EVIL: Evidential Inference Learning for Trustworthy Semi-supervised Medical Image Segmentation
CoRR(2023)
摘要
Recently, uncertainty-aware methods have attracted increasing attention in semi-supervised medical image segmentation. However, current methods usually suffer from the drawback that it is difficult to balance the computational cost, estimation accuracy, and theoretical support in a unified framework. To alleviate this problem, we introduce the Dempster-Shafer Theory of Evidence (DST) into semi-supervised medical image segmentation, dubbed Evidential Inference Learning (EVIL). EVIL provides a theoretically guaranteed solution to infer accurate uncertainty quantification in a single forward pass. Trustworthy pseudo labels on unlabeled data are generated after uncertainty estimation. The recently proposed consistency regularization-based training paradigm is adopted in our framework, which enforces the consistency on the perturbed predictions to enhance the generalization with few labeled data. Experimental results show that EVIL achieves competitive performance in comparison with several state-of-the-art methods on the public dataset.
更多查看译文
关键词
evidential inference learning,segmentation,image,semi-supervised
AI 理解论文
溯源树
样例
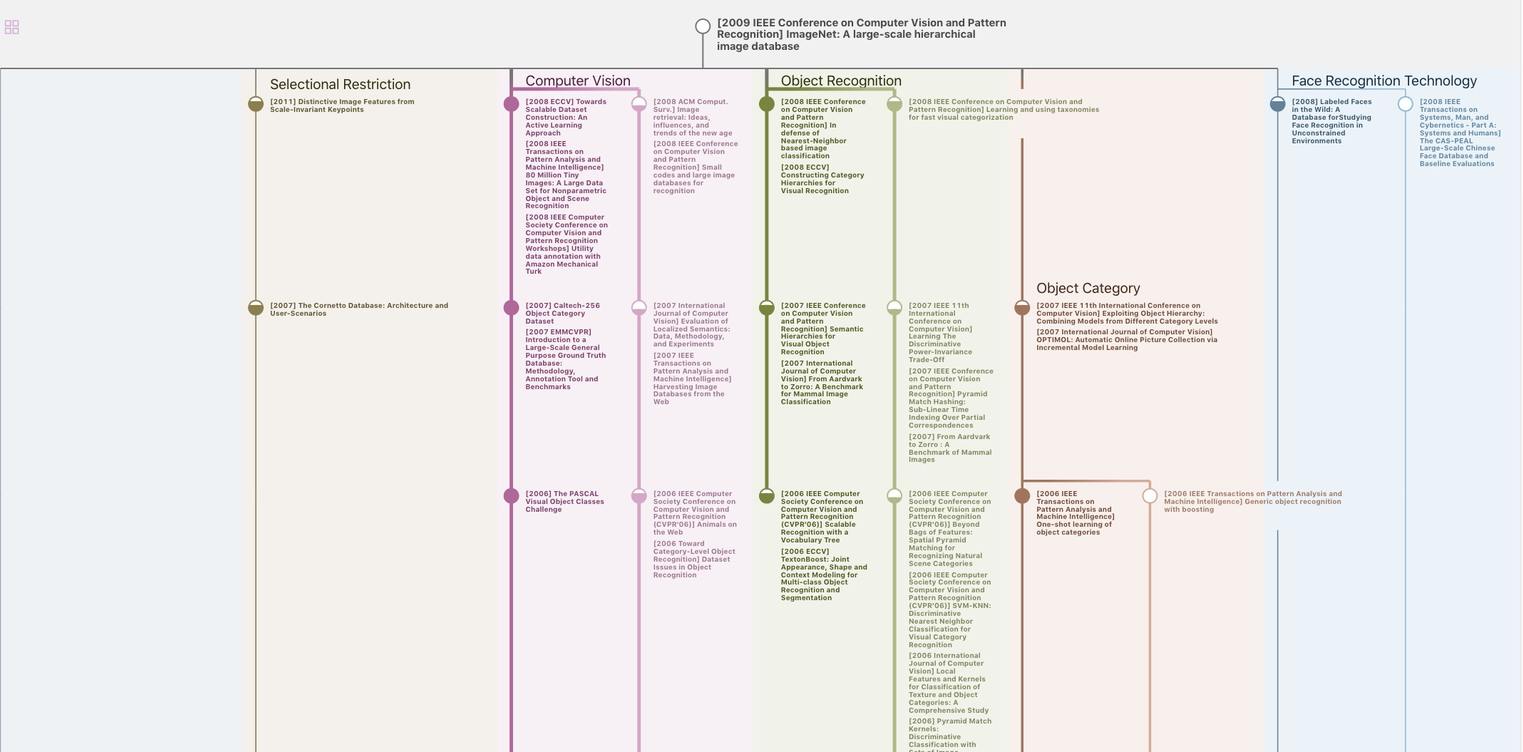
生成溯源树,研究论文发展脉络
Chat Paper
正在生成论文摘要