Data-Driven Optimal Control of Tethered Space Robot Deployment with Learning Based Koopman Operator
arXiv (Cornell University)(2023)
Abstract
To avoid complex constraints of the traditional nonlinear method for tethered space robot (TSR) deployment, this paper proposes a data-driven optimal control framework with an improved deep learning based Koopman operator that could be applied to complex environments. In consideration of TSR's nonlinearity, its finite dimensional lifted representation is derived with the state-dependent only embedding functions in the Koopman framework. A deep learning approach is adopted to approximate the global linear representation of TSR. Deep neural networks (DNN) are developed to parameterize Koopman operator and its embedding functions. An auxiliary neural network is developed to encode the nonlinear control term of finite dimensional lifted system. In addition, the state matrix A and control matrix B of lifted linear system in the embedding space are also estimated during training DNN. Then three loss functions that related to reconstruction and prediction ability of network and controllability of lifted linear system are designed for training the entire network. With the global linear system produced from DNN, Linear Quadratic Regulator (LQR) is applied to derive the optimal control policy for the TSR deployment. Finally, simulation results verify the effectiveness of proposed framework and show that it could deploy tethered space robot more quickly with less swing of in-plane angle.
MoreTranslated text
Key words
tethered space robot deployment,learning based koopman operator,optimal control,data-driven
AI Read Science
Must-Reading Tree
Example
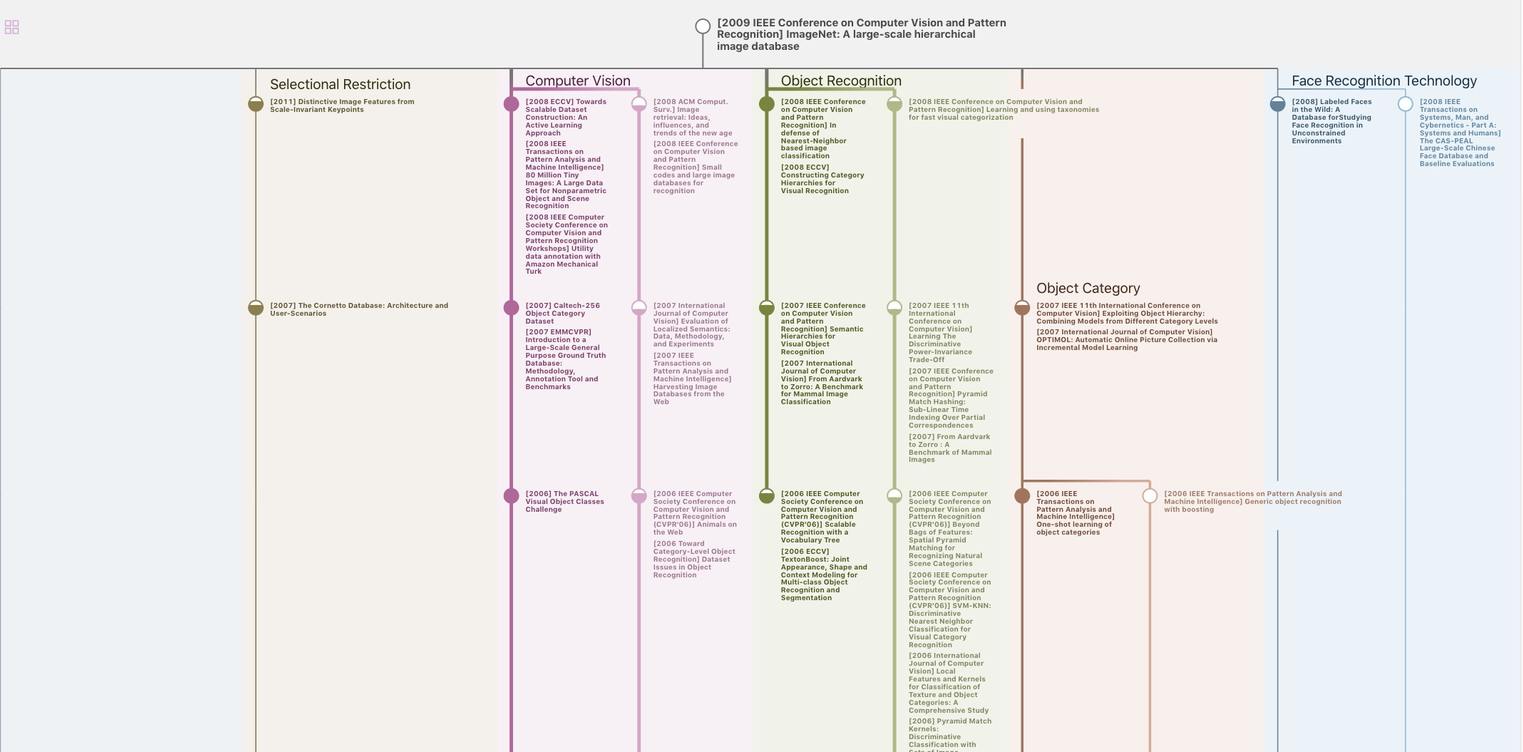
Generate MRT to find the research sequence of this paper
Chat Paper
Summary is being generated by the instructions you defined