Drive Like a Human: Rethinking Autonomous Driving with Large Language Models
2024 IEEE/CVF Winter Conference on Applications of Computer Vision Workshops (WACVW)(2023)
摘要
In this paper, we explore the potential of using a large language model (LLM) to understand the driving environment in a human-like manner and analyze its ability to reason, interpret, and memorize when facing complex scenarios. We argue that traditional optimization-based and modular autonomous driving (AD) systems face inherent performance limitations when dealing with long-tail corner cases. To address this problem, we propose that an ideal AD system should drive like a human, accumulating experience through continuous driving and using common sense to solve problems. To achieve this goal, we identify three key abilities necessary for an AD system: reasoning, interpretation, and memorization. We demonstrate the feasibility of employing an LLM in driving scenarios by building a closed-loop system to showcase its comprehension and environment-interaction abilities. Our extensive experiments show that the LLM exhibits the impressive ability to reason and solve long-tailed cases, providing valuable insights for the development of human-like autonomous driving. The related code are available at https://github.com/PJLab-ADG/DriveLikeAHuman .
更多查看译文
关键词
large language
models,autonomous driving,large language
AI 理解论文
溯源树
样例
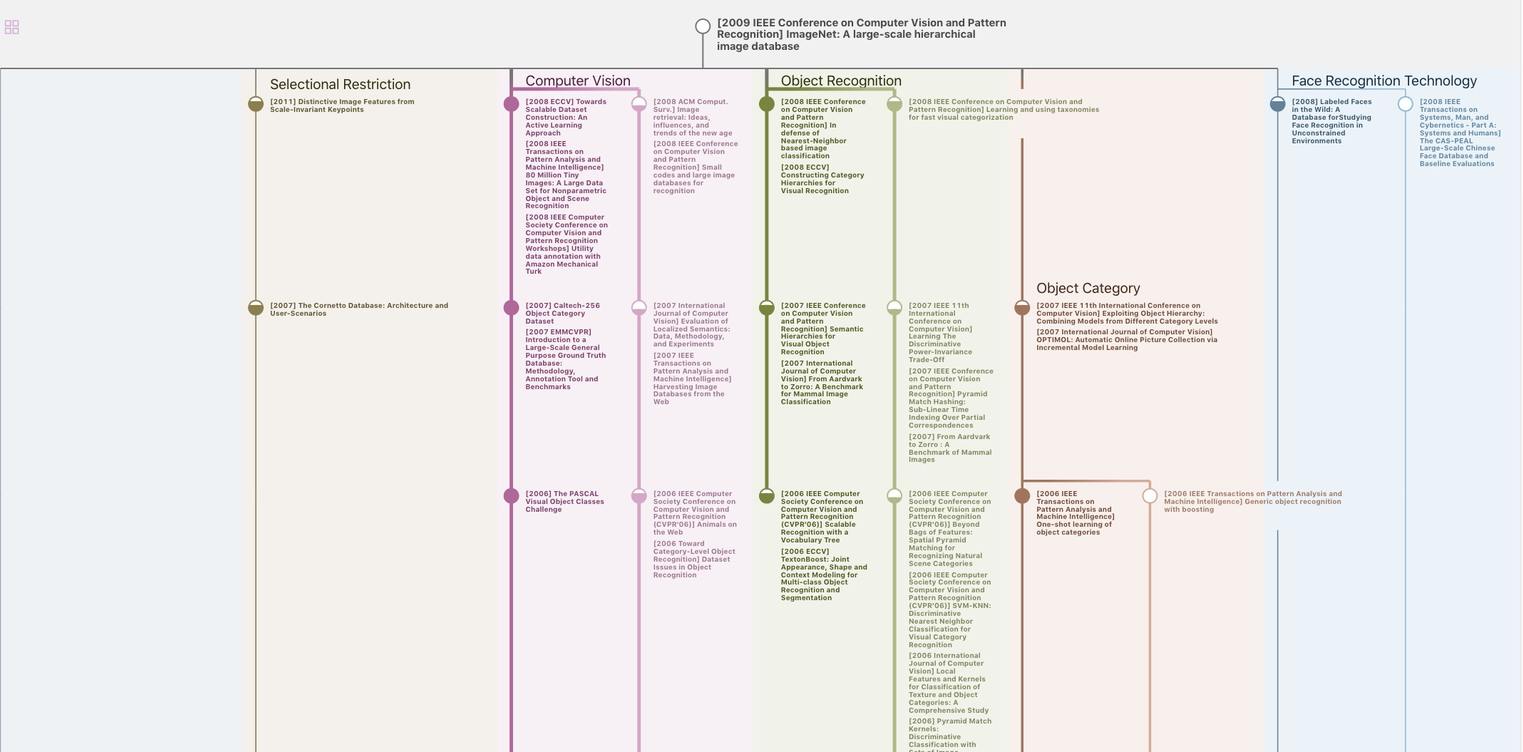
生成溯源树,研究论文发展脉络
Chat Paper
正在生成论文摘要