Multi-scale convolutional attention network for lightweight image super-resolution
Journal of Visual Communication and Image Representation(2023)
摘要
Convolutional neural network (CNN) based methods have recently achieved extraordinary performance in single image super-resolution (SISR) tasks. However, most existing CNN-based approaches increase the model’s depth by stacking massive kernel convolutions, bringing expensive computational costs and limiting their application in mobile devices with limited resources. Furthermore, large kernel convolutions are rarely used in lightweight super-resolution designs. To alleviate the above problems, we propose a multi-scale convolutional attention network (MCAN), a lightweight and efficient network for SISR. Specifically, a multi-scale convolutional attention (MCA) is designed to aggregate the spatial information of different large receptive fields. Since the contextual information of the image has a strong local correlation, we design a local feature enhancement unit (LFEU) to further enhance the local feature extraction. Extensive experimental results illustrate that our proposed MCAN can achieve better performance with lower model complexity compared with other state-of-the-art lightweight methods.
更多查看译文
关键词
Image super-resolution,Convolutional neural network,Lightweight,Attention mechanism
AI 理解论文
溯源树
样例
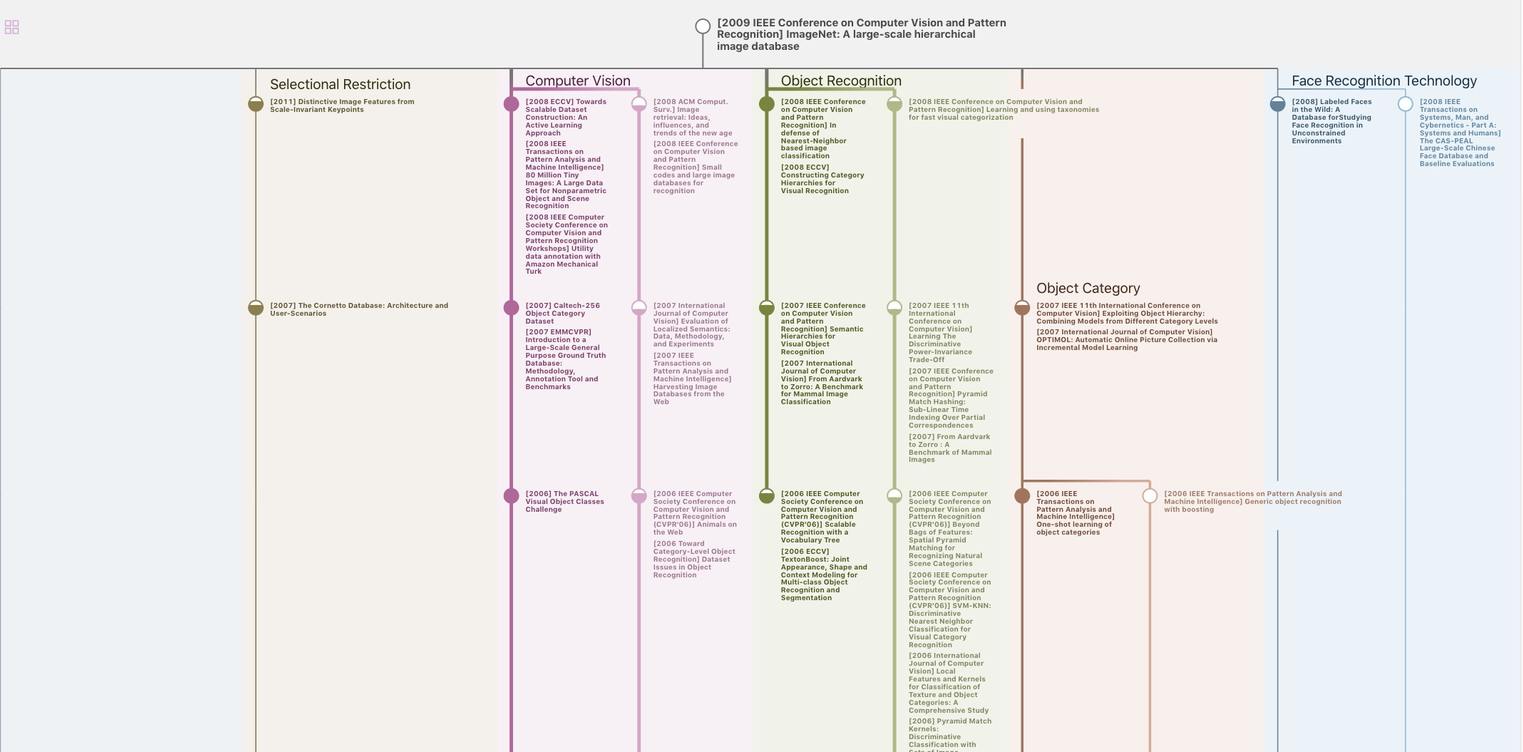
生成溯源树,研究论文发展脉络
Chat Paper
正在生成论文摘要