Meta-Value Learning: a General Framework for Learning with Learning Awareness
CoRR(2023)
摘要
Gradient-based learning in multi-agent systems is difficult because the gradient derives from a first-order model which does not account for the interaction between agents' learning processes. LOLA (arXiv:1709.04326) accounts for this by differentiating through one step of optimization. We extend the ideas of LOLA and develop a fully-general value-based approach to optimization. At the core is a function we call the meta-value, which at each point in joint-policy space gives for each agent a discounted sum of its objective over future optimization steps. We argue that the gradient of the meta-value gives a more reliable improvement direction than the gradient of the original objective, because the meta-value derives from empirical observations of the effects of optimization. We show how the meta-value can be approximated by training a neural network to minimize TD error along optimization trajectories in which agents follow the gradient of the meta-value. We analyze the behavior of our method on the Logistic Game and on the Iterated Prisoner's Dilemma.
更多查看译文
关键词
learning,awareness,meta-value
AI 理解论文
溯源树
样例
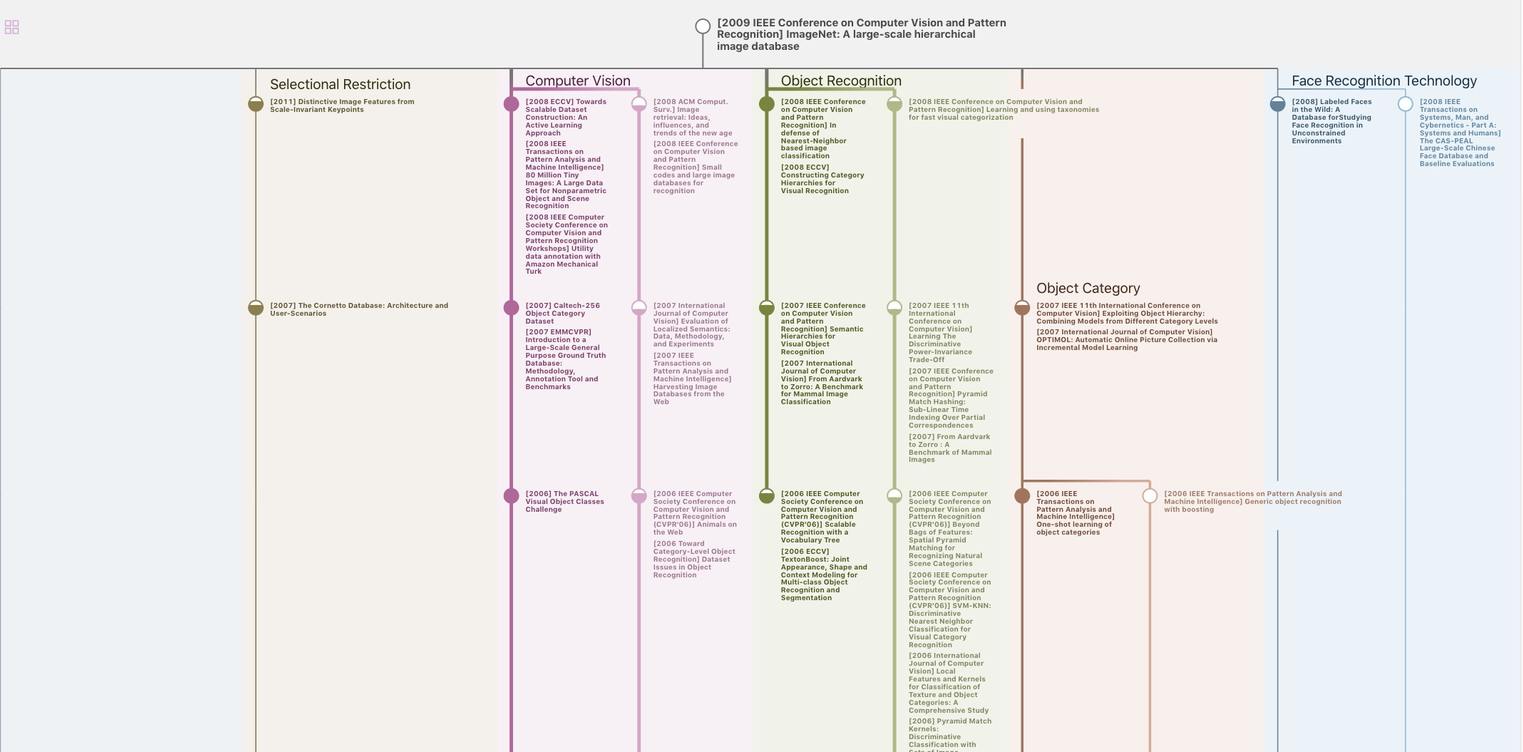
生成溯源树,研究论文发展脉络
Chat Paper
正在生成论文摘要