Self-supervised learning based domain regularization for mask-wearing speaker verification
Speech Communication(2023)
摘要
Automatic speaker verification (ASV) faces an unprecedented problem due to mask-wearing speakers, a consequence of COVID-19. Masked speakers unconsciously alter their normal speaking styles to compensate for the mask transfer effect, changing the statistical distribution of speech and resulting in a domain mismatch that can be dealt with by domain adaptation (DA) algorithms. However, most DA algorithms align speaker embedding distributions to reduce domain shift, which inevitably reduces the discriminative power of speaker representations for the target domain. To improve the adaptation performance, we propose a self-supervised learning-based domain regularization (SDR) algorithm that focuses on the discriminative class structure by removing the domain effect. Different from most DA algorithms, SDR is a co-training structure with two modules, which uses a self-supervised learning strategy to explore domain variables and discriminative speaker representations in the target domain. We collected speech data from 271 mask-wearing speakers, and carried out ASV experiments, whose results showed that the proposed SDR improved performance by 10%-20% based on the equal error rate (EER), compared with state-of-the-art adversarial DA frameworks.
更多查看译文
关键词
Speaker verification,Mask-wearing,Domain adaptation,Self-supervised learning
AI 理解论文
溯源树
样例
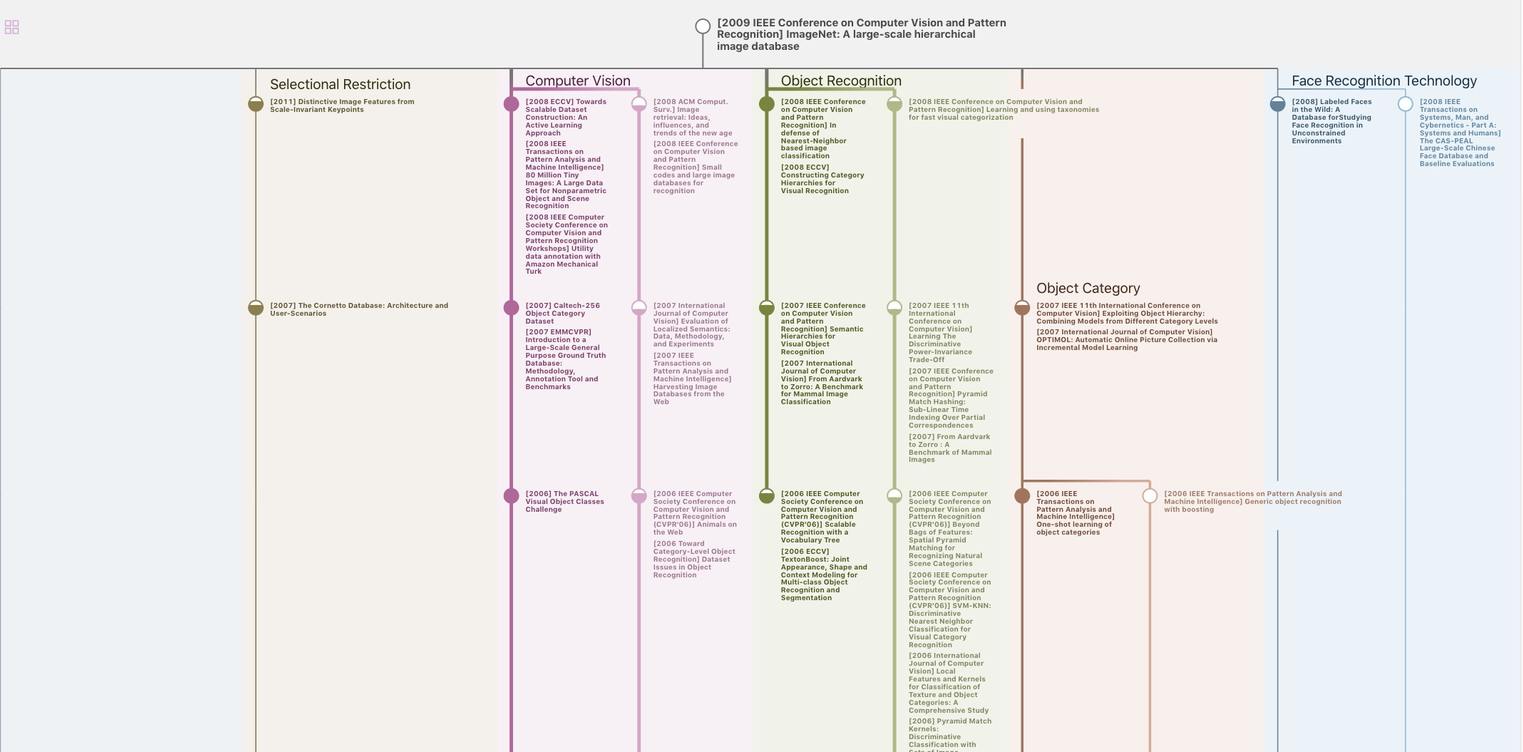
生成溯源树,研究论文发展脉络
Chat Paper
正在生成论文摘要