The fundamental importance of exploring the risks alongside the benefits of artificial intelligence.
Journal of hepatology(2023)
摘要
Artificial intelligence, machine learning, and deep learning in liver transplantationJournal of HepatologyVol. 78Issue 6PreviewLiver transplantation (LT) is a life-saving treatment for individuals with end-stage liver disease. The management of LT recipients is complex, predominantly because of the need to consider demographic, clinical, laboratory, pathology, imaging, and omics data in the development of an appropriate treatment plan. Current methods to collate clinical information are susceptible to some degree of subjectivity; thus, clinical decision-making in LT could benefit from the data-driven approach offered by artificial intelligence (AI). Full-Text PDF Open Access PB- Writing, creating the infographic and reviewing the manuscript. SK- Writing the manuscript and creating the infographic. No conflicts of interest. No financial support or competing interests. Bhat et al present convincing examples and arguments for the benefits of artificial intelligence (AI) in the field of liver transplantation, from recipient selection and graft matching to post-transplantation management.[1]Bhat M. Rabindranath M. Chara B.S. Simonetto D.A. Artificial intelligence, machine learning, and deep learning in liver transplantation.J Hepatol. 2023 Jun; 78 (PMID: 37208107): 1216-1233https://doi.org/10.1016/j.jhep.2023.01.006Abstract Full Text Full Text PDF PubMed Scopus (4) Google Scholar However, discussion around potential risks is limited. Even though the evidence they present is based on contained learning set, it must be recognised that we are experiencing a wave of anxiety related to AI, and in our view, it is vital that any exploration of AI in medicine explores possible downsides transparently and comprehensively. Prakash et al summarised the ethical conundrums raised by introducing AI based on large language models (LLM) to medicine, identifying fairness and justice as important matters.[2]Prakash S. Balaji J.N. Joshi A. Surapaneni K.M. Ethical Conundrums in the Application of Artificial Intelligence (AI) in Healthcare-A Scoping Review of Reviews.J Pers Med. 2022 Nov 16; 12 (PMID: 36422090; PMCID: PMC9698424): 1914https://doi.org/10.3390/jpm12111914Crossref PubMed Scopus (10) Google Scholar Respecting these principles is especially important in the field of transplantation, where there are existing, unresolved issues related to blanket scoring systems and equity of access across geographical boundaries or between ethnic and gender groups.[3]Berry P. Kotha S. Diminished autonomy and justice in liver transplantation – The price of scarcity?.Clinical Ethics. 2021; 16: 291-297https://doi.org/10.1177/14777509211016286Crossref Scopus (0) Google Scholar The main risk from AI based on LLM is bias. The authors acknowledge this but only insofar as to reassure us that de-biasing methods have been developed. More detail is required. We know that certain groups (ethnic minorities, LGBTQIA+ individuals, pregnant women, and older people) are not well represented in the existing evidence base, for instance in drug or vaccine trials.[4]Treweek S. Banister K. Bower P. Cotton S. Devane D. Gardner H.R. Isaacs T. Nestor G. Oshisanya A. Parker A. Rochester L. Soulsby I. Williams H. Witham M.D. Developing the INCLUDE Ethnicity Framework-a tool to help trialists design trials that better reflect the communities they serve.Trials. 2021 May 10; 22 (PMID: 33971916; PMCID: PMC8108025): 337https://doi.org/10.1186/s13063-021-05276-8Crossref PubMed Scopus (24) Google Scholar Language itself may be skewed; Caliskan and colleagues, writing in Science, found that negative stereotypes were prevalent in bodies of text on which LLMs derive their predictions.[5]Caliskan A. Bryson J.J. Narayanan A. Semantics derived automatically from language corpora contain human-like biases.Science. 2017 Apr 14; 356 (PMID: 28408601): 183-186https://doi.org/10.1126/science.aal4230Crossref PubMed Scopus (1230) Google Scholar Even on a smaller scale than LLM, bias has been detected in the output of algorithms that work on fallible categories or labels; in a US study of 50,000 patients, an AI health economics tool used healthcare expenditure as a proxy for health and concluded that Black people are healthier than they really are; an underlying inequity of access to healthcare was misinterpreted.[6]Obermeyer Z. Powers B. Vogeli C. Mullainathan S. Dissecting racial bias in an algorithm used to manage the health of populations.Science. 2019 Oct 25; 366 (PMID: 31649194): 447-453https://doi.org/10.1126/science.aax2342Crossref PubMed Scopus (1593) Google Scholar We do not know, at present, how the highly complex algorithms on which recommendations for medical treatment will be based may be influenced by hard to detect drifts towards pre-existing societal inequities. Immunisation of AI systems against these pitfalls is critically important. Therefore, it is necessary to forecast how proposed AI applications will fit into existing or developing ethical governance structures.[7]Zhang J. Zhang Z.M. Ethics and governance of trustworthy medical artificial intelligence.BMC Med Inform Decis Mak. 2023 Jan 13; 23 (PMID: 36639799; PMCID: PMC9840286): 7https://doi.org/10.1186/s12911-023-02103-9Crossref PubMed Scopus (15) Google Scholar Safety measures such as continuous audit of outcomes and third-party analysis will be required; for contained models, larger fears such as sentience, conflicting goals, instrumental convergence or malevolent intent should be less relevant.