Byzantine-Robust Distributed Online Learning: Taming Adversarial Participants in An Adversarial Environment
IEEE TRANSACTIONS ON SIGNAL PROCESSING(2024)
摘要
This paper studies distributed online learning under Byzantine attacks. The performance of an online learning algorithm is often characterized by (adversarial) regret, which evaluates the quality of one-step-ahead decision-making when an environment incurs adversarial losses, and a sublinear regret bound is preferred. But we prove that, even with a class of state-of-the-art robust aggregation rules, in an adversarial environment and in the presence of Byzantine participants, distributed online gradient descent can only achieve a linear adversarial regret bound, which is tight. This is the inevitable consequence of Byzantine attacks, even though we can control the constant of the linear adversarial regret to a reasonable level. Interestingly, when the environment is not fully adversarial so that the losses of the honest participants are i.i.d. (independent and identically distributed), we show that sublinear stochastic regret, in contrast to the aforementioned adversarial regret, is possible. We develop Byzantine-robust distributed online momentum algorithms to attain such sublinear stochastic regret bounds for a class of robust aggregation rules. Numerical experiments corroborate our theoretical analysis.
更多查看译文
关键词
Distributed databases,Distributed optimization,Byzantine-robustness,online learning
AI 理解论文
溯源树
样例
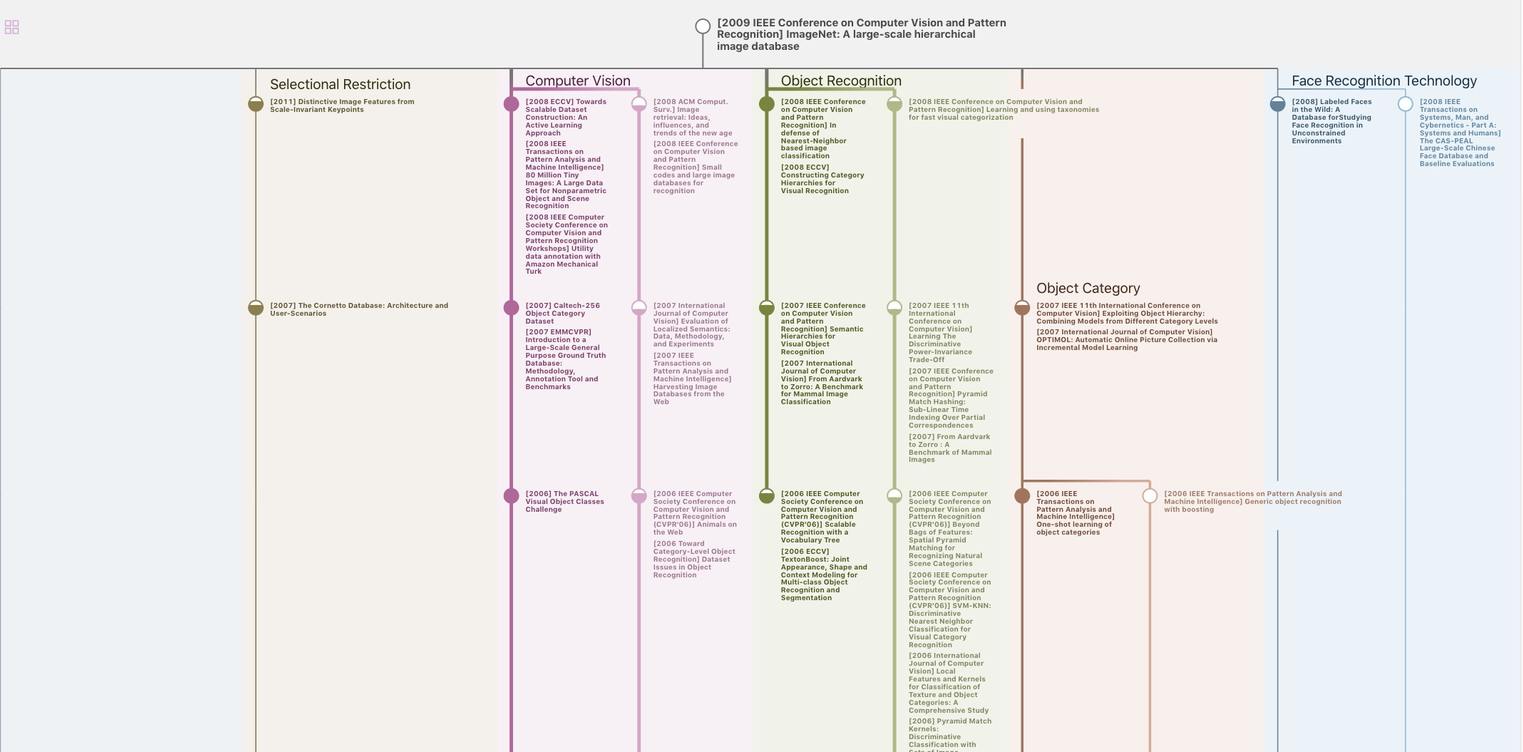
生成溯源树,研究论文发展脉络
Chat Paper
正在生成论文摘要