Air traffic density prediction using Bayesian ensemble graph attention network (BEGAN)
Transportation Research Part C: Emerging Technologies(2023)
摘要
Air traffic density prediction is crucial to aviation safety and air traffic management (ATM). Understanding the complex spatial–temporal varying traffic patterns and the inter-dependencies of air traffic networks is especially demanding. To address these challenges, a novel knowledge-based deep learning framework, Bayesian ensemble graph attention network (BEGAN), is proposed to leverage the interactions of traffic density at different airspace grids and predict stochastic traffic density near the terminal. The core concept of BEGAN is to divide the large airspace into small overlapped gridded sections for individual training, and then assemble posterior distributions of density forecasts for large-scale prediction. The ensemble method improves the scalability of handling heterogeneous air traffic flow patterns. Algorithm-wise, a weighted directed graph interleaves the connection between different gridded airspaces. A parallel graph attention network (P-GAT) block followed by the long short-term memory (LSTM) network is designed to learn the spatial–temporal dependencies of the graph. Notably, P-GAT can incorporate existing domain knowledge into data-driven learning, such as historical trajectory paths, future flight plans, and airspace restrictions. The inclusion of existing knowledge and rules proves beneficial for air traffic density prediction in heavily regulated and controlled airspace. We evaluate BEGAN with real-world data collected from Hartsfield-Jackson Atlanta International Airport (KATL) and Orlando International Airport (KMCO). Extensive experiments show that the proposed framework can make accurate and robust predictions, outperforming nine baseline models. Based on the proposed study, limitations and future research directions are given.
更多查看译文
关键词
air traffic density prediction,ensemble,graph
AI 理解论文
溯源树
样例
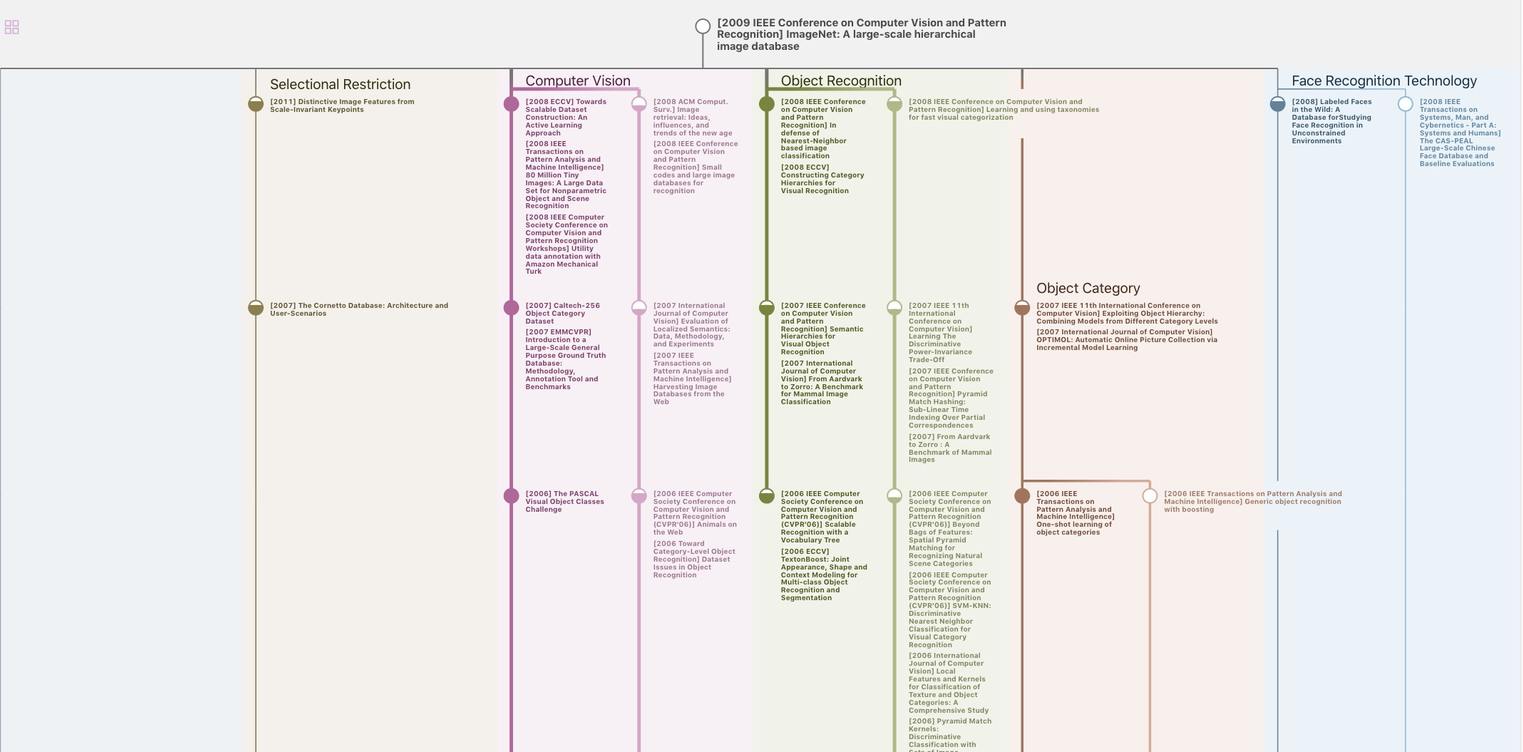
生成溯源树,研究论文发展脉络
Chat Paper
正在生成论文摘要