A non-monotone trust-region method with noisy oracles and additional sampling
arXiv (Cornell University)(2023)
摘要
In this work, we introduce a novel stochastic second-order method, within the
framework of a non-monotone trust-region approach, for solving the
unconstrained, nonlinear, and non-convex optimization problems arising in the
training of deep neural networks. The proposed algorithm makes use of
subsampling strategies which yield noisy approximations of the finite sum
objective function and its gradient. To effectively control the resulting
approximation error, we introduce an adaptive sample size strategy based on
inexpensive additional sampling. Depending on the estimated progress of the
algorithm, this can yield sample size scenarios ranging from mini-batch to full
sample functions. We provide convergence analysis for all possible scenarios
and show that the proposed method achieves almost sure convergence under
standard assumptions for the trust-region framework. We report numerical
experiments showing that the proposed algorithm outperforms its
state-of-the-art counterpart in deep neural network training for image
classification and regression tasks while requiring a significantly smaller
number of gradient evaluations.
更多查看译文
关键词
non-monotone,extra-gradient,trust-region
AI 理解论文
溯源树
样例
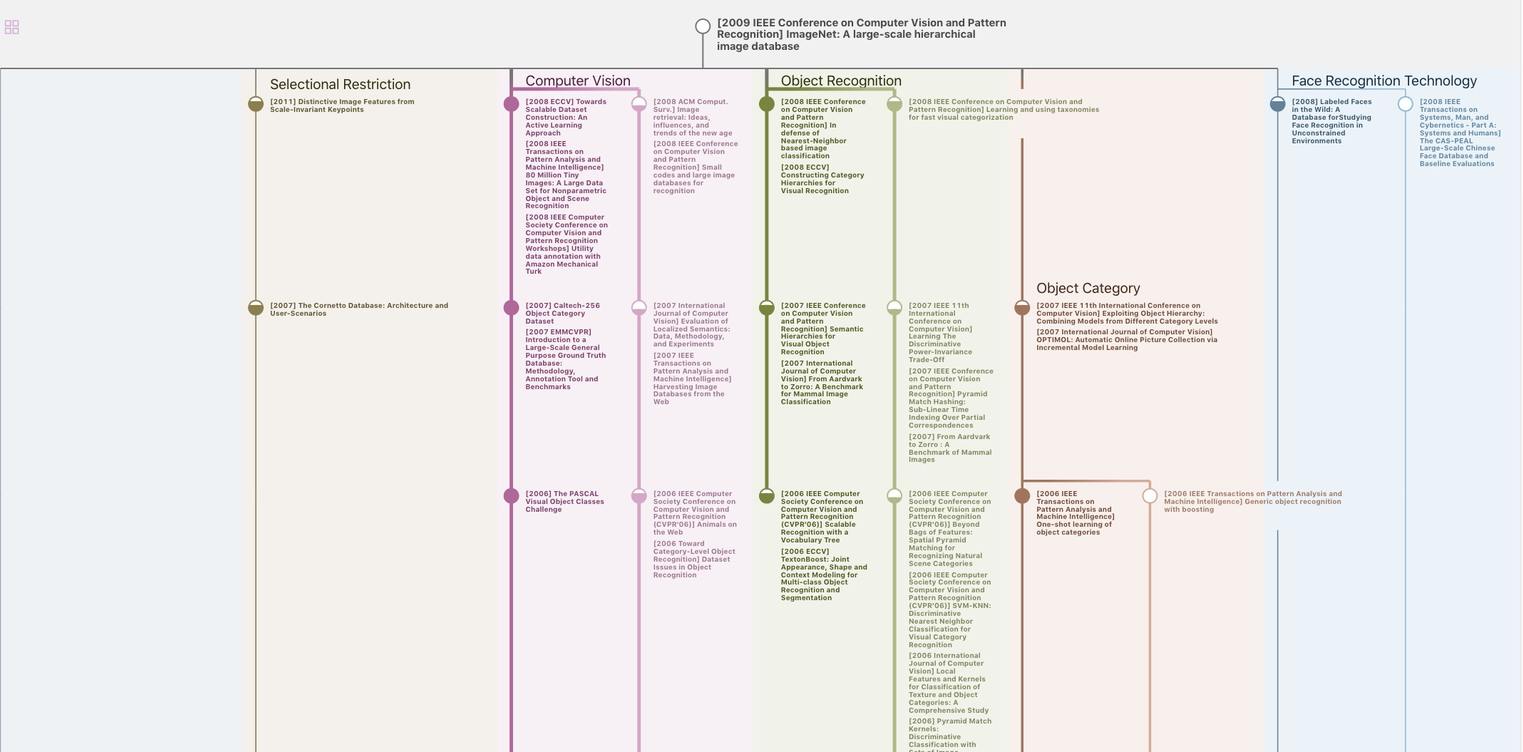
生成溯源树,研究论文发展脉络
Chat Paper
正在生成论文摘要