Combining predictive and prescriptive techniques for optimizing electric vehicle fleet charging
Transportation Research Part C: Emerging Technologies(2023)
摘要
The last decade has witnessed a burgeoning interest in transportation electrification from the academia, government, and industry. A current barrier faced by fleet operators is the charge scheduling, a problem that becomes more pronounced with the fleet size, heterogeneity (in both the vehicle fleet and the charging infrastructure), and uncertainty, which has given rise to the Charging-as-a-Service (CaaS) industry. A CaaS provider intermediates between the fleet owner and the macrogrid, and is key to ease the transition to the future of transportation with electric vehicles. This paper addresses the CaaS providers’ electric vehicle fleet (EVF) charge scheduling problem with time-varying electricity prices. We develop a rolling-horizon online optimization approach reinforced with a predictive model and a heuristic warm-start to solve this emerging multi-stage stochastic optimization problem. A numerical experiment demonstrates that our method outperforms an industry benchmark by 13.24%–18.44% with respect to charging costs under the tested conditions. In addition to cost savings, the energy use profile of resulting schedules consumes less energy in peak hours, which can reduce carbon emissions and improve grid stability. Thus, the proposed approach identifies charging schedules that simultaneously benefit CaaS providers, fleet owners, electric power producers, and the macrogrid in general.
更多查看译文
关键词
Transportation, Sustainability, Electric vehicle charging, Mathematical programming, Predictive modeling
AI 理解论文
溯源树
样例
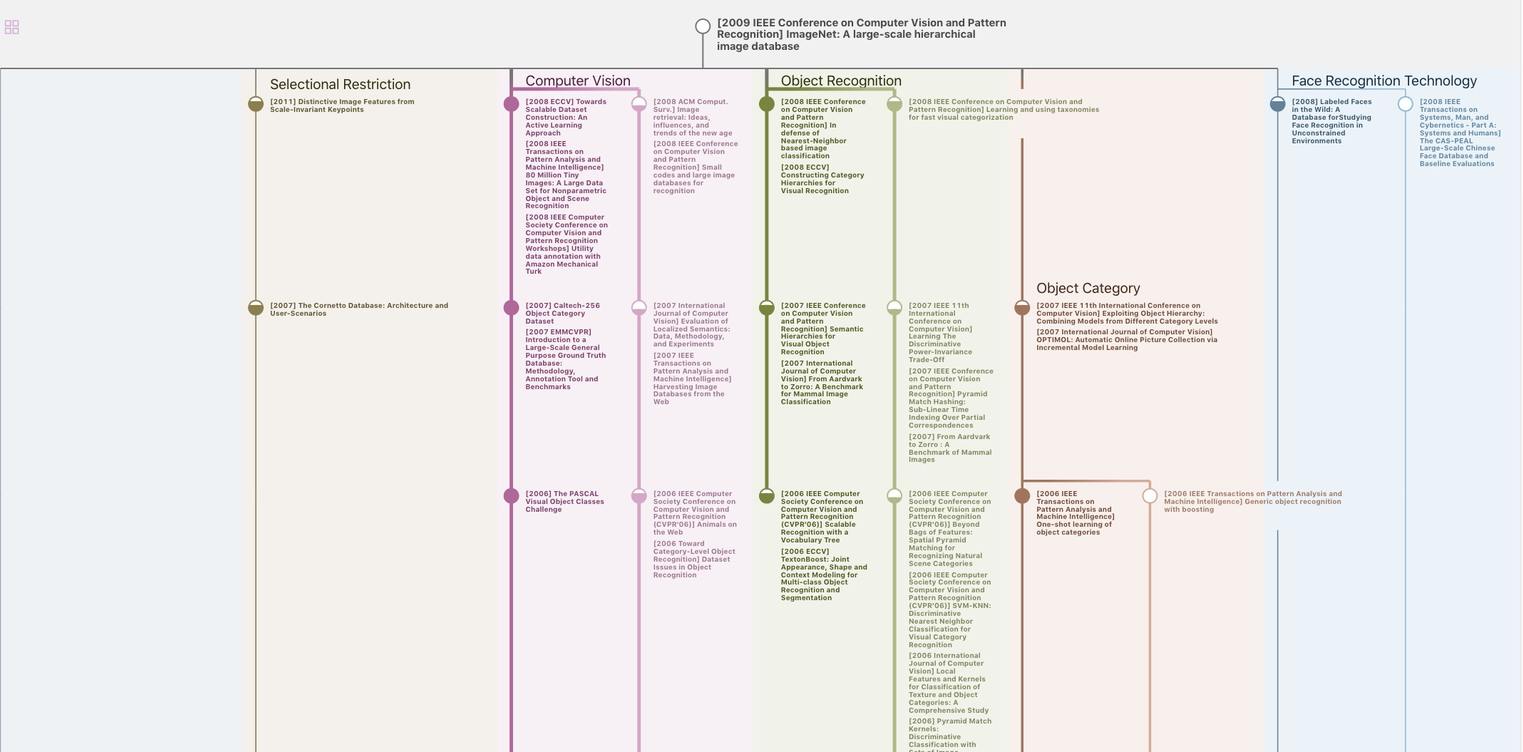
生成溯源树,研究论文发展脉络
Chat Paper
正在生成论文摘要