Path-based multi-hop reasoning over knowledge graph for answering questions via adversarial reinforcement learning
Knowledge-Based Systems(2023)
摘要
Multi-hop knowledge graph question answering targets at pinpointing the answer entities by inferring across multiple triples in knowledge graphs. To enhance model interpretability, path-based methods are proposed. Specifically, with the advances of deep reinforcement learning (DRL), this paper explores to extend the line of RL-based approaches. However, existing solutions suffer from the issue of spurious paths. A major reason lies in that the agent takes an opportunistic way to explicitly pursue the predictive accuracy of answer entities instead of considering correct reasoning paths. To overcome this challenge, our idea is inspired by adversarial learning and we expect that a discriminator could effectively distinguish whether the reasoning chain is correct or not. To this end, we propose an interpretable reasoning method based on adversarial reinforcement learning for multi-hop KGQA, namely Adversarial Reinforcement Reasoning Network (AR2N). AR2N consists of two crucial components: an answer generator (i.e., policy network of RL) and a path discriminator. By alternately updating two components in an adversarial manner, the generator is able to infer answer entities by following the correct reasoning chain, the discriminator is capable of evaluating the plausibility of reasoning paths. Extensive experiments conducted over three benchmark datasets well demonstrate the effectiveness of our method.11For reproducibility, we publicly release the code at https://github.com/iDylanCui/AR2N.
更多查看译文
关键词
knowledge graph,reinforcement learning,path-based,multi-hop
AI 理解论文
溯源树
样例
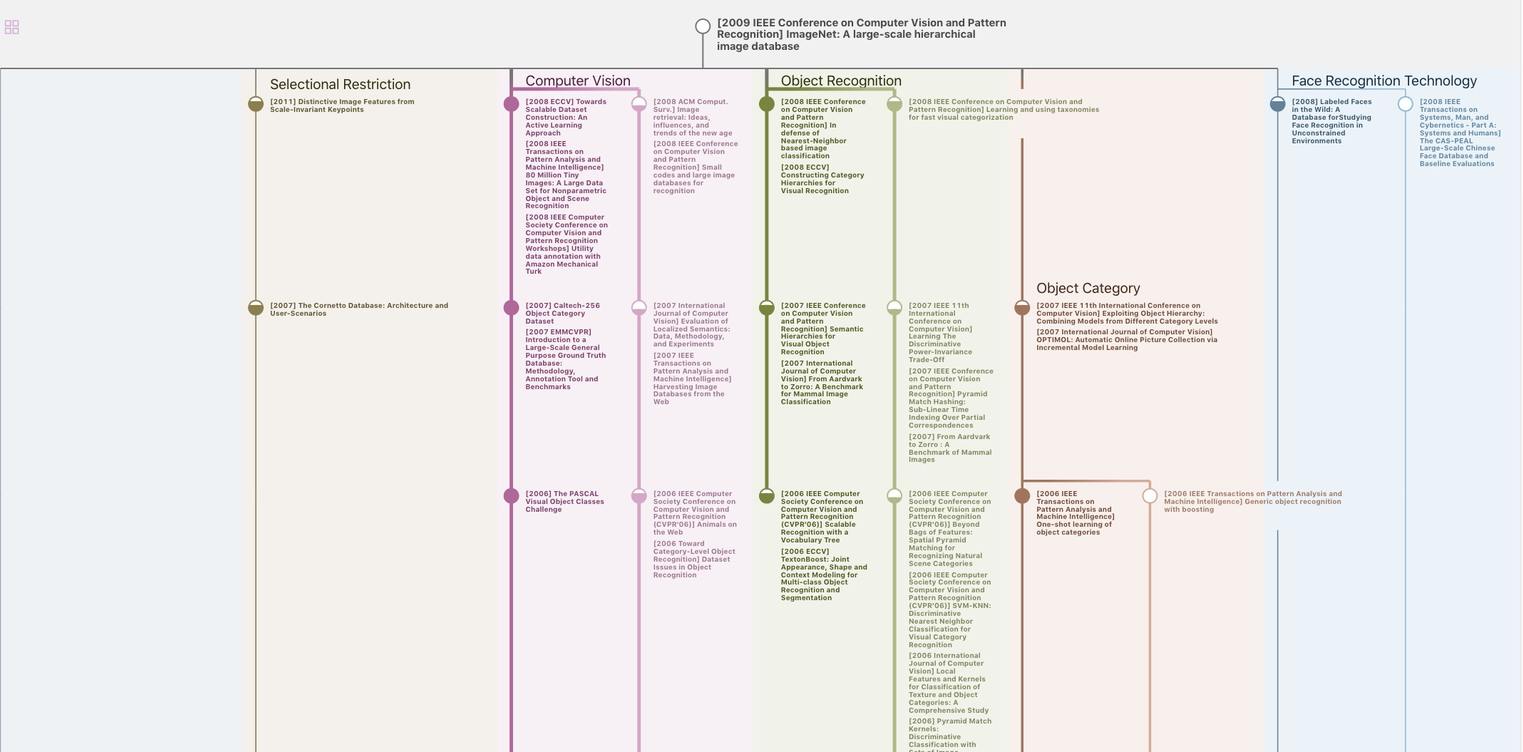
生成溯源树,研究论文发展脉络
Chat Paper
正在生成论文摘要