DyHNet: Learning dynamic heterogeneous network representations
Information Sciences(2023)
摘要
Many real-world networks, such as social networks, contain structural heterogeneity and experience temporal evolution. However, while there has been growing literature on network representation learning, only a few have addressed the need to learn representations for dynamic heterogeneous networks. The objective of our work in this paper is to introduce DyHNet, which learns representations for such networks and distinguishes itself from the state-of-the-art by systematically capturing (1) local node semantics, (2) global network semantics and (3) longer-range temporal associations between network snapshots when learning network representations. Through experiments on four real-world datasets from different domains, namely IMDB with 4,178 movies, AMiner with 10,674 papers, Yelp with 2,693 businesses, and DBLP with 14,376 papers, we demonstrate that our proposed method is able to show consistently better and more robust performance compared to the state-of-the-art techniques on link prediction and node classification tasks. More specifically, we are superior to the best baseline in the temporal link prediction task by approximately 13% and 15% on F1-score for the IMDB and AMiner datasets, respectively. Further, in the node classification task, our findings illustrate that the Micro F1 scores of our proposed model increase by 13% and 17% compared to the runner-up model on the Yelp and DBLP datasets, respectively.1
更多查看译文
关键词
Dynamic heterogeneous network,Network representation learning,Random walk
AI 理解论文
溯源树
样例
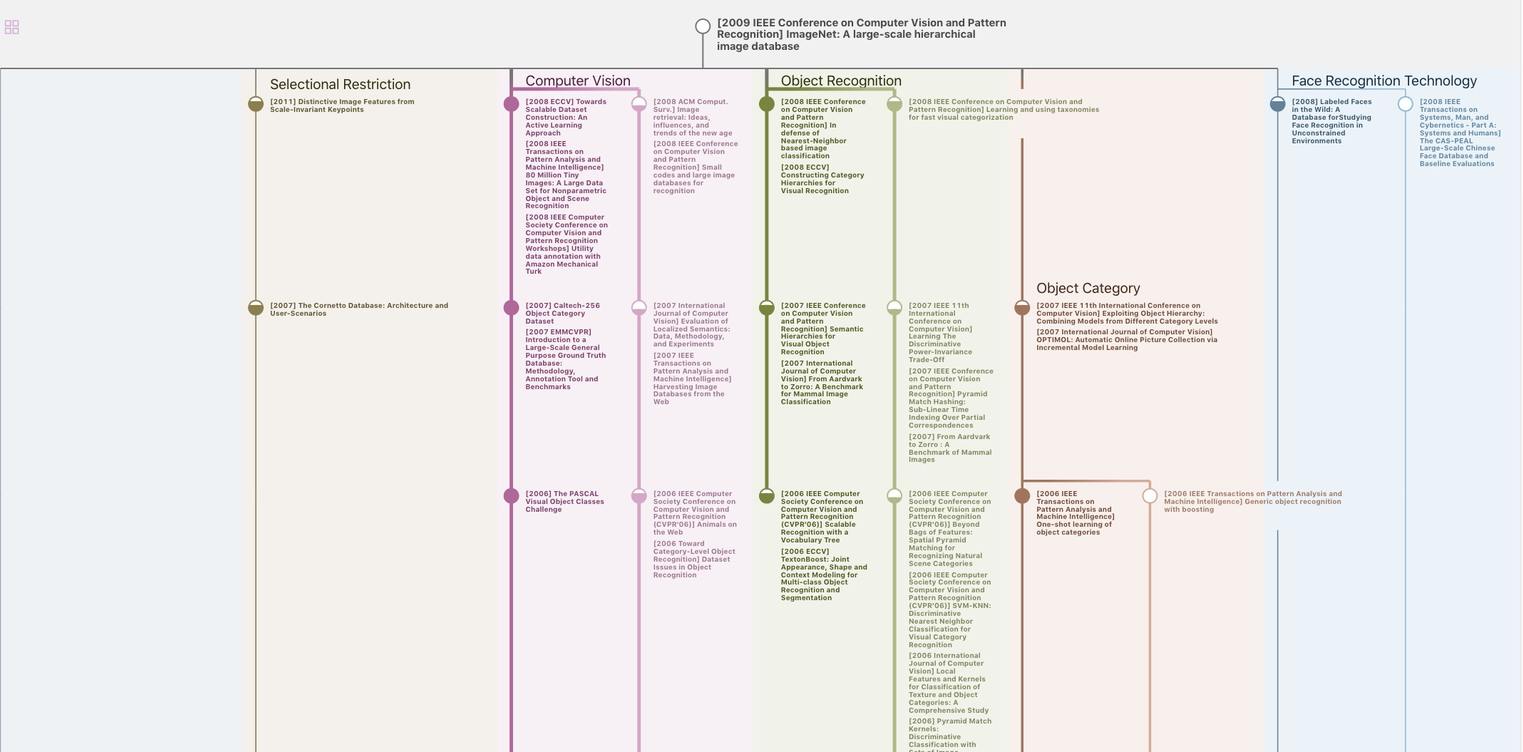
生成溯源树,研究论文发展脉络
Chat Paper
正在生成论文摘要