Artificial Intelligence for Detecting Acute Fractures in Patients Admitted to an Emergency Department: Real-Life Performance of Three Commercial Algorithms.
Academic radiology(2023)
摘要
RATIONALE AND OBJECTIVES:Interpreting radiographs in emergency settings is stressful and a burden for radiologists. The main objective was to assess the performance of three commercially available artificial intelligence (AI) algorithms for detecting acute peripheral fractures on radiographs in daily emergency practice.
MATERIALS AND METHODS:Radiographs were collected from consecutive patients admitted for skeletal trauma at our emergency department over a period of 2 months. Three AI algorithms-SmartUrgence, Rayvolve, and BoneView-were used to analyze 13 body regions. Four musculoskeletal radiologists determined the ground truth from radiographs. The diagnostic performance of the three AI algorithms was calculated at the level of the radiography set. Accuracies, sensitivities, and specificities for each algorithm and two-by-two comparisons between algorithms were obtained. Analyses were performed for the whole population and for subgroups of interest (sex, age, body region).
RESULTS:A total of 1210 patients were included (mean age 41.3 ± 18.5 years; 742 [61.3%] men), corresponding to 1500 radiography sets. The fracture prevalence among the radiography sets was 23.7% (356/1500). Accuracy was 90.1%, 71.0%, and 88.8% for SmartUrgence, Rayvolve, and BoneView, respectively; sensitivity 90.2%, 92.6%, and 91.3%, with specificity 92.5%, 70.4%, and 90.5%. Accuracy and specificity were significantly higher for SmartUrgence and BoneView than Rayvolve for the whole population (P < .0001) and for subgroups. The three algorithms did not differ in sensitivity (P = .27). For SmartUrgence, subgroups did not significantly differ in accuracy, specificity, or sensitivity. For Rayvolve, accuracy and specificity were significantly higher with age 27-36 than ≥53 years (P = .0029 and P = .0019). Specificity was higher for the subgroup knee than foot (P = .0149). For BoneView, accuracy was significantly higher for the subgroups knee than foot (P = .0006) and knee than wrist/hand (P = .0228). Specificity was significantly higher for the subgroups knee than foot (P = .0003) and ankle than foot (P = .0195).
CONCLUSION:The performance of AI detection of acute peripheral fractures in daily radiological practice in an emergency department was good to high and was related to the AI algorithm, patient age, and body region examined.
更多查看译文
AI 理解论文
溯源树
样例
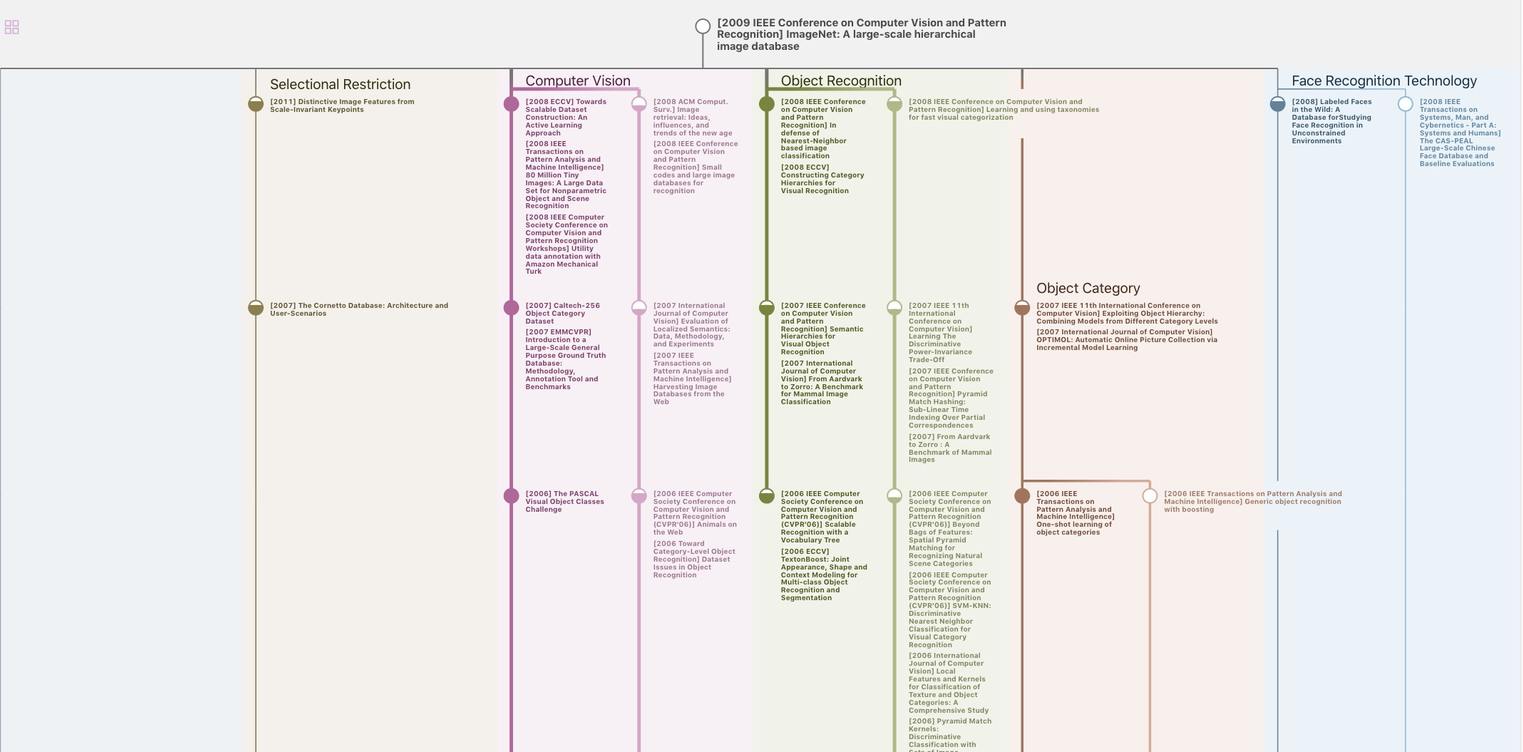
生成溯源树,研究论文发展脉络
Chat Paper
正在生成论文摘要