Verifying Performance Properties of Probabilistic Inference
CoRR(2023)
摘要
In this extended abstract, we discuss the opportunity to formally verify that inference systems for probabilistic programming guarantee good performance. In particular, we focus on hybrid inference systems that combine exact and approximate inference to try to exploit the advantages of each. Their performance depends critically on a) the division between exact and approximate inference, and b) the computational resources consumed by exact inference. We describe several projects in this direction. Semi-symbolic Inference (SSI) is a type of hybrid inference system that provides limited guarantees by construction on the exact/approximate division. In addition to these limited guarantees, we also describe ongoing work to extend guarantees to a more complex class of programs, requiring a program analysis to ensure the guarantees. Finally, we also describe work on verifying that inference systems using delayed sampling -- another type of hybrid inference -- execute in bounded memory. Together, these projects show that verification can deliver the performance guarantees that probabilistic programming languages need.
更多查看译文
关键词
probabilistic inference,performance properties
AI 理解论文
溯源树
样例
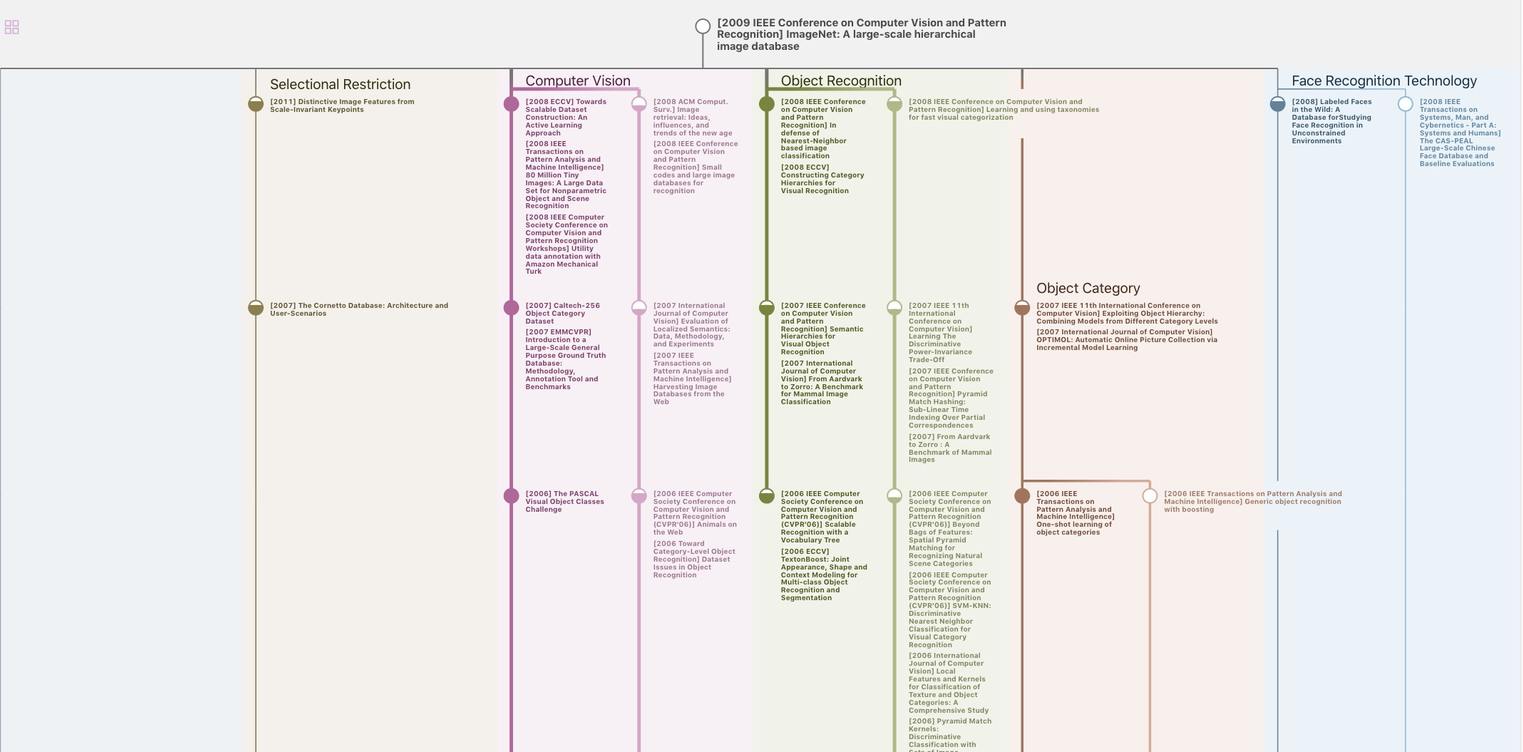
生成溯源树,研究论文发展脉络
Chat Paper
正在生成论文摘要