A Surrogate Data Assimilation Model for the Estimation of Dynamical System in a Limited Area.
CoRR(2023)
摘要
We propose a novel learning-based surrogate data assimilation (DA) model for efficient state estimation in a limited area. Our model employs a feedforward neural network for online computation, eliminating the need for integrating high-dimensional limited-area models. This approach offers significant computational advantages over traditional DA algorithms. Furthermore, our method avoids the requirement of lateral boundary conditions for the limited-area model in both online and offline computations. The design of our surrogate DA model is built upon a robust theoretical framework that leverages two fundamental concepts: observability and effective region. The concept of observability enables us to quantitatively determine the optimal amount of observation data necessary for accurate DA. Meanwhile, the concept of effective region substantially reduces the computational burden associated with computing observability and generating training data.
更多查看译文
关键词
surrogate data assimilation model,estimation,dynamical
AI 理解论文
溯源树
样例
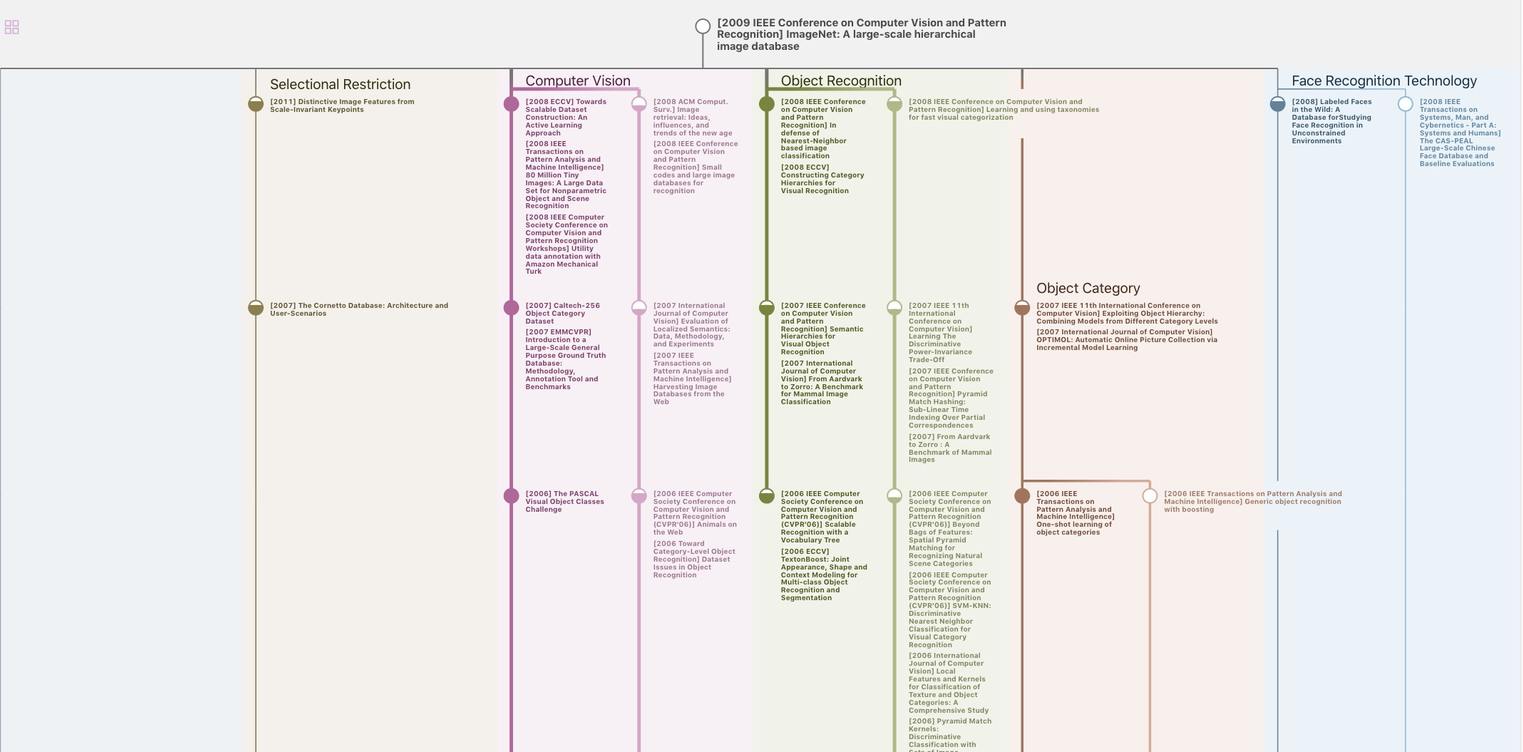
生成溯源树,研究论文发展脉络
Chat Paper
正在生成论文摘要