CalibNet: Dual-branch Cross-modal Calibration for RGB-D Salient Instance Segmentation
CoRR(2023)
摘要
We propose a novel approach for RGB-D salient instance segmentation using a dual-branch cross-modal feature calibration architecture called CalibNet. Our method simultaneously calibrates depth and RGB features in the kernel and mask branches to generate instance-aware kernels and mask features. CalibNet consists of three simple modules, a dynamic interactive kernel (DIK) and a weight-sharing fusion (WSF), which work together to generate effective instance-aware kernels and integrate cross-modal features. To improve the quality of depth features, we incorporate a depth similarity assessment (DSA) module prior to DIK and WSF. In addition, we further contribute a new DSIS dataset, which contains 1,940 images with elaborate instance-level annotations. Extensive experiments on three challenging benchmarks show that CalibNet yields a promising result, i.e., 58.0% AP with 320*480 input size on the COME15K-N test set, which significantly surpasses the alternative frameworks. Our code and dataset are available at: https://github.com/PJLallen/CalibNet.
更多查看译文
关键词
segmentation,calibration,dual-branch,cross-modal
AI 理解论文
溯源树
样例
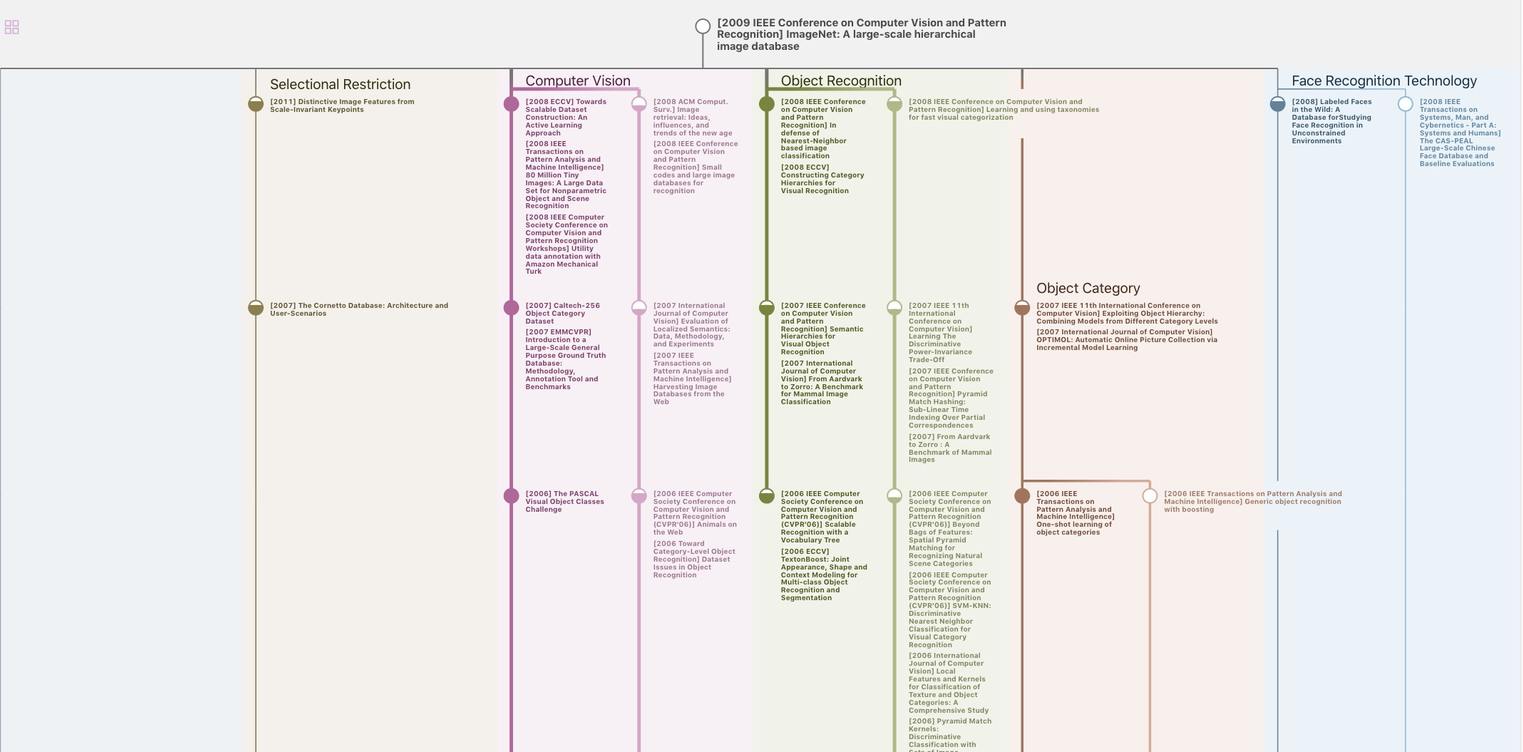
生成溯源树,研究论文发展脉络
Chat Paper
正在生成论文摘要