Deep learning characterization of rock conditions based on tunnel boring machine data
Underground Space(2023)
摘要
Rock condition perception based on tunnel boring machine (TBM) data is of great importance for not only ensuring tunnel boring safety but also improving construction efficiency. The prediction of TBM boring responses (i.e., torque and total thrust of the cutterhead) largely determines the reliability of rock condition perception. In this paper, a new architecture of a two-dimensional convolutional neural network (2D-CNN) with a dual-input strategy is proposed to predict the TBM responses. The TBM Lot 3 of the Yinsong project in Jilin province, China, is taken as the case study in this paper. Two types of models that follow different learning strategies are compared: one is defined as the point model, which only learns data of the stable phase, and the other is defined as the line model, which learns data from both the loading and stable boring phases. The line model is further improved by the weighted loss function method. The results indicate that the strategy of learning data from both the loading phase and stable boring phase and increasing the weight of samples from the stable phase is shown to be optimal in predicting TBM boring responses. In terms of learning strategies, the line model can learn the influence of active control parameters on passive response parameters, but the point model cannot. In terms of machine learning algorithms, 2D-CNN has the best performance, with R2 values of 0.865 and 0.923 for torque and total thrust, respectively. The proposed line model can overcome the problem that the traditional model failed to learn the influence of control parameters. Such a model can provide a solid base for the timely optimization of the control parameters in TBM boring process.
更多查看译文
关键词
rock conditions,deep learning,tunnel,boring machine data
AI 理解论文
溯源树
样例
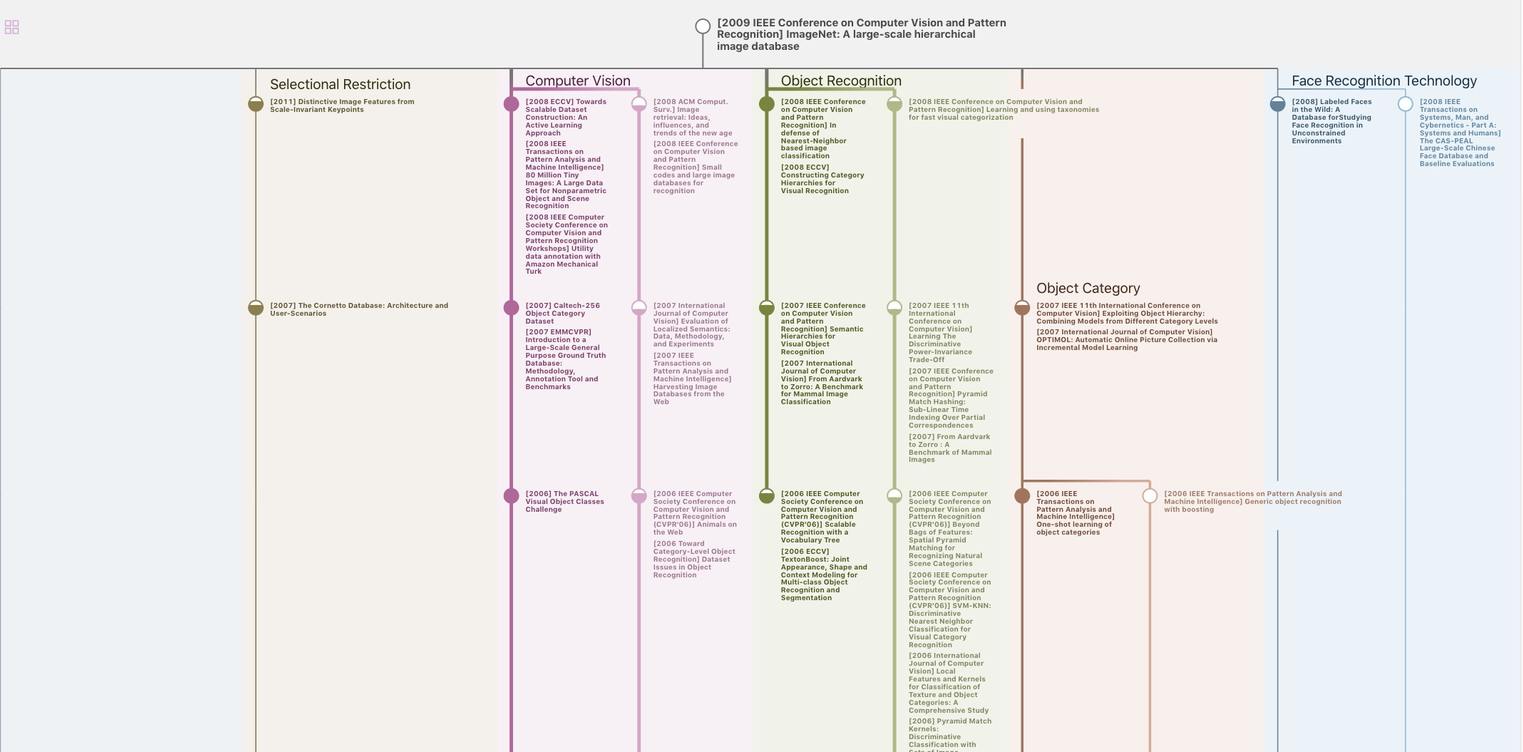
生成溯源树,研究论文发展脉络
Chat Paper
正在生成论文摘要