A Weibit-Based sequential transit assignment model based on hyperpath graph and generalized extreme value network representation
Transportation Research Part C: Emerging Technologies(2023)
摘要
This study aims to develop a weibit-based stochastic transit assignment model for situations in which passengers' travel choice strategies are stochastic. The weibit choice model, which was originally developed for the stochastic traffic assignment problem, is adapted using a hyperpath graph network representation. Unlike the traditional logit model, the weibit model can account for the homogeneous perception variance problem. Besides, a joint choice network representation originated from the network generalized extreme value (N-GEV) model, known as the GEV network, is adopted to depict the correlation among the arcs within the hypergraph consisting of all efficient hyperpaths. The GEV network uses a link-based parameter, the degree of membership, to capture the overlap effect, which is thus well incorporated with the link-based transit assignment approach. For implementation, a link-based stochastic network loading approach is proposed for solving the weibit-based transit assignment model in the sequential process. This sequential assignment approach does not need to enumerate and store the transit hyperpaths in the solution process. This model is then extended to a stochastic transit equilibrium model under congestion conditions, which is formulated as a fixed-point problem. Thus, a recent fast linesearch scheme, i.e., the Barzilai and Borwein step-size, is adopted for solving the stochastic transit equilibrium model. Numerical examples are provided to illustrate the features of the proposed weibit-based stochastic transit assignment model. A real transit network is used to demonstrate the algorithm efficiency and the detailed results of the weibit-based transit equilibrium model.
更多查看译文
关键词
Stochastic transit assignment,Weibit model,Fixed point,Network generalized extreme value,Hyperpath graph
AI 理解论文
溯源树
样例
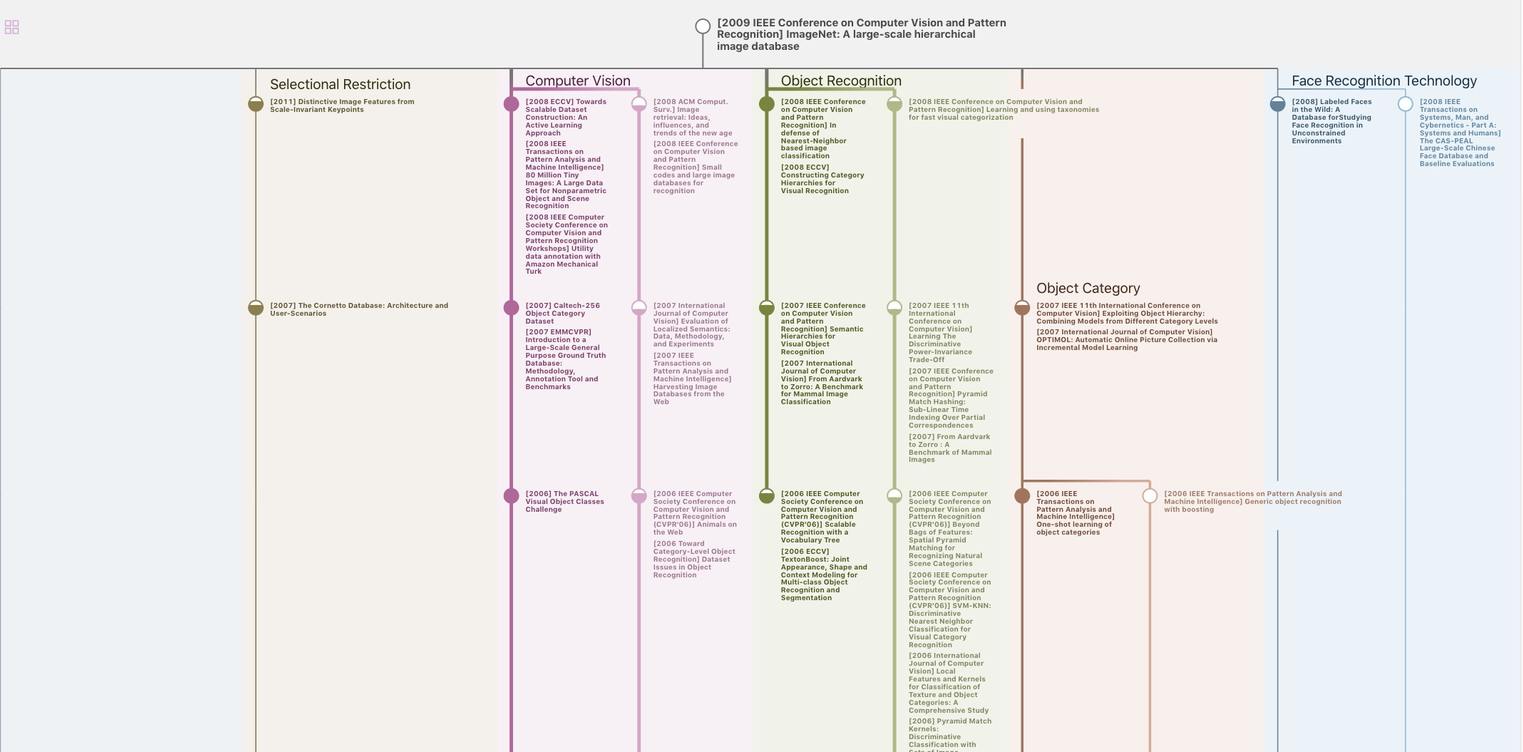
生成溯源树,研究论文发展脉络
Chat Paper
正在生成论文摘要