Long Short-term Memory with Two-Compartment Spiking Neuron
CoRR(2023)
摘要
The identification of sensory cues associated with potential opportunities and dangers is frequently complicated by unrelated events that separate useful cues by long delays. As a result, it remains a challenging task for state-of-the-art spiking neural networks (SNNs) to identify long-term temporal dependencies since bridging the temporal gap necessitates an extended memory capacity. To address this challenge, we propose a novel biologically inspired Long Short-Term Memory Leaky Integrate-and-Fire spiking neuron model, dubbed LSTM-LIF. Our model incorporates carefully designed somatic and dendritic compartments that are tailored to retain short- and long-term memories. The theoretical analysis further confirms its effectiveness in addressing the notorious vanishing gradient problem. Our experimental results, on a diverse range of temporal classification tasks, demonstrate superior temporal classification capability, rapid training convergence, strong network generalizability, and high energy efficiency of the proposed LSTM-LIF model. This work, therefore, opens up a myriad of opportunities for resolving challenging temporal processing tasks on emerging neuromorphic computing machines.
更多查看译文
关键词
memory,long,short-term,two-compartment
AI 理解论文
溯源树
样例
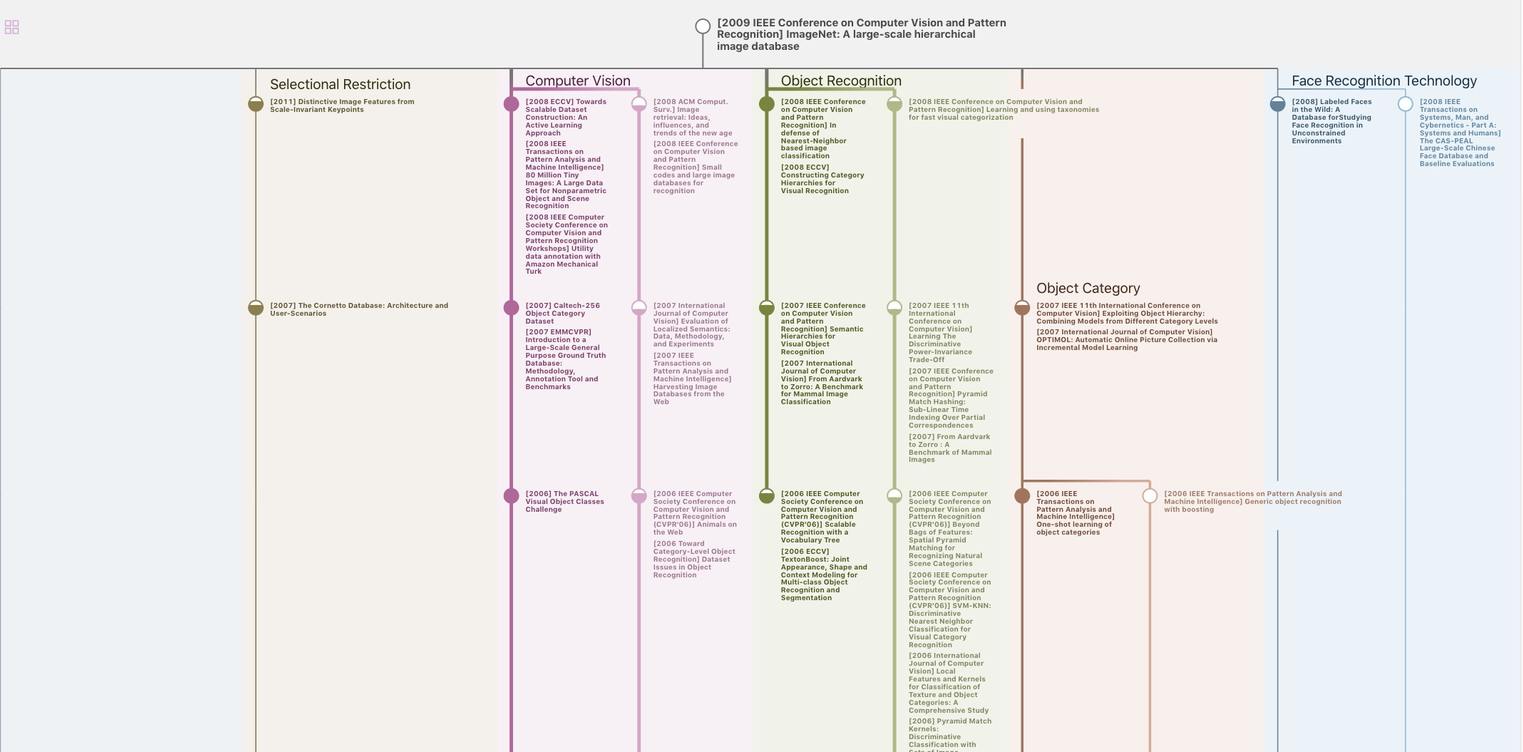
生成溯源树,研究论文发展脉络
Chat Paper
正在生成论文摘要