[8]Bostrom N. The superintelligent will: motivation and instrumental rationality in advanced artificial agents.Minds and Machines. May 2012; 22Crossref Scopus (99) Google Scholar These concepts may be sound overblown, even hysterical, in the context of the applications presented by Bhat et al, but they do inform the zeitgeist. Addressing concerns will go some way to allaying the uncertainties and fears being experience by the public who must, at the end of the day, give permission for us to employ AI in day-to-day decision making. We might learn from the divided response to vaccination against COVID-19, where new technologies (e.g. mRNA-based vaccines) were poorly understood and ultimately rejected by many. While Bhat et al successfully describe AI’s undoubted positives, and are cause for great optimism and excitement, more space and time is required to bring healthcare workers and patients along with them. AI has the potential to shape an exciting future for transplantation by improving organ allocation, donor selection, prediction of rejection and precision medicine for immunosuppression; however, it requires a cautious approach with active governance to detect and avoid bias, an awareness of potential inequities and patient engagement to determine the limits of its role in medical decisions of great consequence. Potential benefits and risks of AI applications in liver transplantation are shown in Table 1.Table 1Benefits and risks of AI applications in liver transplantation.PhaseApplicationBenefitsRisksEvidence of patient benefit/safety requiredEthical consensus required#including public engagement.Pre-transplantDiagnosisValidation of clinical diagnosis based on EHR, laboratory data and imagingLikely low (questionable added value in this field)●PrognosisSurvival without LT; modelling HCC biology; monitoring response/progression after locoregional therapy; impact of acute complications of cirrhosis; refining ALF models; risk stratification of progression of fibrosis in NAFLD and viral hepatitisQualitative data inputs requiring agreed criteria to ensure equity across regions; high-quality data inputs required to ensure accurate output●Transplantation assessment and candidacyPersonalised outcome estimation (utility); 1 and 5-year survival; likelihood of HCC/primary disease recurrenceDiminished influence of individual patient goals/values; difficulty measuring quality of life outcomes; requirement for human judgement in ethically challenging cases (re-transplantation, active alcohol use, social support, adherence); bias inherent in data may exacerbates inequities (especially in minority groups); ‘explainability’ of decisions to patients; unclear how appeals would be handled●Prioritisation (urgency)Week-by-week or real-time assessment; updated waitlist mortality estimation; reduced subjectivity; rapid pan-national or international comparisonsTrust in ‘black-box’ mechanisms required; ‘explainability’; agreement needed on weight given to evolving symptoms (e.g. fatigue) or complications (e.g. HPS, HCC)●Peri-operativeDonor-recipient matchingRapid calculation of transplant benefit considering recipient and donor factorsAgreeing relative weight of experienced opinion vs AI-generated outcomes; loss of subjective component (emotional) of clinical decision making●●Image analysis and virtual surgical planningVolumetric assessment; vascular anatomy; graft steatosisTechnical; need to develop new ways of working to incorporate AI-based advice into clinical decision making; clarify lines of responsibility/accountability if AI driven decision results in poor surgical outcome●●Post-transplantEarly post-operative monitoringReal-time analysis of vital signs, lab results; detecting subtle changes or early signs of complications; early recognition of primary non-function with relisting; reduced length of stay in hospitalEvidence base required if AI suggesting major change of management (e.g. re-transplantation) prior to human clinical decision; challenge of integrating into existing clinical systems●Personalised therapeutics/immunosuppressionAnticipating drug-drug interactions; pharmacokinetic; dosage recommendations; recipients’ genome, gene expression analysis; risk stratification for long term adverse effects (cardiovascular, neoplasia, opportunistic infection); optimise patient and graft survivalDiminished clinical skillset (not limited to this field); integration into existing clinical systems●Post-LT survivalBetter predictive accuracy compared to MELD, BAR or SOFT scores; AI can be trained to predict survival accurately in different populationsPotential inaccuracies due to imperfect datasets●Strategic/organisationalInternet of things (IOT)Remote patient monitoring (wearables/implants), organ transport and tracking, real-time communication and data sharing between transplant centres, organ procurement organizations, and donor registriesData security and privacy; physical reliability●Research and developmentAnalysis of large-scale clinical and -omics datasets; detection of novel patterns; federated and ‘swarm’ learningHeterogeneity of data across organisations; ownership; privacy; need for agreed governance protocols, clarify standard for evidence prior to implementation●●Health economicsOptimised utilisation of resources and donor organs; reduced length of stay; reduced graft failure and re-transplantationCaution required around the optics of linking AI in healthcare to savings & diminished trust); clarification of who benefits from savings (AI developers, health organisations, governments)●Abbreviations: EHR – electronic health records; LT – liver transplantation; HCC – hepatocellular carcinoma; HPS – hepatopulmonary syndrome; MELD – model for end stage liver disease; BAR - Balance of Risk Score, SOFT - Survival Outcomes Following Liver Transplantation.# including public engagement. Open table in a new tab Abbreviations: EHR – electronic health records; LT – liver transplantation; HCC – hepatocellular carcinoma; HPS – hepatopulmonary syndrome; MELD – model for end stage liver disease; BAR - Balance of Risk Score, SOFT - Survival Outcomes Following Liver Transplantation.
