Differentially Flat Learning-Based Model Predictive Control Using a Stability, State, and Input Constraining Safety Filter
IEEE Control. Syst. Lett.(2023)
摘要
Learning-based optimal control algorithms control unknown systems using past trajectory data and a learned model of the system dynamics. These controllers use either a linear approximation of the learned dynamics, trading performance for faster computation, or nonlinear optimization methods, which typically perform better but can limit real-time applicability. In this letter, we present a novel nonlinear controller that exploits differential flatness to achieve similar performance to state-of-the-art learning-based controllers but with significantly less computational effort. Differential flatness is a property of dynamical systems whereby nonlinear systems can be exactly linearized through a nonlinear input mapping. Here, the nonlinear transformation is learned as a Gaussian process and is used in a safety filter that guarantees, with high probability, stability as well as input and flat state constraint satisfaction. This safety filter is then used to refine inputs from a flat model predictive controller to perform constrained nonlinear learning-based optimal control through two successive convex optimizations. We compare our method to state-of-the-art learning-based control strategies and achieve similar performance, but with significantly better computational efficiency, while also respecting flat state and input constraints, and guaranteeing stability.
更多查看译文
关键词
Machine learning,predictive control for nonlinear systems,robotics
AI 理解论文
溯源树
样例
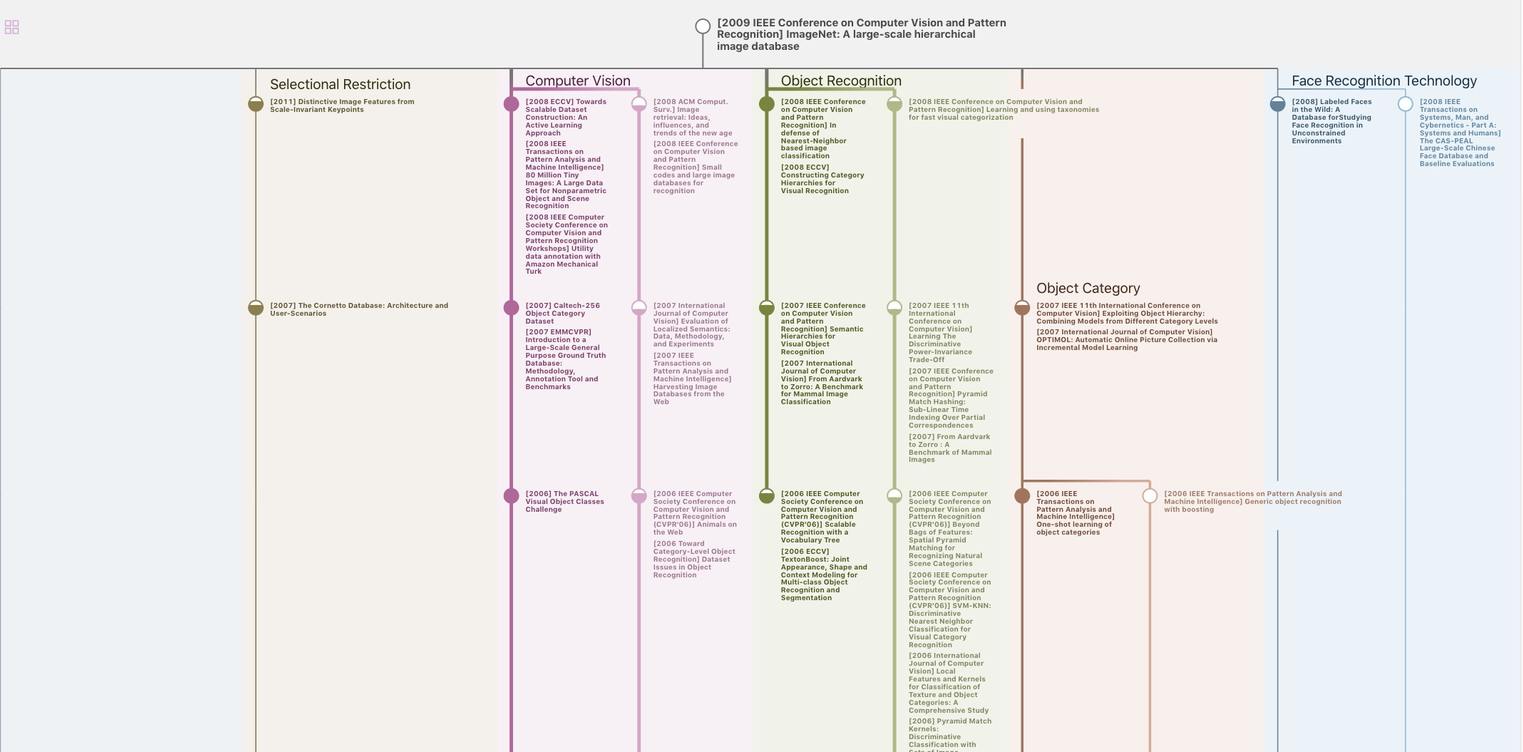
生成溯源树,研究论文发展脉络
Chat Paper
正在生成论文摘要