) than the conventional US model (0.822–0.853 vs. 0.642–0.706, p < 0.05) and potentially decreased unnecessary cholecystectomy rate in a speculative comparison with performing cholecystectomy for lesions sized over 10 mm (2.7–13.8% vs. 53.6–64.9%, p < 0.05) in the validation and test sets. The AUCs of the XGBoost-based US radiomics model for discriminating carcinomas from benign GB lesions were higher than the conventional US model (0.904–0.979 vs. 0.706–0.766, p < 0.05). The XGBoost-US radiomics model performed better than the CEUS model in discriminating GB carcinomas (AUC: 0.995 vs. 0.902, p = 0.011). Conclusions The proposed ML-based US radiomics models possess the potential capacity for risk stratification of GB masses and may reduce the unnecessary cholecystectomy rate and use of CEUS. Clinical relevance statement The machine learning-based ultrasound radiomics models have potential for risk stratification of gallbladder masses and may potentially reduce unnecessary cholecystectomies. Key Points • The XGBoost-based US radiomics models are useful for the risk stratification of GB masses. • The XGBoost-based US radiomics model is superior to the conventional US model for discriminating neoplastic from non-neoplastic GB lesions and may potentially decrease unnecessary cholecystectomy rate for lesions sized over 10 mm in comparison with the current consensus guideline. • The XGBoost-based US radiomics model could overmatch CEUS model in discriminating GB carcinomas from benign GB lesions.
更多查看译文
关键词
Ultrasound,Gallbladder masses,Machine learning,Radiomics,Risk stratification
AI 理解论文
溯源树
样例
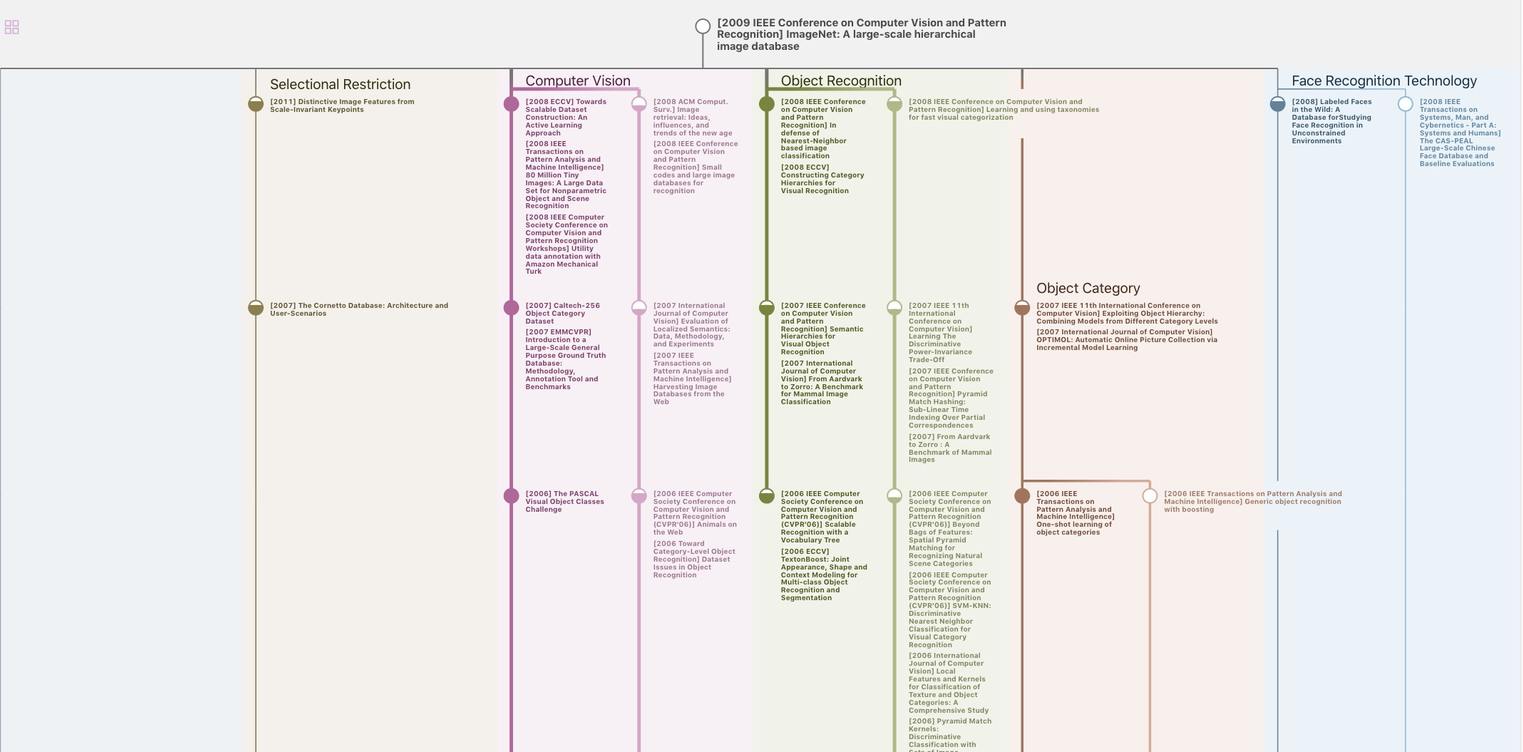
生成溯源树,研究论文发展脉络
Chat Paper
正在生成论文摘要