DeUEDroid: Detecting Underground Economy Apps Based on UTG Similarity
PROCEEDINGS OF THE 32ND ACM SIGSOFT INTERNATIONAL SYMPOSIUM ON SOFTWARE TESTING AND ANALYSIS, ISSTA 2023(2023)
摘要
In recent years, the underground economy is proliferating in the mobile system. These underground economy apps (UEware for short) make profits from providing non-compliant services, especially in sensitive areas (e.g., gambling, porn, loan). Unlike traditional malware, most of them (over 80%) do not have malicious payloads. Due to their unique characteristics, existing detection approaches cannot effectively and efficiently mitigate this emerging threat. To address this problem, we propose a novel approach to effectively and efficiently detect UEware by considering their UI transition graphs (UTGs). Based on the proposed approach, we design and implement a system, named DeUEDroid, to perform the detection. To evaluate DeUEDroid, we collect 25, 717 apps and build up the first large-scale ground-truth dataset (1, 700 apps) of UEware. The evaluation result based on the ground-truth dataset shows that DeUEDroid can cover new UI features and statically construct precise UTG. It achieves 98.22% detection F1-score and 98.97% classification accuracy, a significantly better performance than the traditional approaches. The evaluation result involving 24, 017 apps demonstrates the effectiveness and efficiency of UEware detection in real-world scenarios. Furthermore, the result also reveals that UEware are prevalent, i.e., 54% apps in the wild and 11% apps in the app stores are UEware. Our work sheds light on the future work of analyzing and detecting UEware. To engage the community, we have made our prototype system and the dataset available online.
更多查看译文
关键词
Underground economy,UI transition graph,Machine learning
AI 理解论文
溯源树
样例
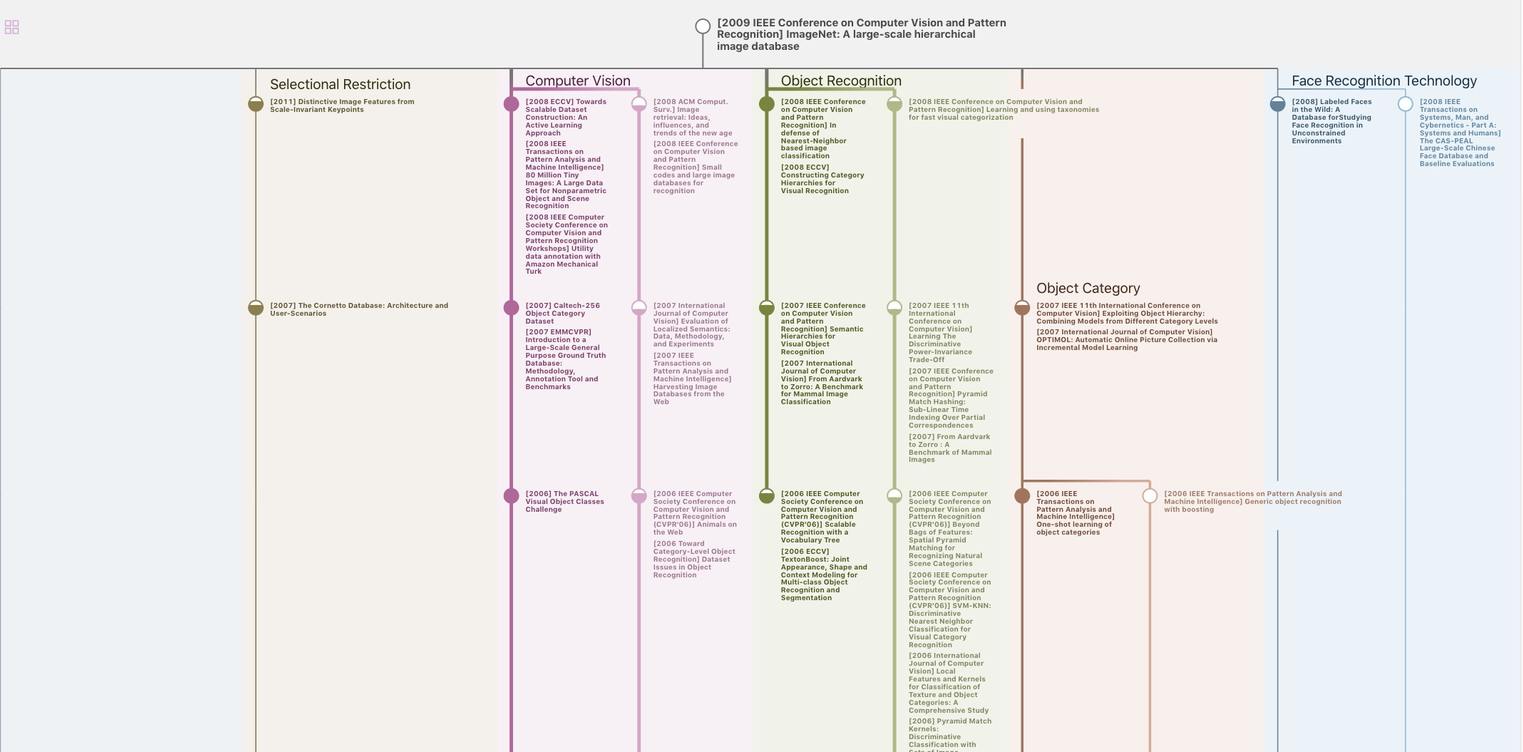
生成溯源树,研究论文发展脉络
Chat Paper
正在生成论文摘要