Component-aware generative autoencoder for structure hybrid and shape completion
Graphical Models(2023)
摘要
Assembling components of man-made objects to create new structures or complete 3D shapes is a popular approach in 3D modeling techniques. Recently, leveraging deep neural networks for assembly-based 3D modeling has been widely studied. However, exploring new component combinations even across different categories is still challenging for most of the deep-learning-based 3D modeling methods. In this paper, we propose a novel generative autoencoder that tackles the component combinations for 3D modeling of man-made objects. We use the segmented input objects to create component volumes that have redundant components and random configurations. By using the input objects and the associated component volumes to train the autoencoder, we can obtain an object volume consisting of components with proper quality and structure as the network output. Such a generative autoencoder can be applied to either multiple object categories for structure hybrid or a single object category for shape completion. We conduct a series of evaluations and experimental results to demonstrate the usability and practicability of our method.
更多查看译文
关键词
Generative autoencoder,Structure hybrid,Shape completion,3D modeling
AI 理解论文
溯源树
样例
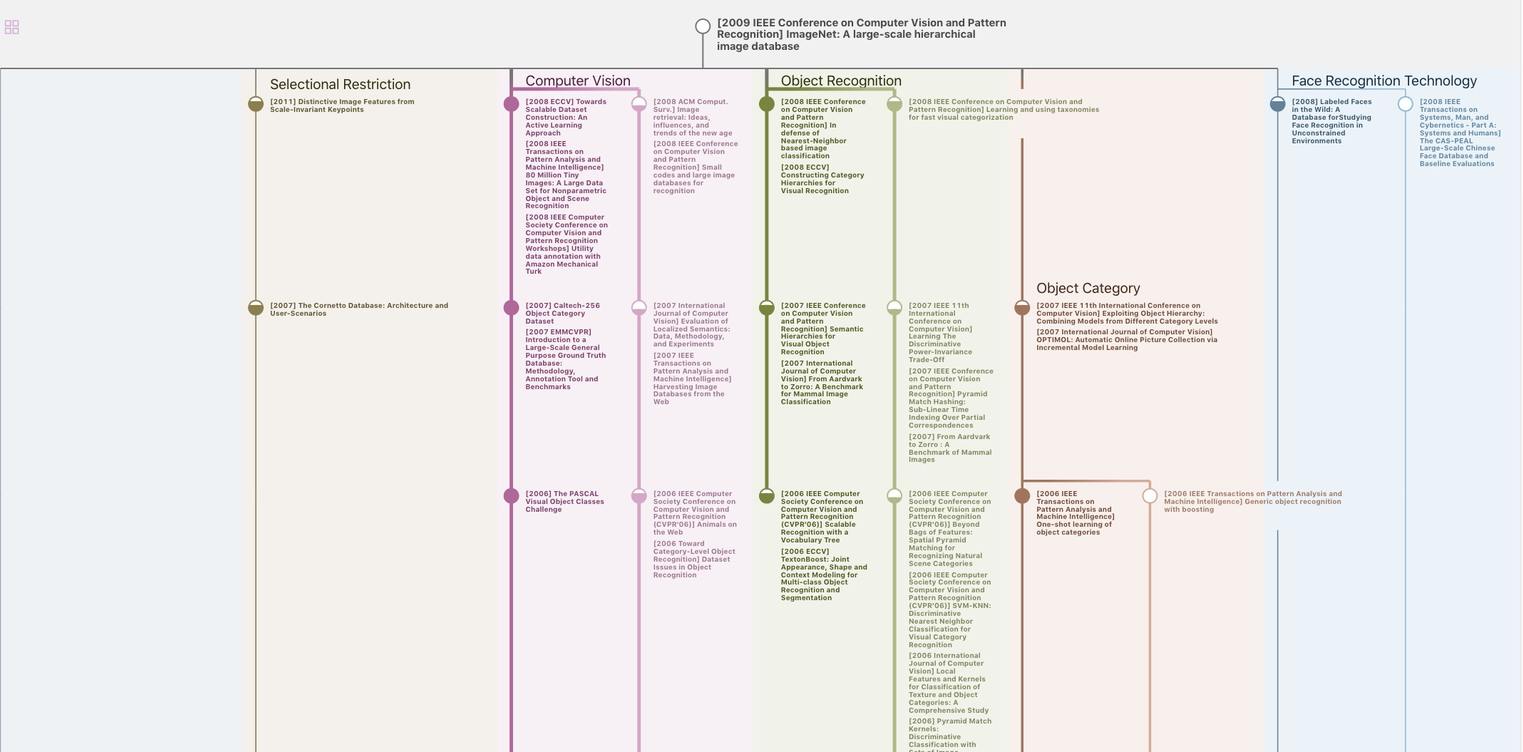
生成溯源树,研究论文发展脉络
Chat Paper
正在生成论文摘要