Urban form features determine spatio-temporal variation of ambient temperature: A comparative study of three European cities
Urban Climate(2023)
摘要
Driven by ongoing urbanization and accelerated global warming, urban heat stress develops into a major threat for a large part of the world population. While the literature is clear about the relevance of urban form in modifying urban heat, the spatially explicit context of heat modifications by the built environment is insufficiently explored across cities. Here we develop a consistent methodology based on a machine learning model to determine the predictive features of urban form that shape urban ambient temperature (AT) under different urban and climate conditions. For this, we combine urban climate data at 100 m resolution from the UrbClim model with urban form features computed from different open datasets. We exemplify our innovation by evaluating summer daytime and nighttime temperature variation within three European cities that are representative of distinct geographies and climates: Berlin, Zurich, and Sevilla. Mean AT at dense urban spots was higher by 3 degrees C (on average) during summer compared to sub-urban sites across all cities. We find that urban form features explain around 2/3 of within-city temperature variations. Vegetation cover and water bodies are most significant in explaining spatial temperature patterns during the daytime, while the impervious land cover is most critical at nighttime. Cooling effect of water areas can accumulate to-1.5 degrees C, while heating effects of roads can induce +0.5 degrees C during daytime. The magnitude of effects varies across cities, primarily based on the climate type, altitude, and dominant land-cover. However, further testing should be conducted to develop a better understanding of impact variations. The findings of this research will provide urban planners and policymakers with crucial insights towards heat mitigation and sustainable urban developments through region-tailored modifications and replacement of land-cover.
更多查看译文
关键词
Urban heat,Land use,Machine learning,Heat mitigation
AI 理解论文
溯源树
样例
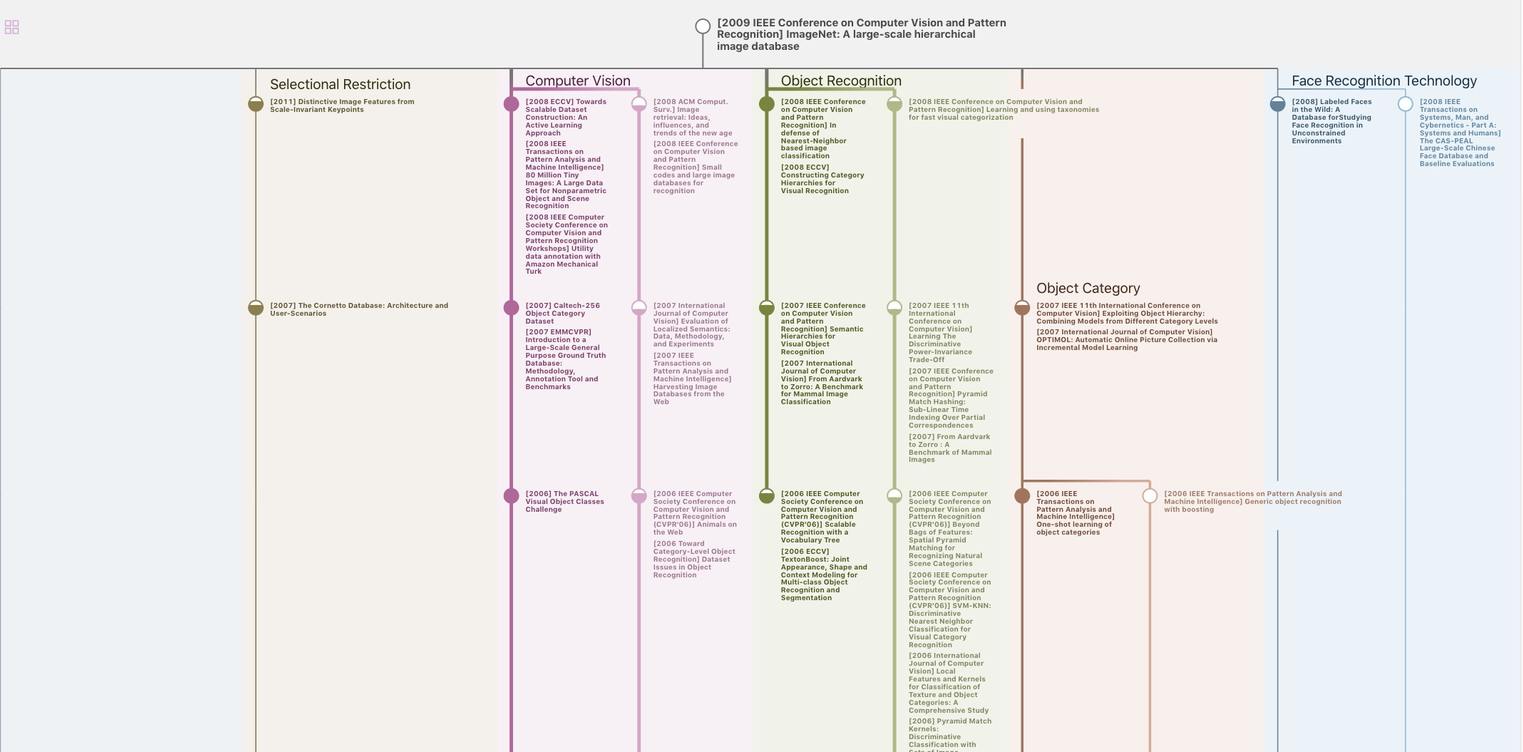
生成溯源树,研究论文发展脉络
Chat Paper
正在生成论文摘要