Detection of outlying patterns from sparse and irregularly sampled electronic health records data
ENGINEERING APPLICATIONS OF ARTIFICIAL INTELLIGENCE(2023)
摘要
Within the intensive care unit (ICU), vital signs such as arterial blood pressure (ABP) collected from electronic health records (EHRs) are typically recorded at different and uneven sampling frequencies and are often infrequently measured due to the nature of the medical treatment. Furthermore, from a temporal trajectory perspective, EHR data are likely to be corrupted by outlying patterns that deviate from normal samples in terms of the curves' magnitude and shape. In this work, we propose a two-stage outlier detection approach for sparse and irregularly sampled (SiS) temporal data using functional data analysis (FDA) tools. In the first stage, an outlier identification measure is defined by a max-min statistic and a clean subset that contains nonoutliers. In the second stage, a multiple hypothesis testing problem is formulated based on the asymptotic distribution of the proposed measure. The simulation-based framework shows that the proposed method is robust to different types of shape and magnitude outliers. The detection results are more accurate than the widely used functional depth methods, especially in extremely sparse settings where the proportion of the observed data points over the entire time series is approximately 10%. Extensive experiments are also conducted on the real-world MIMIC-II dataset, which demonstrate that the method effectively detects clinically meaningful outlying patterns.
更多查看译文
关键词
Clinical decision support,Functional data analysis,Outlier detection,Electronic health records (EHRs)
AI 理解论文
溯源树
样例
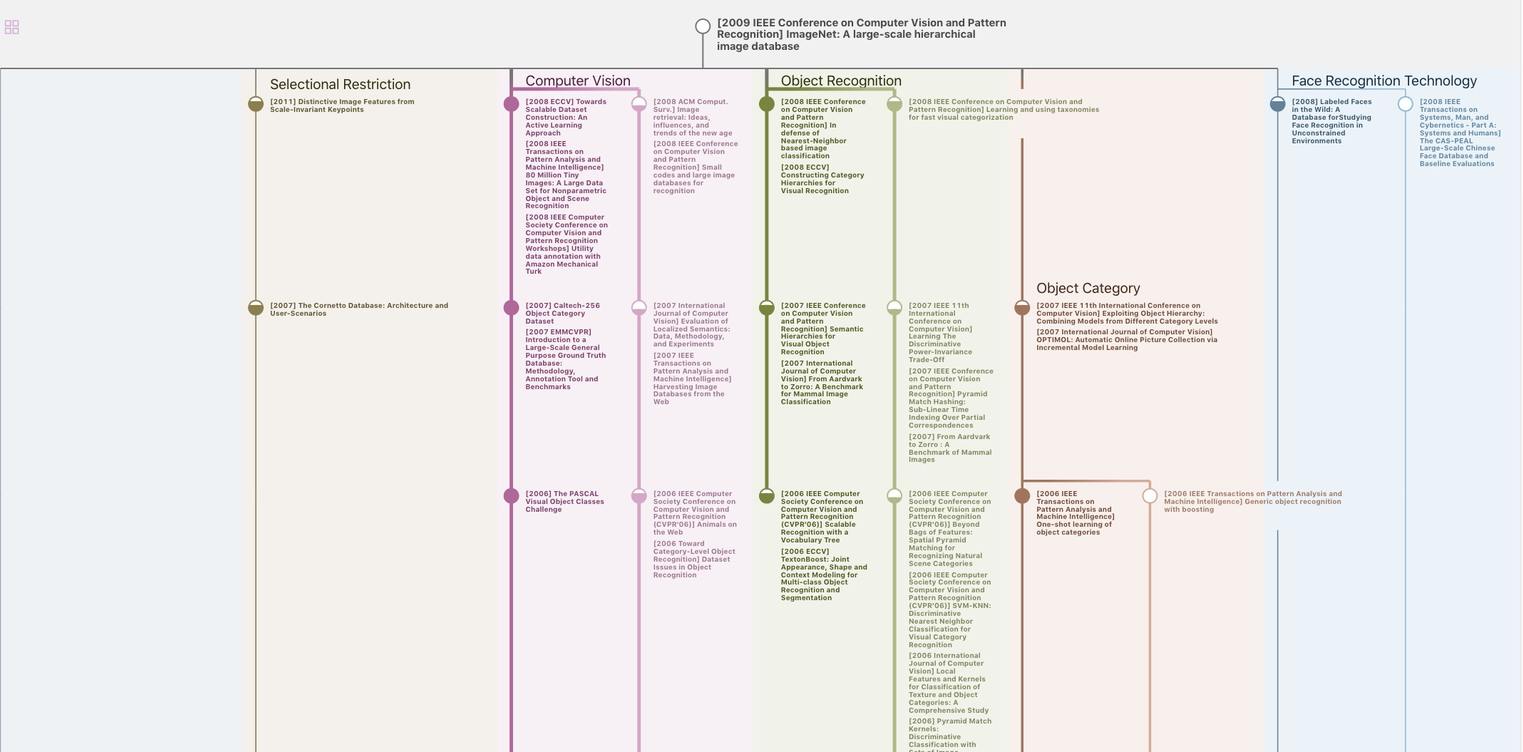
生成溯源树,研究论文发展脉络
Chat Paper
正在生成论文摘要