TUNeS: A Temporal U-Net with Self-Attention for Video-based Surgical Phase Recognition
CoRR(2023)
摘要
To enable context-aware computer assistance in the operating room of the
future, cognitive systems need to understand automatically which surgical phase
is being performed by the medical team. The primary source of information for
surgical phase recognition is typically video, which presents two challenges:
extracting meaningful features from the video stream and effectively modeling
temporal information in the sequence of visual features. For temporal modeling,
attention mechanisms have gained popularity due to their ability to capture
long-range dependencies. In this paper, we explore design choices for attention
in existing temporal models for surgical phase recognition and propose a novel
approach that uses attention more effectively: TUNeS, an efficient and simple
temporal model that incorporates self-attention at the core of a convolutional
U-Net structure. In addition, we propose to train the feature extractor, a
standard CNN, together with an LSTM on preferably long video segments, i.e.,
with long temporal context. In our experiments, all temporal models performed
better on top of feature extractors that were trained with longer temporal
context. On these contextualized features, TUNeS achieves state-of-the-art
results on the Cholec80 dataset.
更多查看译文
关键词
recognition,u-net,self-attention,video-based
AI 理解论文
溯源树
样例
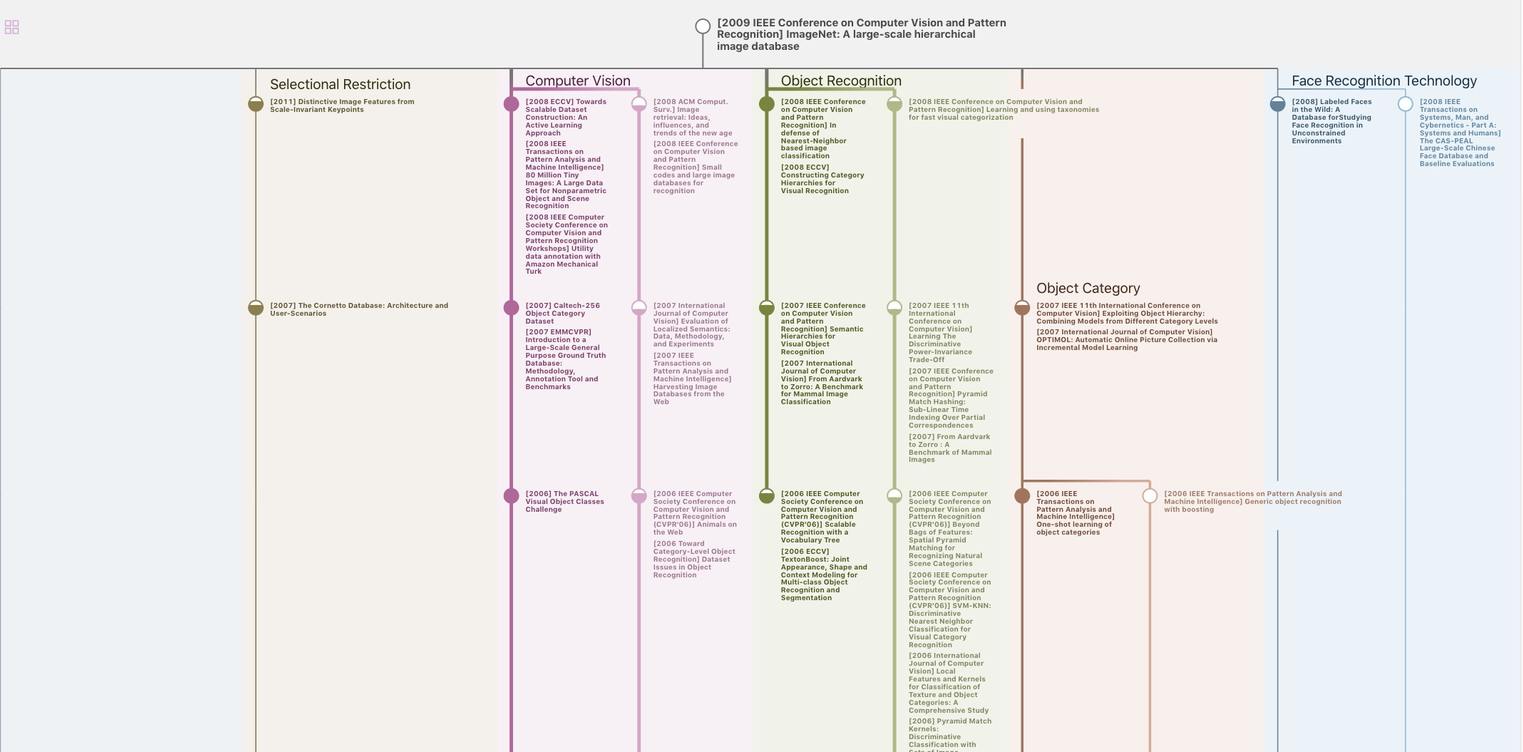
生成溯源树,研究论文发展脉络
Chat Paper
正在生成论文摘要