Modeling Orders of User Behaviors via Differentiable Sorting: A Multi-task Framework to Predicting User Post-click Conversion
PROCEEDINGS OF THE 46TH INTERNATIONAL ACM SIGIR CONFERENCE ON RESEARCH AND DEVELOPMENT IN INFORMATION RETRIEVAL, SIGIR 2023(2023)
Abstract
User post-click conversion prediction is of high interest to researchers and developers. Recent studies employ multi-task learning to tackle the selection bias and data sparsity problem, two severe challenges in post-click behavior prediction, by incorporating click data. However, prior works mainly foucsed on pointwise learning and the orders of labels (i.e., click and post-click) are not well explored, which naturally poses a listwise learning problem. Inspired by recent advances on differentiable sorting, in this paper, we propose a novel multi-task framework that leverages orders of user behaviors to predict user post-click conversion in an end-to-end approach. Specifically, we define an aggregation operator to combine predicted outputs of different tasks to a unified score, then we use the computed scores to model the label relations via differentiable sorting. Extensive experiments on public and industrial datasets show the superiority of our proposed model against competitive baselines.
MoreTranslated text
Key words
Recommendation,multi-task learning,differentiable sorting
AI Read Science
Must-Reading Tree
Example
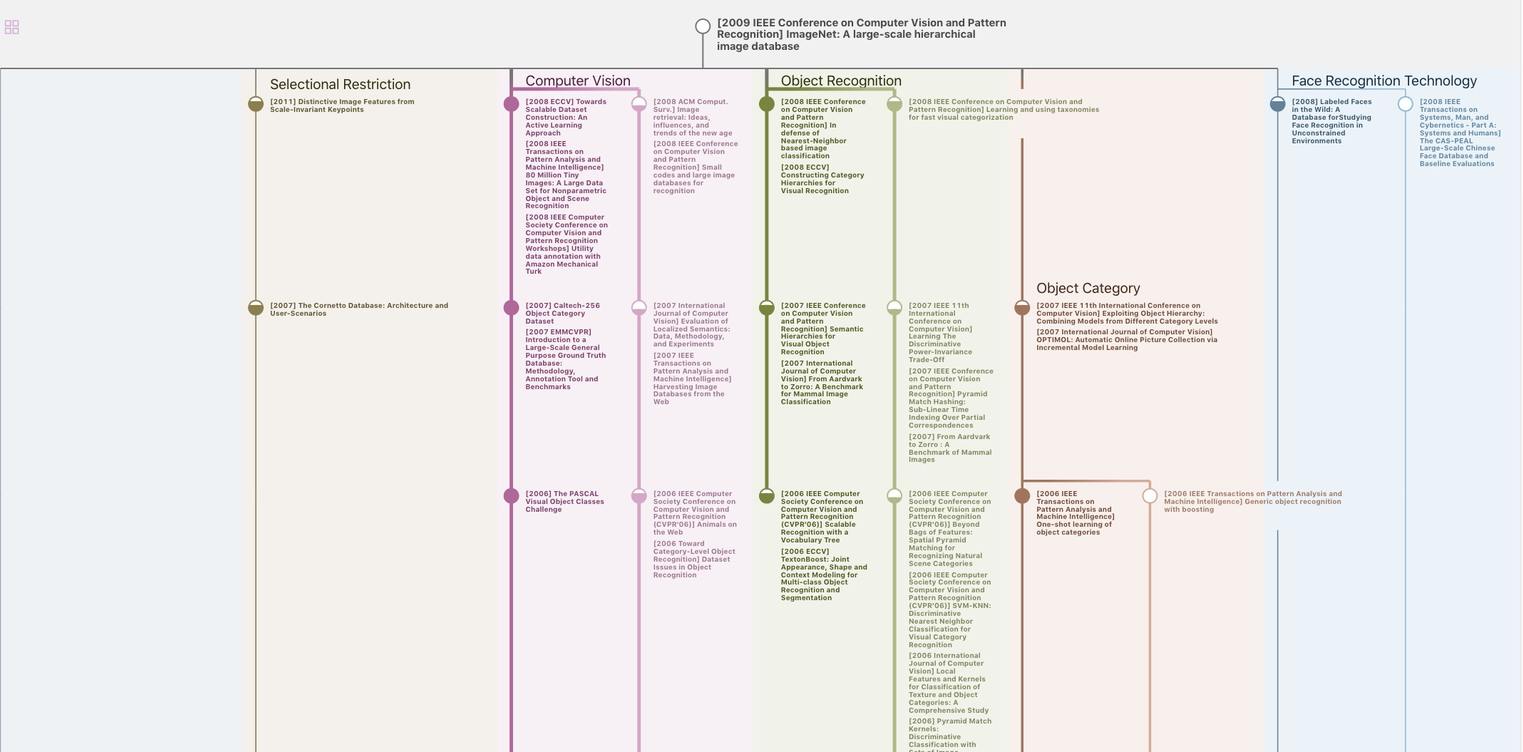
Generate MRT to find the research sequence of this paper
Chat Paper
Summary is being generated by the instructions you defined