Segmentation of gastric cancer from microscopic biopsy images using deep learning approach
BIOMEDICAL SIGNAL PROCESSING AND CONTROL(2023)
摘要
In Computer vision for microscopy image analysis applications, object segmentation, classification, and structure localization are essential processes for many biological researchers across the globe. The proposed method is significant as it provides automated cancer diagnosis. The manual interpretation of microscopic biopsy for cancer diagnosis is subjective, time-consuming, and dependent on pathologists' interpretations, degree of skill. An automated cancer diagnosis is required to address these constraints. In this work, A deep learning-based architecture, namely enhanced EMD convolution neural networks (EECNNs) for object segmentation is proposed for microscopy images. The multiple IMFs give detailed frequency components of an image for feature extraction. This improves the performance significantly as compared to the previous works of feature extraction. The presented network is utilized for the segmentation of nuclei and cells in microscopy. The network uses multi-resolution deconvolution filters to train at several levels of an input image, it links the intervening layers for improved localization and context and provides the outcome. The additional convolution layers, which skip the max-pooling function, allow the network to train for varying input intensities and object dimensions while making it adaptable to noisy data. Here, we have compared the experimental results of the proposed method on widely accessible data sets which demonstrate that the proposed method provides superior results in terms of Accuracy of 0.9445, Specificity of 0.9256, Sensitivity of 0.9245, MCC of 0.9654, and BCR of 0.9250.
更多查看译文
关键词
Microscopic biopsy image analysis,Deep learning,Image segmentation,Cancer detection
AI 理解论文
溯源树
样例
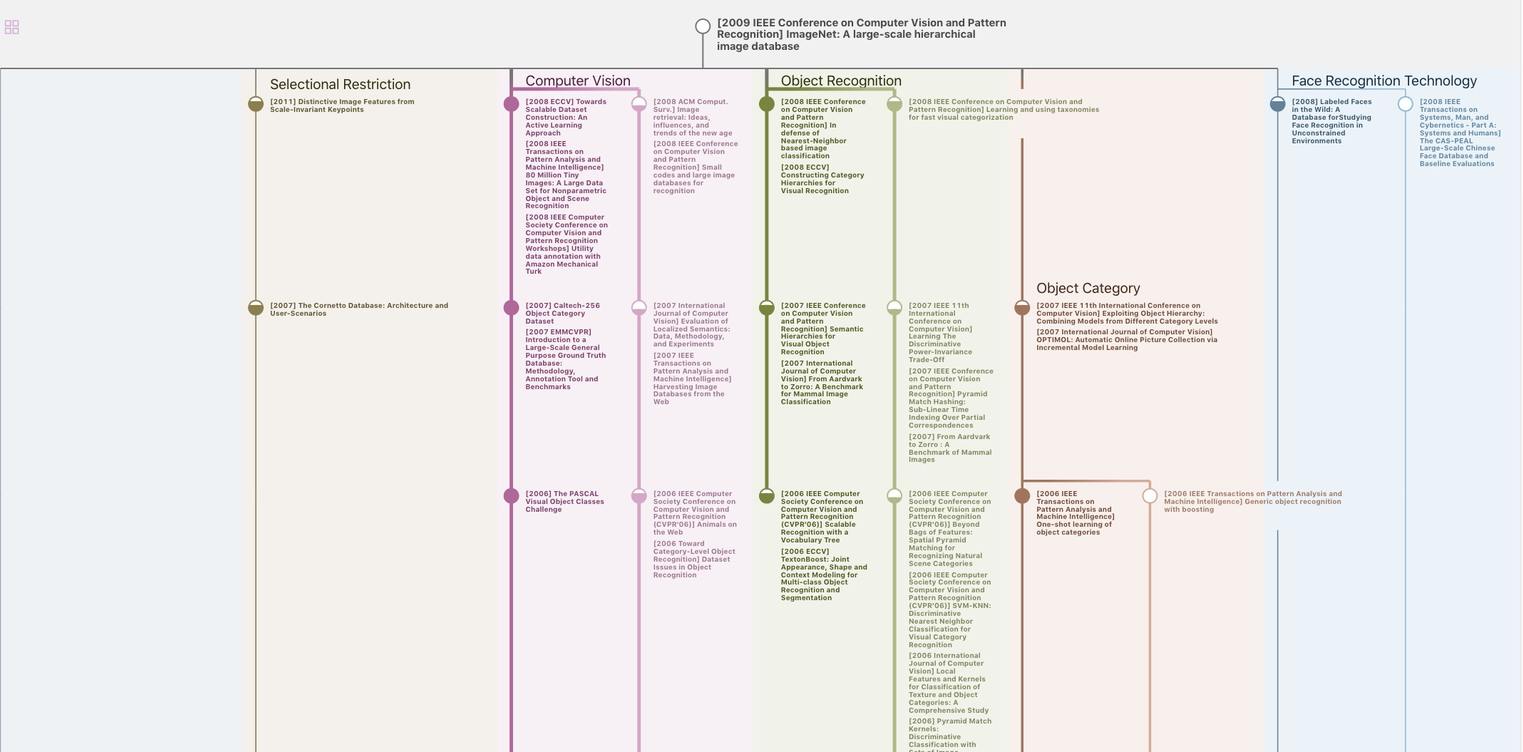
生成溯源树,研究论文发展脉络
Chat Paper
正在生成论文摘要