Kick Back & Relax: Learning to Reconstruct the World by Watching SlowTV
CoRR(2023)
Abstract
Self-supervised monocular depth estimation (SS-MDE) has the potential to scale to vast quantities of data. Unfortunately, existing approaches limit themselves to the automotive domain, resulting in models incapable of generalizing to complex environments such as natural or indoor settings. To address this, we propose a large-scale SlowTV dataset curated from YouTube, containing an order of magnitude more data than existing automotive datasets. SlowTV contains 1.7M images from a rich diversity of environments, such as worldwide seasonal hiking, scenic driving and scuba diving. Using this dataset, we train an SS-MDE model that provides zero-shot generalization to a large collection of indoor/outdoor datasets. The resulting model outperforms all existing SSL approaches and closes the gap on supervised SoTA, despite using a more efficient architecture. We additionally introduce a collection of best-practices to further maximize performance and zero-shot generalization. This includes 1) aspect ratio augmentation, 2) camera intrinsic estimation, 3) support frame randomization and 4) flexible motion estimation. Code is available at https://github.com/jspenmar/slowtv_monodepth.
MoreTranslated text
Key words
slowtv,learning,world
AI Read Science
Must-Reading Tree
Example
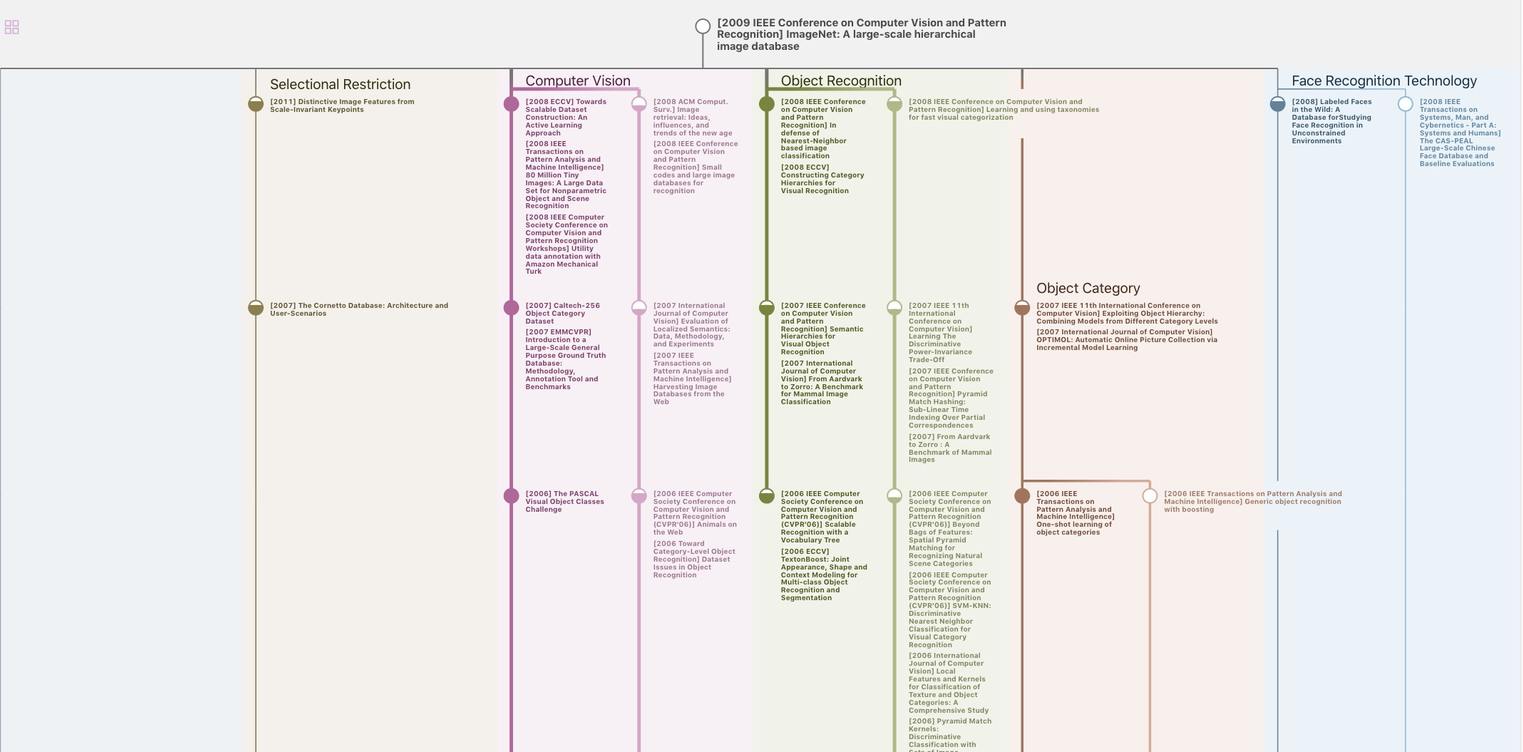
Generate MRT to find the research sequence of this paper
Chat Paper
Summary is being generated by the instructions you defined