Building Critical Testing Scenarios for Autonomous Driving from Real Accidents
PROCEEDINGS OF THE 32ND ACM SIGSOFT INTERNATIONAL SYMPOSIUM ON SOFTWARE TESTING AND ANALYSIS, ISSTA 2023(2023)
摘要
One of the aims of the development and spread of autonomous driving technology is to reduce traffic accidents caused by human factors. But recently reported data on fatal accidents involving autonomous driving system (ADS) shows that this important goal has not been achieved. So there is an emerge requirement on more comprehensive and targeted testing especially on safe driving. In this paper, we propose an approach to automatically building critical testing scenarios from real-world accident data. Firstly, we propose a new model called M-CPS (Multi-channel Panoptic Segmentation) to extract the effective information from the accident record (such as images or videos), and separate the independent individuals of different traffic participants for further scene recovery. Compared with the traditional panoramic segmentation models, M-CPS model is able to effectively handle segmentation challenges due to the shooting angle, image quality, pixel overlap and other problems existing in the accident record. Next, the extracted core information is then connected with the virtual testing platform to generate the original scene set. Besides, we also design a mutation testing solution on the basis of the original scene set, thus greatly enriching the scene library for testing. In our experiments, the M-CPS model reaches a result of 66.1% PQ on CityScapes test set, shows that our model has only slight fluctuations on performance compared with the best benchmark model on pure panoptic segmentation task. It also reaches a result of 84.5% IoU for semantic segmentation branch and 40.3% mAP for instance segmentation branch on SHIFT dataset. Then we use UCF-Crime, CADP and US-Accidents datasets to generate the original and mutated scene set. Those generated scene sets are connected to Apollo and Carla simulation platforms to test ADS prototypes. We find three types of scenarios that can lead to accidents of ADS prototypes, which indicates that the existing ADS prototype has defects. Our solution provides a new possible direction for the recovery of key scenarios in ADS testing, and can improve the efficiency in related fields.
更多查看译文
关键词
Autonomous Driving Systems,Panoptic Segmentation,Testing,Parameter Mutation,Scene Recovery
AI 理解论文
溯源树
样例
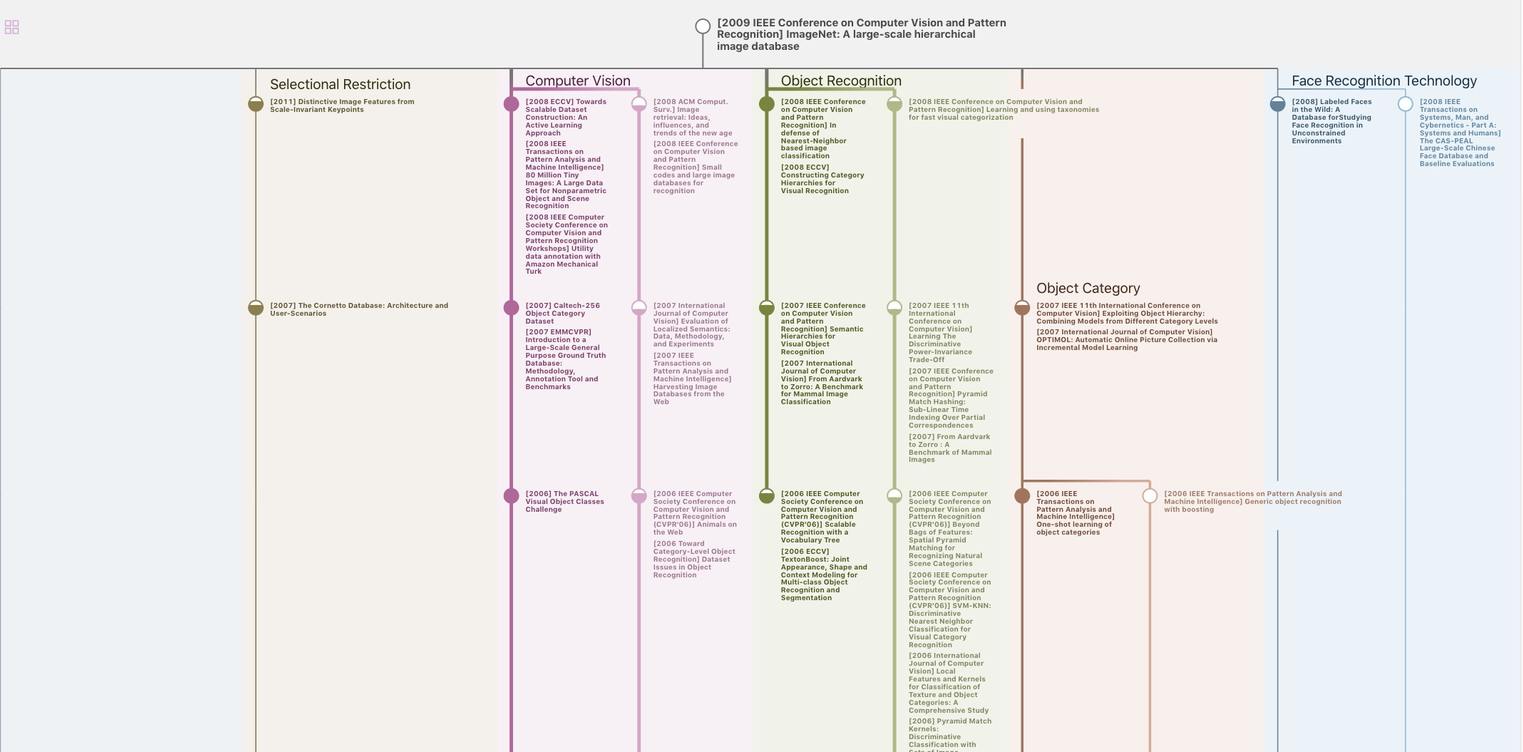
生成溯源树,研究论文发展脉络
Chat Paper
正在生成论文摘要