Frequency-Mixed Single-Source Domain Generalization for Medical Image Segmentation
MEDICAL IMAGE COMPUTING AND COMPUTER ASSISTED INTERVENTION, MICCAI 2023, PT VI(2023)
摘要
The annotation scarcity of medical image segmentation poses challenges in collecting sufficient training data for deep learning models. Specifically, models trained on limited data may not generalize well to other unseen data domains, resulting in a domain shift issue. Consequently, domain generalization (DG) is developed to boost the performance of segmentation models on unseen domains. However, the DG setup requires multiple source domains, which impedes the efficient deployment of segmentation algorithms in clinical scenarios. To address this challenge and improve the segmentation model's generalizability, we propose a novel approach called the Frequency-mixed Single-source Domain Generalization method (FreeSDG). By analyzing the frequency's effect on domain discrepancy, FreeSDG leverages a mixed frequency spectrum to augment the single-source domain. Additionally, self-supervision is constructed in the domain augmentation to learn robust context-aware representations for the segmentation task. Experimental results on five datasets of three modalities demonstrate the effectiveness of the proposed algorithm. FreeSDG outperforms state-of-the-art methods and significantly improves the segmentation model's generalizability. Therefore, FreeSDG provides a promising solution for enhancing the generalization of medical image segmentation models, especially when annotated data is scarce. The code is available at https://github.com/liamheng/Non-IID_Medical_Image_Segmentation.
更多查看译文
关键词
Medical image segmentation,single-source domain generalization,domain augmentation,frequency spectrum
AI 理解论文
溯源树
样例
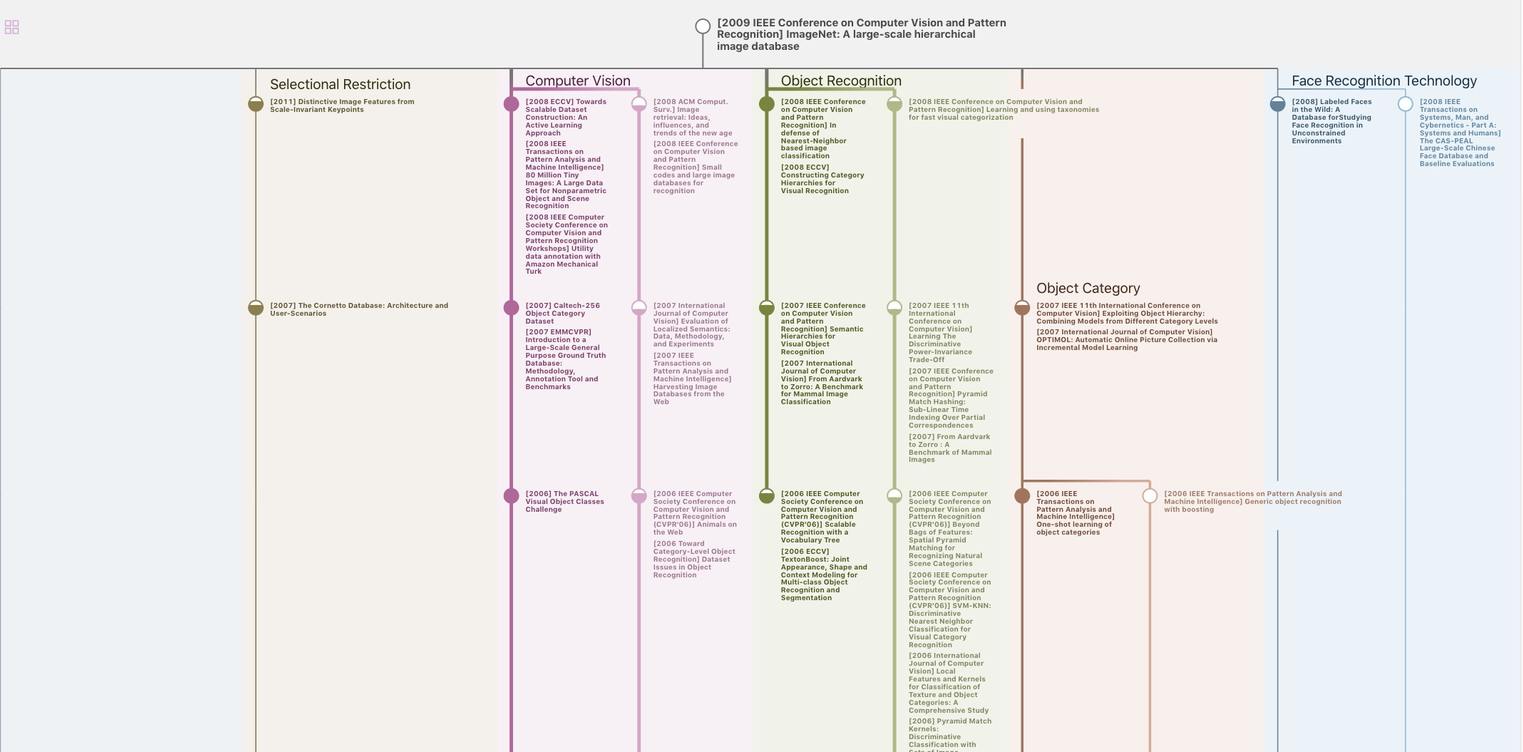
生成溯源树,研究论文发展脉络
Chat Paper
正在生成论文摘要