Approximating Edit Distance in the Fully Dynamic Model
2023 IEEE 64TH ANNUAL SYMPOSIUM ON FOUNDATIONS OF COMPUTER SCIENCE, FOCS(2023)
摘要
The edit distance is a fundamental measure of sequence similarity, defined as the minimum number of character insertions, deletions, and substitutions needed to transform one string into the other. Given two strings of length at most n, a simple dynamic programming computes their edit distance exactly in O(n(2)) time, which is also the best possible (up to subpolynomial factors) assuming the Strong Exponential Time Hypothesis (SETH). The last few decades have seen tremendous progress in edit distance approximation, where the runtime has been brought down to subquadratic, to near-linear, and even to sublinear at the cost of approximation. In this paper, we study the dynamic edit distance problem where the strings change dynamically as the characters are substituted, inserted, or deleted over time. Each change may happen at any location of either of the two strings. The goal is to maintain the (exact or approximate) edit distance of such dynamic strings while minimizing the update time. The exact edit distance can be maintained in O(n log(2) n) time per update (Charalampopoulos, Kociumaka, Mozes; 2020), which is again tight assuming SETH. Unfortunately, even with the unprecedented progress in edit distance approximation in the static setting, strikingly little is known regarding dynamic edit distance approximation. Utilizing the best near-linear-time (Andoni, Nosatzki; 2020) and sublinear-time (Goldenberg, Kociumaka, Krauthgamer, Saha; 2022) approximation algorithm, an old exact algorithm (Landau and Vishkin; 1988), and a generic dynamic strings implementation (Mehlhorn, Sundar, Uhrig; 1996), it is possible to achieve an O(n(c))-approximation in n(0.5-c+o(1)) update time for any constant c is an element of [0, 1/6]. Improving upon this trade-off, characterized by the approximation-ratio and update-time product n(0.5+o(1)), remains wide open. The contribution of this work is a dynamic n(o(1))-approximation algorithm with amortized expected update time of n(o(1)). In other words, we bring the approximation-ratio and update-time product down to n(o(1)), which is also the best possible with the current state of the art in static algorithms. Our solution utilizes an elegant framework of precision sampling trees for edit distance approximation (Andoni, Krauthgamer, Onak; 2010). We show how to dynamically maintain precision sampling trees, which comes with significant nontriviality and can be an independent tool of interest for further development in dynamic string algorithms.
更多查看译文
关键词
edit distance,dynamic algorithms,approximation
AI 理解论文
溯源树
样例
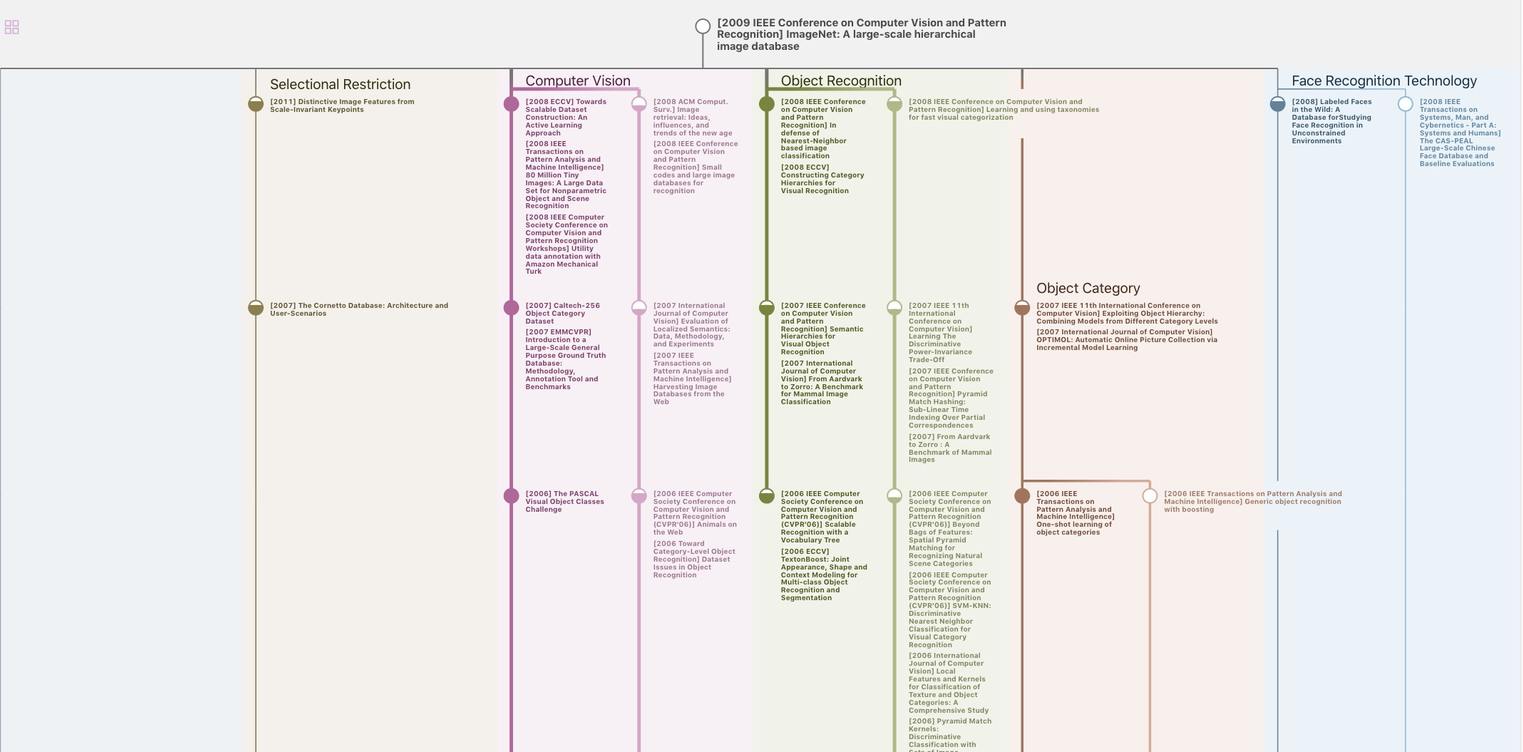
生成溯源树,研究论文发展脉络
Chat Paper
正在生成论文摘要