FSTNet: Learning spatial–temporal correlations from fingerprints for indoor positioning
Ad Hoc Networks(2023)
摘要
Due to the absence of a global positioning system (GPS) signal and pervasive deployment of wireless local area networks (WLANs), Wireless Fidelity (WiFi) fingerprinting has been attracting much attention in indoor positioning services. However, accurate indoor positioning is challenging due to fingerprint ambiguity and instability problems. Most existing positioning methods do not model the spatial and temporal correlations of fingerprints, and this cannot yield satisfactory localization results. Targeting the shortcomings of existing studies, in this paper, we propose a novel deep learning framework, named FSTNet, to learn the spatial–temporal correlations from fingerprints to improve indoor positioning accuracy. In our framework, we first propose a new concept called path fingerprint to solve the fingerprint ambiguity and instability problem. Then, a convolution network is utilized to efficiently capture the local features in path fingerprints. Next, the fingerprint attention mechanism is designed to efficiently capture the spatial features and obtain stable positioning results. Finally, actual on-site experiments are conducted to verify the effectiveness of FSTNet. It is concluded that the proposed modeling and positioning method can effectively capture the temporal and spatial correlations in the received signal strength (RSS) measurements to improve positioning accuracy. In particular, the proposed model could achieve a performance improvement of 44% in terms of a mean positioning error, and 99.2% of the positioning errors are within 2 m.
更多查看译文
关键词
Indoor positioning, Spatial-temporal correlations, Temporal convolutional network, Path fingerprint
AI 理解论文
溯源树
样例
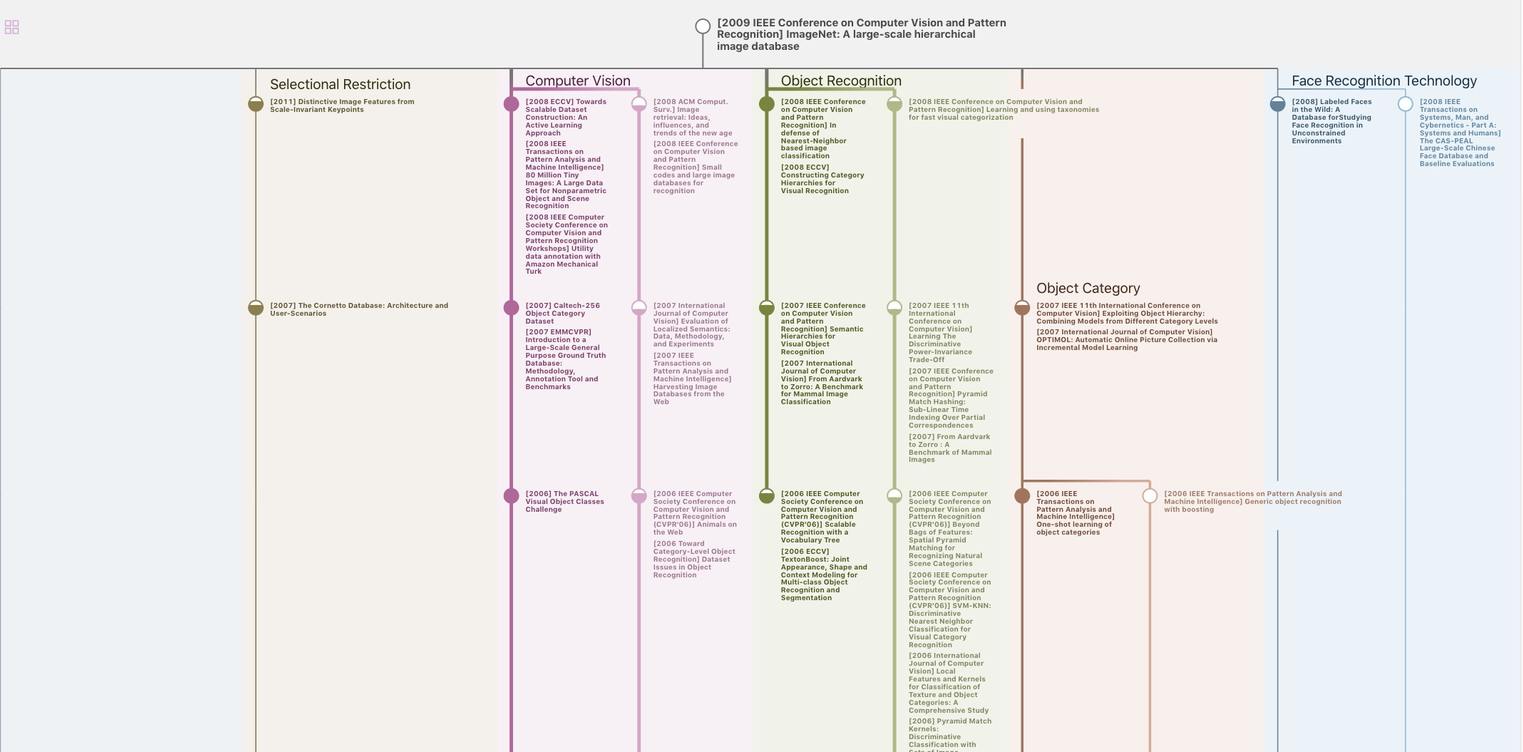
生成溯源树,研究论文发展脉络
Chat Paper
正在生成论文摘要