Soft Curriculum for Learning Conditional GANs with Noisy-Labeled and Uncurated Unlabeled Data
2024 IEEE/CVF Winter Conference on Applications of Computer Vision (WACV)(2023)
摘要
Label-noise or curated unlabeled data is used to compensate for the assumption of clean labeled data in training the conditional generative adversarial network; however, satisfying such an extended assumption is occasionally laborious or impractical. As a step towards generative modeling accessible to everyone, we introduce a novel conditional image generation framework that accepts noisy-labeled and uncurated unlabeled data during training: (i) closed-set and open-set label noise in labeled data and (ii) closed-set and open-set unlabeled data. To combat it, we propose soft curriculum learning, which assigns instance-wise weights for adversarial training while assigning new labels for unlabeled data and correcting wrong labels for labeled data. Unlike popular curriculum learning, which uses a threshold to pick the training samples, our soft curriculum controls the effect of each training instance by using the weights predicted by the auxiliary classifier, resulting in the preservation of useful samples while ignoring harmful ones. Our experiments show that our approach outperforms existing semi-supervised and label-noise robust methods in terms of both quantitative and qualitative performance. In particular, the proposed approach is able to match the performance of (semi-) supervised GANs even with less than half the labeled data.
更多查看译文
关键词
Algorithms,Generative models for image,video,3D,etc.,Algorithms,Computational photography,image and video synthesis
AI 理解论文
溯源树
样例
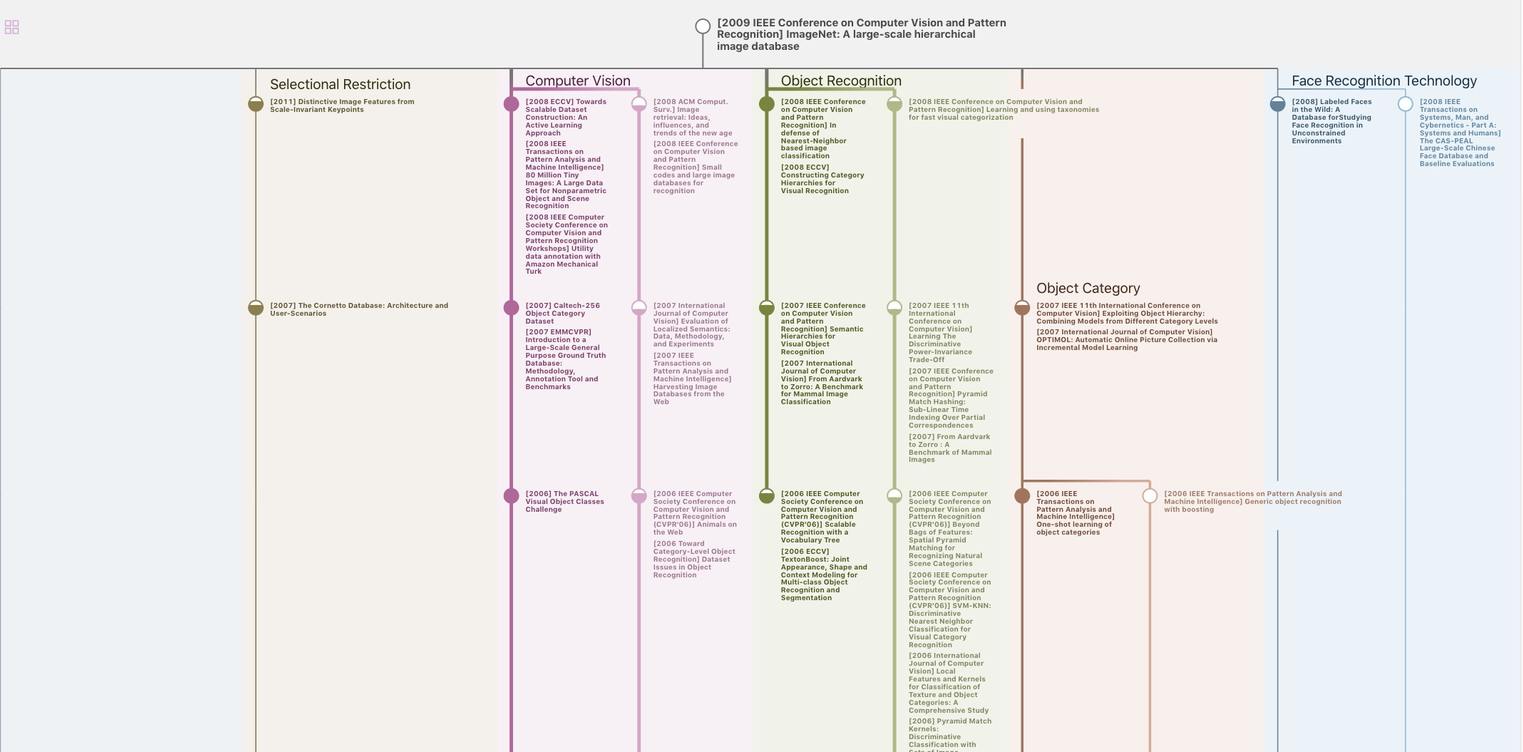
生成溯源树,研究论文发展脉络
Chat Paper
正在生成论文摘要