Federated Learning for Network Intrusion Detection in Ambient Assisted Living Environments
IEEE Internet Computing(2023)
摘要
Given the Internet of Things’ rapid expansion and widespread adoption, it is of great concern to establish secure interaction between devices without worsening the quality of their performance. The use of machine learning techniques has been shown to improve detection of anomalous behavior in these types of networks, but their implementation leads to poor performance and compromised privacy. To better address these shortcomings, federated learning (FL) has been introduced. FL enables devices to collaboratively train and evaluate a shared model while keeping personal data on site (e.g., smart homes, intensive care units, hospitals, and so on), thus minimizing the possibility of an attack and fostering real-time distribution of models and learning. This article investigates the performance of FL in comparison to deep learning (DL) with respect to network intrusion detection in ambient assisted living environments. The results demonstrate comparable performances of FL with DL while achieving improved data privacy and security.
更多查看译文
关键词
federated learning,intrusion detection,network intrusion detection,ambient assisted living environments
AI 理解论文
溯源树
样例
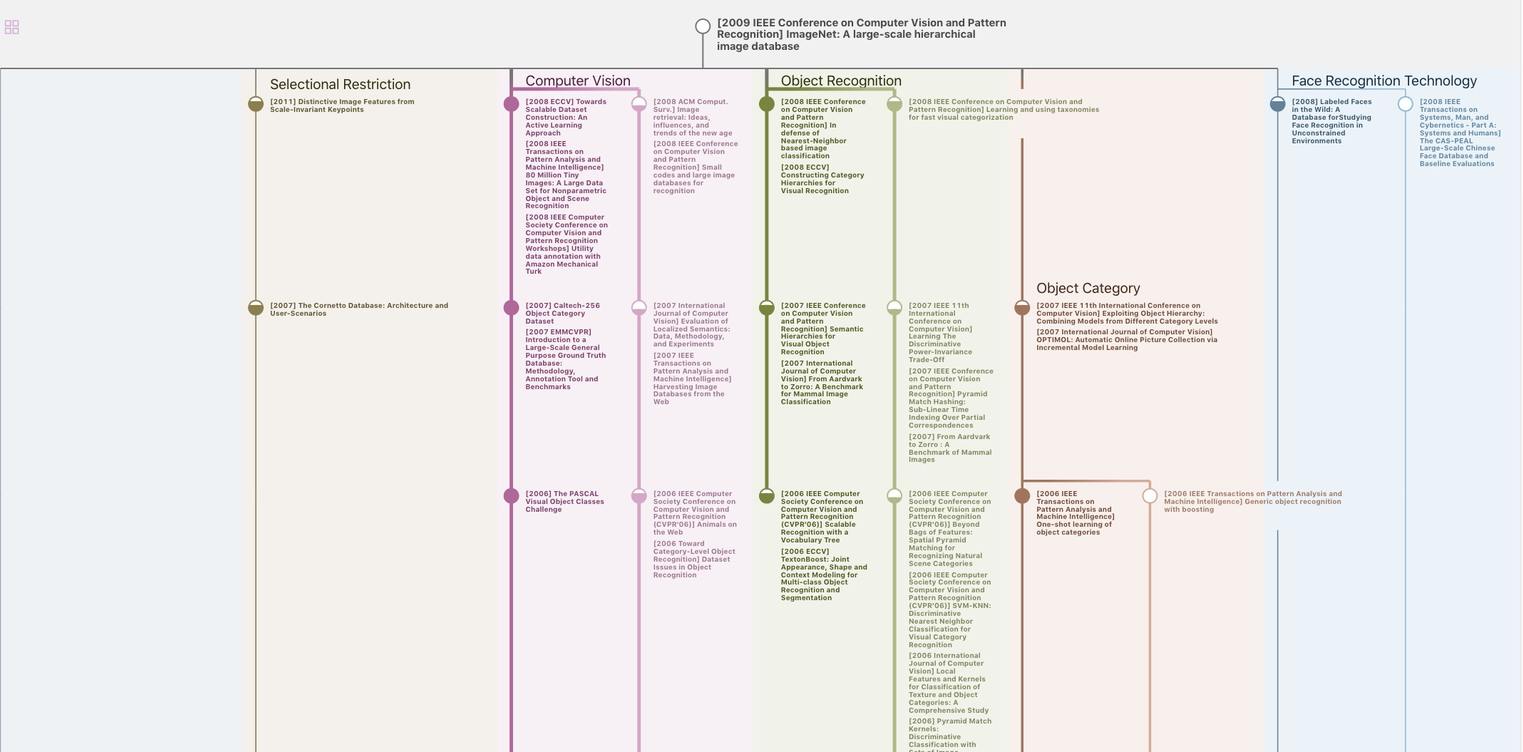
生成溯源树,研究论文发展脉络
Chat Paper
正在生成论文摘要