On-board Federated Learning for Satellite Clusters with Inter-Satellite Links
CoRR(2023)
摘要
The emergence of mega-constellations of interconnected satellites has a major
impact on the integration of cellular wireless and non-terrestrial networks,
while simultaneously offering previously inconceivable data gathering
capabilities. This paper studies the problem of running a federated learning
(FL) algorithm within low Earth orbit satellite constellations connected with
intra-orbit inter-satellite links (ISL), aiming to efficiently process
collected data in situ. Satellites apply on-board machine learning and transmit
local parameters to the parameter server (PS). The main contribution is a novel
approach to enhance FL in satellite constellations using intra-orbit ISLs. The
key idea is to rely on predictability of satellite visits to create a system
design in which ISLs mitigate the impact of intermittent connectivity and
transmit aggregated parameters to the PS. We first devise a synchronous FL,
which is extended towards an asynchronous FL for the case of sparse satellite
visits to the PS. An efficient use of the satellite resources is attained by
sparsification-based compression the aggregated parameters within each orbit.
Performance is evaluated in terms of accuracy and required data transmission
size. We observe a sevenfold increase in convergence speed over the
state-of-the-art using ISLs, and 10× reduction in communication load
through the proposed in-network aggregation strategy.
更多查看译文
关键词
Low Earth orbit,mega-constellations,intra-orbit inter-satellite links,federated learning,sparsification
AI 理解论文
溯源树
样例
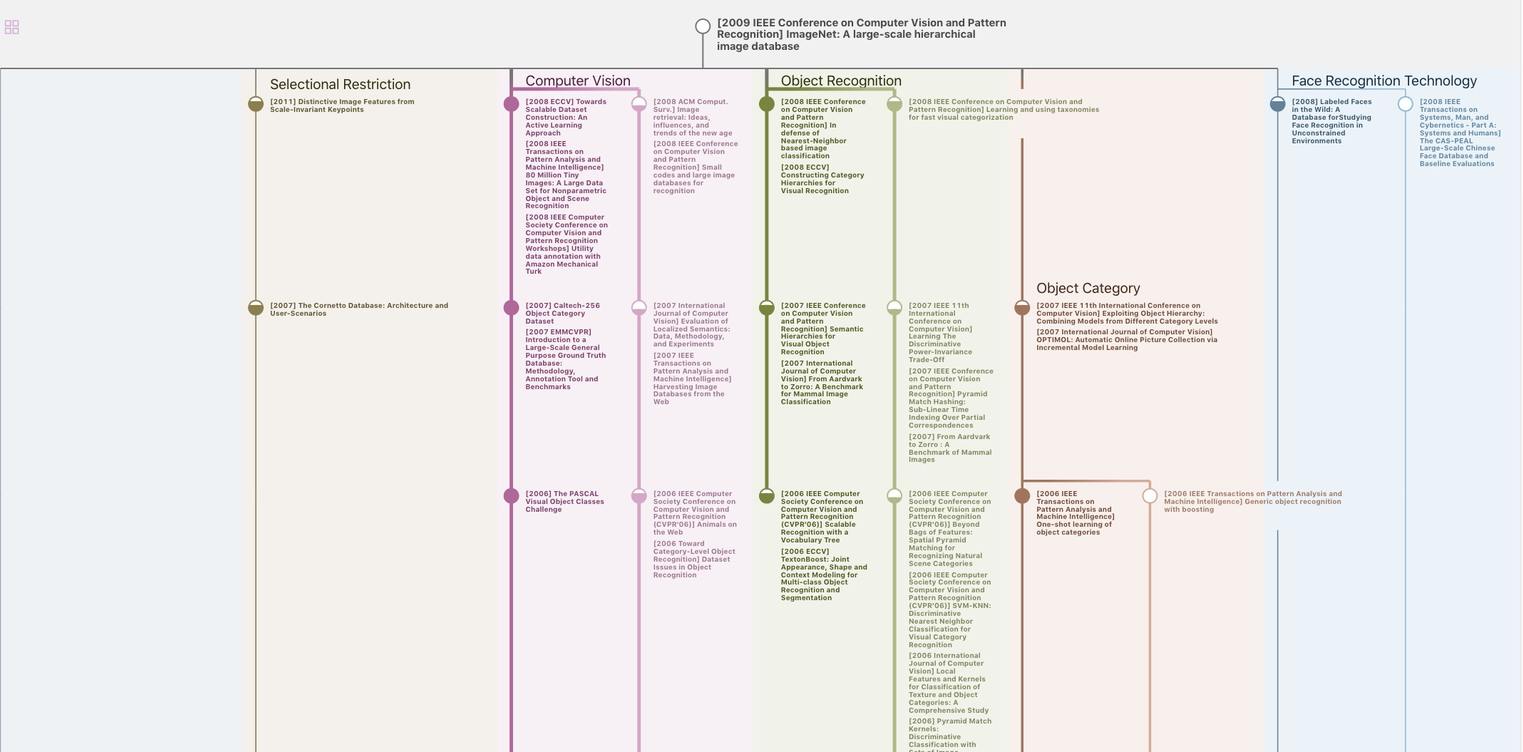
生成溯源树,研究论文发展脉络
Chat Paper
正在生成论文摘要