Multi-task Learning with Hierarchical Guidance for Locating and Stratifying Submucosal Tumors.
IEEE journal of biomedical and health informatics(2023)
摘要
Locating and stratifying the submucosal tumor of the digestive tract from endoscopy ultrasound (EUS) images are of vital significance to the preliminary diagnosis of tumors. However, the above problems are challenging, due to the poor appearance contrast between different layers of the digestive tract wall (DTW) and the narrowness of each layer. Few of existing deep-learning based diagnosis algorithms are devised to tackle this issue. In this article, we build a multi-task framework for simultaneously locating and stratifying the submucosal tumor. And considering the awareness of the DTW is critical to the localization and stratification of the tumor, we integrate the DTW segmentation task into the proposed multi-task framework. Except for sharing a common backbone model, the three tasks are explicitly directed with a hierarchical guidance module, in which the probability map of DTW itself is used to locally enhance the feature representation for tumor localization, and the probability maps of DTW and tumor are jointly employed to locally enhance the feature representation for tumor stratification. Moreover, by means of the dynamic class activation map, probability maps of DTW and tumor are reused to enforce the stratification inference process to pay more attention to DTW and tumor regions, contributing to a reliable and interpretable submucosal tumor stratification model. Additionally, considering the relation with respect to other structures is beneficial for stratifying tumors, we devise a graph reasoning module to replenish non-local relation knowledge for the stratification branch. Experiments on a Stomach-Esophagus and an Intestinal EUS dataset prove that our method achieves very appealing performance on both tumor localization and stratification, significantly outperforming state-of-the-art object detection approaches.
更多查看译文
关键词
submucosal tumors,hierarchical guidance,learning,multi-task
AI 理解论文
溯源树
样例
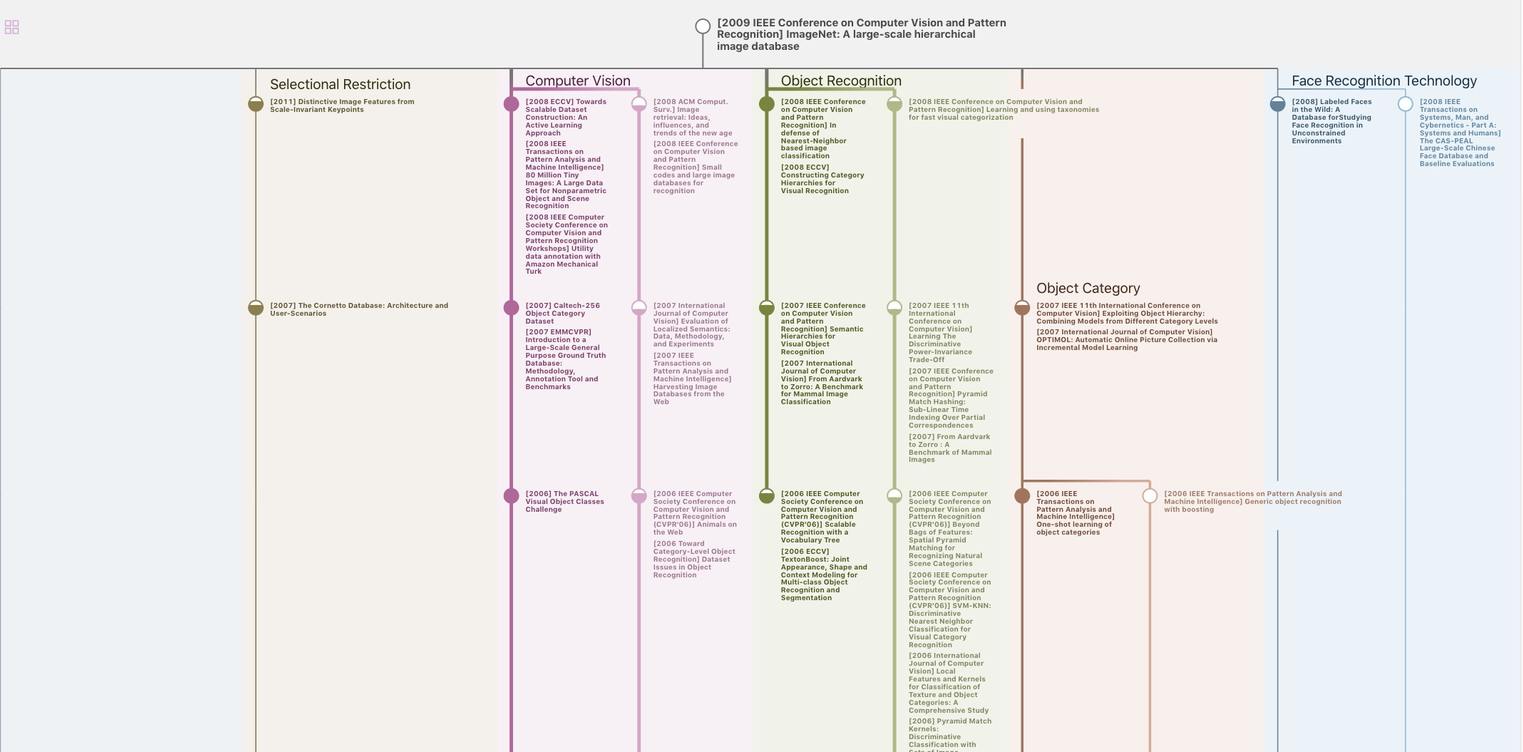
生成溯源树,研究论文发展脉络
Chat Paper
正在生成论文摘要