更多查看译文
关键词
Liver transplantation,AI,machine learning
AI 理解论文
溯源树
样例
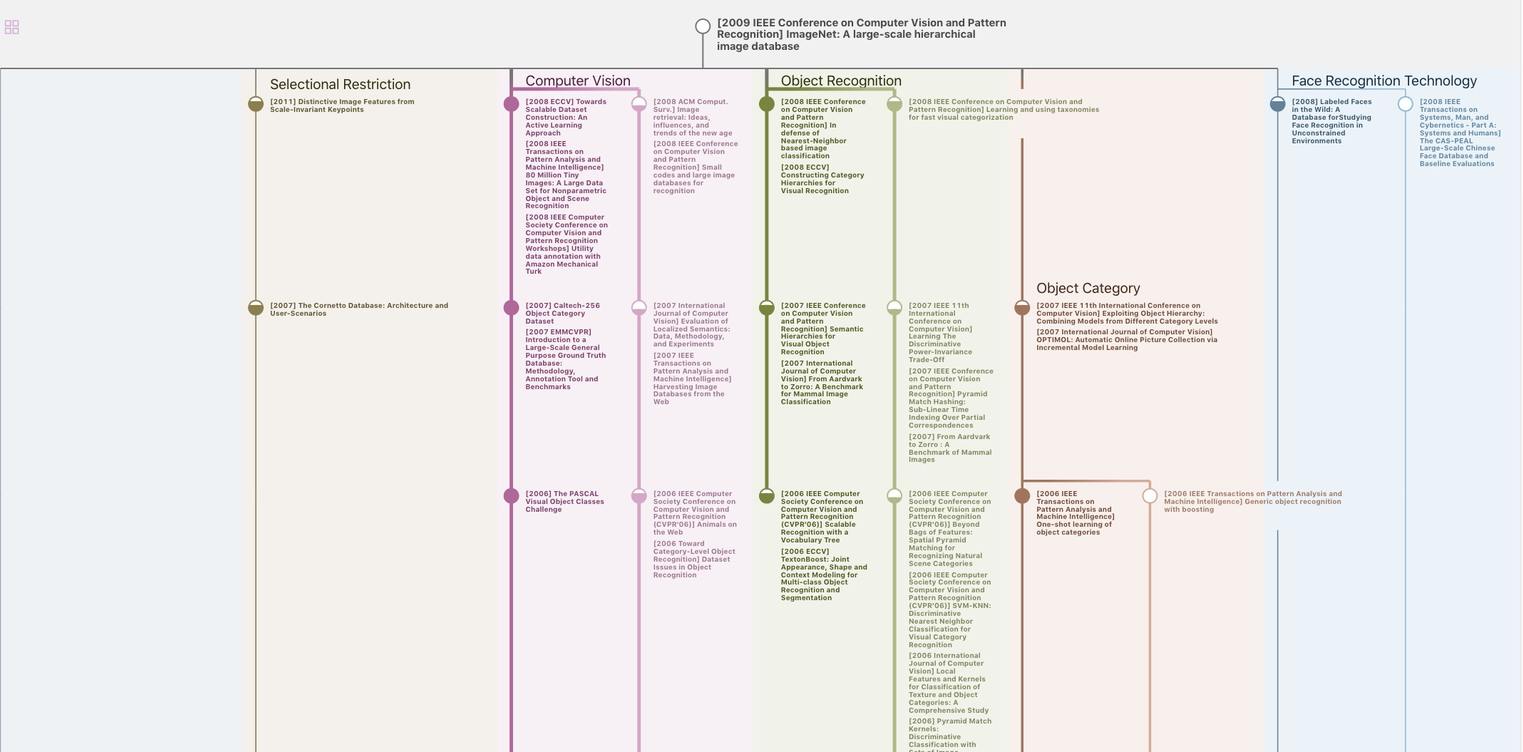
生成溯源树,研究论文发展脉络
Chat Paper
正在生成论文摘要