FlexiAST: Flexibility is What AST Needs
CoRR(2023)
摘要
The objective of this work is to give patch-size flexibility to Audio Spectrogram Transformers (AST). Recent advancements in ASTs have shown superior performance in various audio-based tasks. However, the performance of standard ASTs degrades drastically when evaluated using different patch sizes from that used during training. As a result, AST models are typically re-trained to accommodate changes in patch sizes. To overcome this limitation, this paper proposes a training procedure to provide flexibility to standard AST models without architectural changes, allowing them to work with various patch sizes at the inference stage - FlexiAST. This proposed training approach simply utilizes random patch size selection and resizing of patch and positional embedding weights. Our experiments show that FlexiAST gives similar performance to standard AST models while maintaining its evaluation ability at various patch sizes on different datasets for audio classification tasks.
更多查看译文
关键词
flexibility
AI 理解论文
溯源树
样例
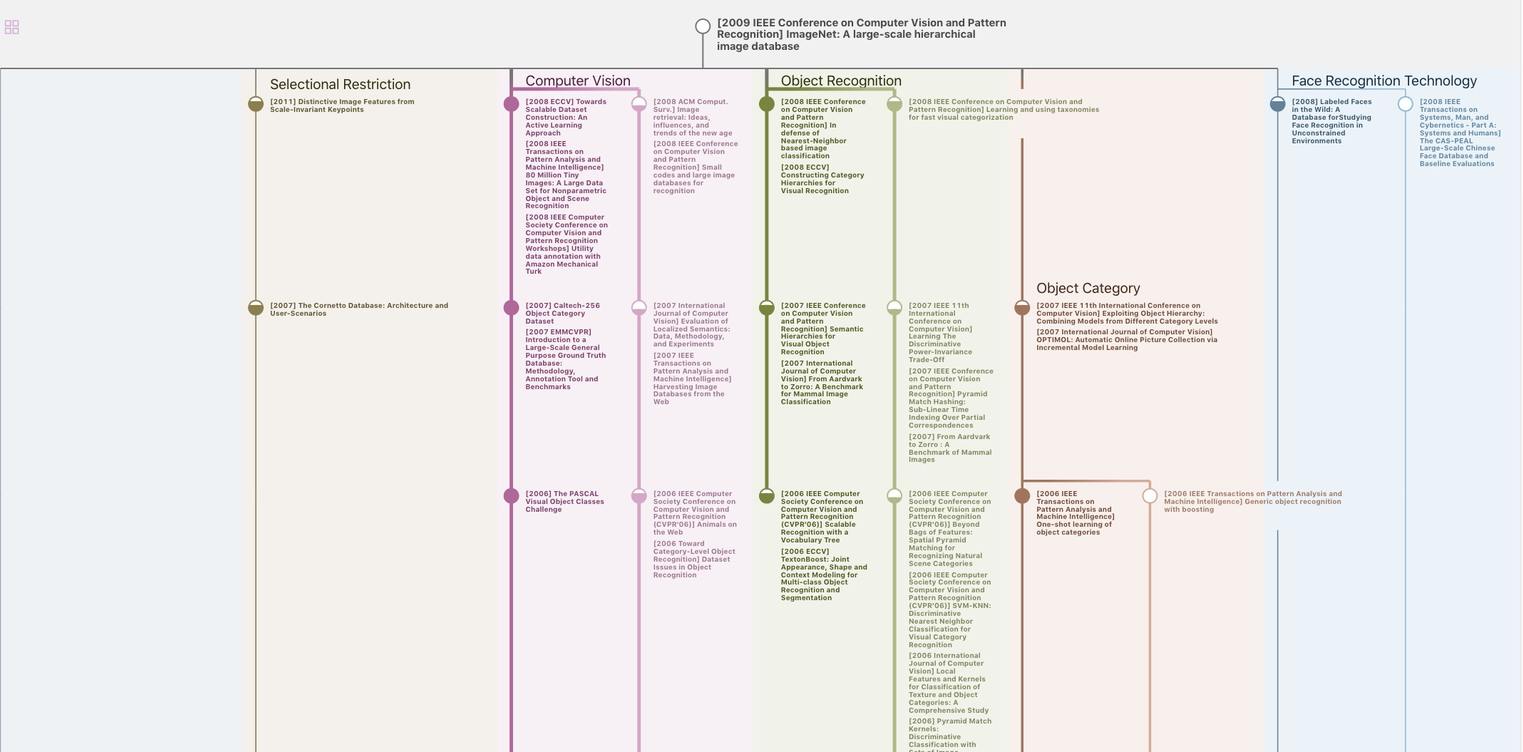
生成溯源树,研究论文发展脉络
Chat Paper
正在生成论文摘要