A Lightweight Approach for Network Intrusion Detection based on Self-Knowledge Distillation
ICC 2023 - IEEE INTERNATIONAL CONFERENCE ON COMMUNICATIONS(2023)
摘要
Network Intrusion Detection (NID) works as a kernel technology for the security network environment, obtaining extensive research and application. Despite enormous efforts by researchers, NID still faces challenges in deploying on resourceconstrained devices. To improve detection accuracy while reducing computational costs and model storage simultaneously, we propose a lightweight intrusion detection approach based on self-knowledge distillation, namely LNet-SKD, which achieves the trade-off between accuracy and efficiency. Specifically, we carefully design the DeepMax block to extract compact representation efficiently and construct the LNet by stacking DeepMax blocks. Furthermore, considering compensating for performance degradation caused by the lightweight network, we adopt batchwise self-knowledge distillation to provide the regularization of training consistency. Experiments on benchmark datasets demonstrate the effectiveness of our proposed LNet-SKD, which outperforms existing state-of-the-art techniques with fewer parameters and lower computation loads.
更多查看译文
关键词
Intrusion detection,deep learning,lightweight network,self-knowledge distillation
AI 理解论文
溯源树
样例
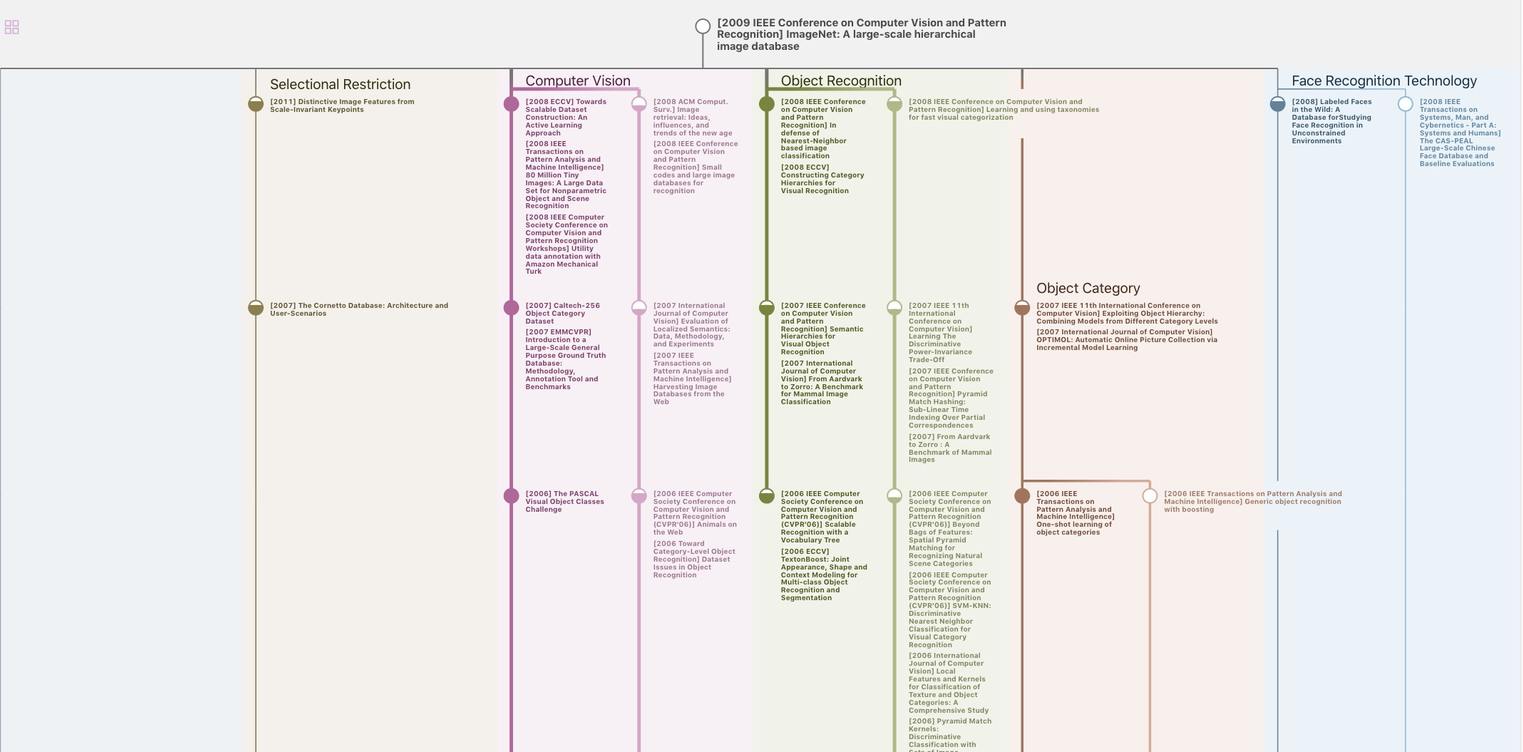
生成溯源树,研究论文发展脉络
Chat Paper
正在生成论文摘要