Multi-view computable online learner modeling by heterogeneous network: An AI-enabled perspective
Information Sciences(2023)
摘要
To support accurate personalized online intelligent education applications in the big data environment, computable online learner modeling is very important. It can accurately capture the unique needs and characteristics of learners in a computable way and provides customized learning experiences. We propose a structured semantic embedding-based approach for online learner modeling, which considers both the multidimensional learners' characteristics and heterogeneous information on the learning context to develop a comprehensive learner model. Specifically, an AI-enabled heterogeneous information network embedding technique is employed to encode complications of different learners' characteristics and rich relationships between them, which integrate different explicit characteristic information and implicit association information about inherent multidimensional interactions for computable learners modeling. Extensive experimental results and evaluations demonstrate that the proposed model achieves the optimal performance on the Precision@N and Recall@N metrics in course recommendation tasks, and the Accuracy and F−score metrics in learner clustering tasks are also better than other baseline models, which prove the ability to boost the performance of downstream tasks by incorporating and capturing the underlying semantic and structural information among different types of nodes to fuse the embedding for learner modeling.
更多查看译文
关键词
Computable modeling, Multi-view, Semantic embeddings, Heterogeneous network
AI 理解论文
溯源树
样例
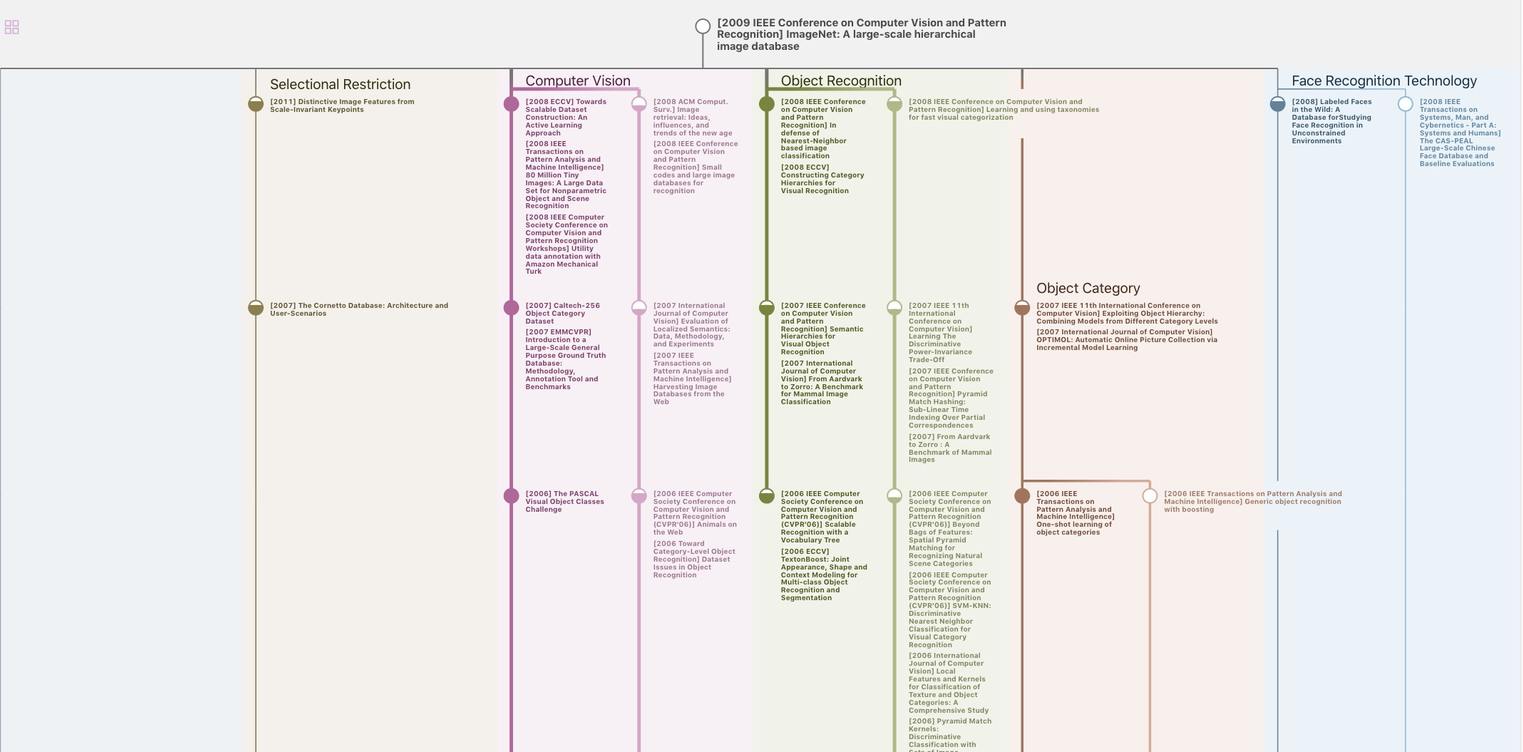
生成溯源树,研究论文发展脉络
Chat Paper
正在生成论文摘要