Enhanced Building Prediction for Smart City Surveillance
2023 International Conference on Advances in Electronics, Communication, Computing and Intelligent Information Systems (ICAECIS)(2023)
摘要
Nearly three-quarters of the total power used in the world is consumed by the built environment sector. Therefore, looking for viable methods to reduce building energy needs and decrease negative environmental repercussions is vital. By incorporating intelligence into applications, everyone in the current situation has become intelligent, which lessens the load of constant interruption or human control. By using Machine to Machine interactions, intelligence enables the ability to connect more real-time parameters and make moral judgments in perilous circumstances. The current study examined the framework, approach, and performance of currently available AI-based techniques. According to the research, choosing the best machine and deep learning model for a given task can be difficult. Among the primary areas of study in relation to the vast amounts of data collected is the viability in the practice of the used algorithms, both standard machine learning and the more recent branch known as deep learning. Present a quantitative analysis contrasting the effectiveness of conventional deep learning and machine learning strategies using the direction of human movement in detecting the applications based on the analogue pyroelectric infrared sensor (PIR) outputs. Here, Random Forest is used as the machine learning method because of its great prediction accuracy. Over deeper datasets, deeper CNNs outperform shallow models as a deep learning method. The study demonstrates that traditional Machine Learning performs with detecting values within the first few seconds, improving real-time detection. Additionally, it might be used on more smart buildings without upgrades.
更多查看译文
关键词
Machine Interactions,AI,Machine Learning,Deep Learning,Pyroelectric Infrared Sensors,Random Forest,Deeper CNN,Smart Building
AI 理解论文
溯源树
样例
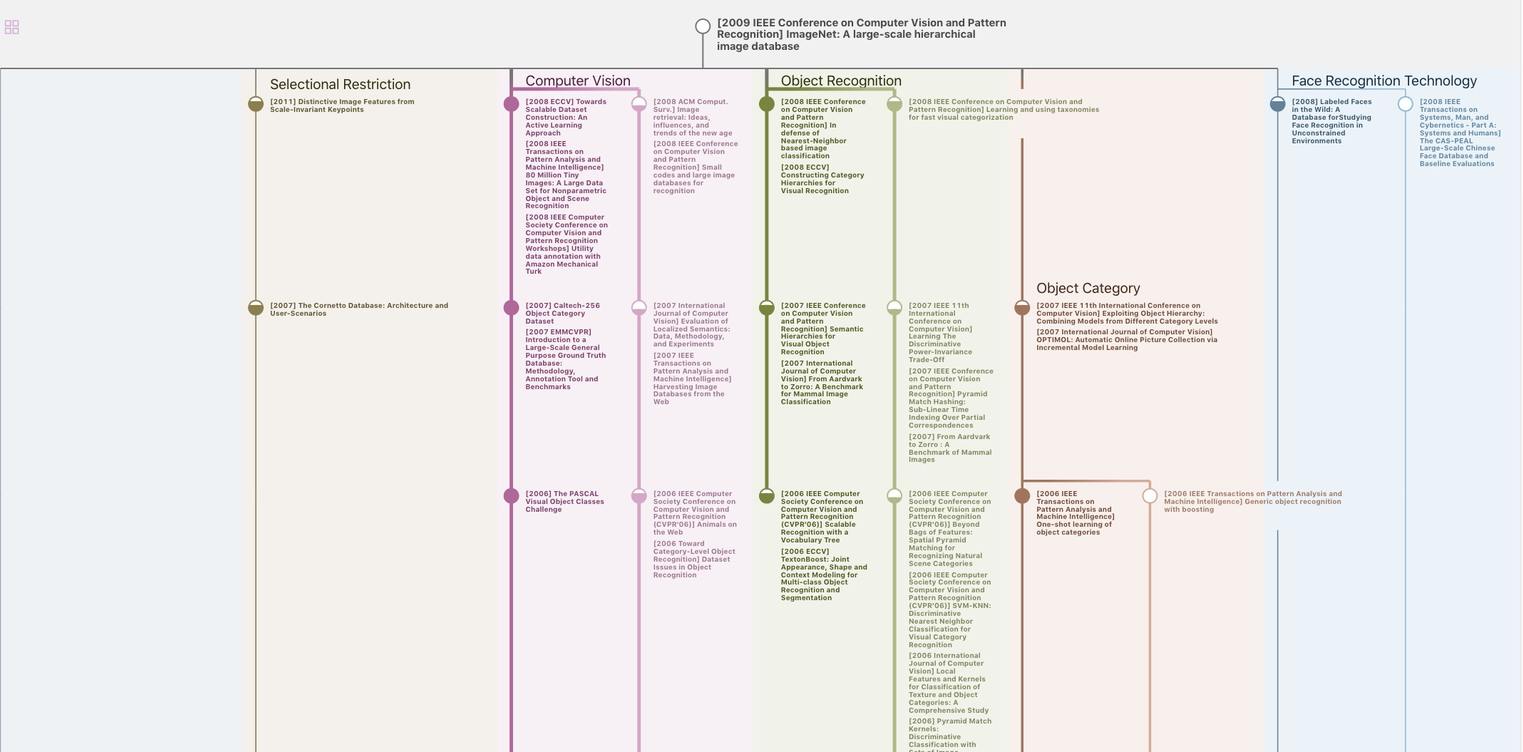
生成溯源树,研究论文发展脉络
Chat Paper
正在生成论文摘要