Neural Network Pruning as Spectrum Preserving Process
CoRR(2023)
摘要
Neural networks have achieved remarkable performance in various application domains. Nevertheless, a large number of weights in pre-trained deep neural networks prohibit them from being deployed on smartphones and embedded systems. It is highly desirable to obtain lightweight versions of neural networks for inference in edge devices. Many cost-effective approaches were proposed to prune dense and convolutional layers that are common in deep neural networks and dominant in the parameter space. However, a unified theoretical foundation for the problem mostly is missing. In this paper, we identify the close connection between matrix spectrum learning and neural network training for dense and convolutional layers and argue that weight pruning is essentially a matrix sparsification process to preserve the spectrum. Based on the analysis, we also propose a matrix sparsification algorithm tailored for neural network pruning that yields better pruning result. We carefully design and conduct experiments to support our arguments. Hence we provide a consolidated viewpoint for neural network pruning and enhance the interpretability of deep neural networks by identifying and preserving the critical neural weights.
更多查看译文
关键词
spectrum preserving process,network
AI 理解论文
溯源树
样例
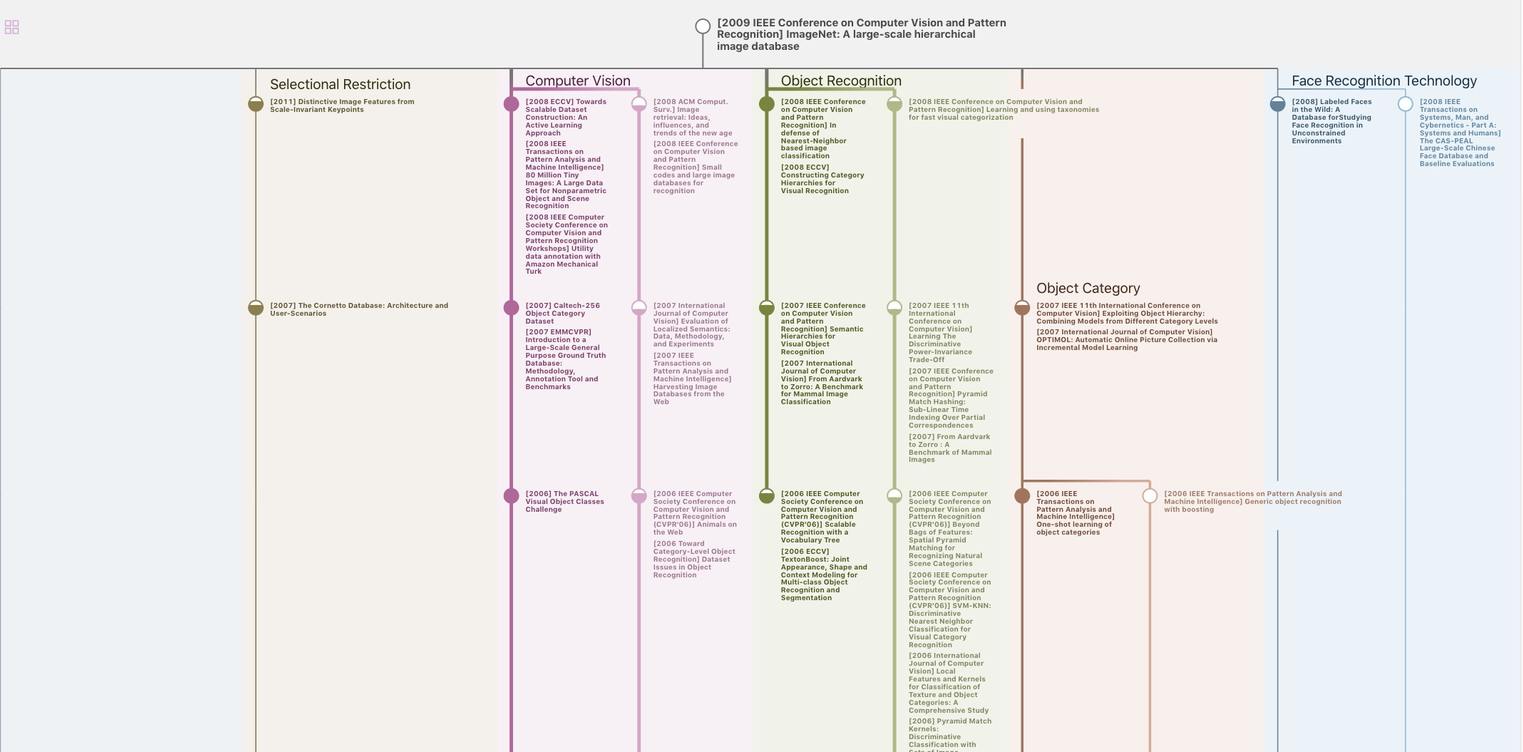
生成溯源树,研究论文发展脉络
Chat Paper
正在生成论文摘要