Fully automated ai-generated models predict post-operative glomerular filtration rate after renal surgery with similar accuracy to a validated clinical model.
The Journal of Urology(2023)
摘要
You have accessJournal of UrologyCME1 Apr 2023PD43-06 FULLY AUTOMATED AI-GENERATED MODELS PREDICT POST-OPERATIVE GLOMERULAR FILTRATION RATE AFTER RENAL SURGERY WITH SIMILAR ACCURACY TO A VALIDATED CLINICAL MODEL. Nour Abdallah, Tarik Benidir, Nicholas Heller, Andrew Wood, Fabian Isensee, Resha Tejpaul, Chalairat Suk-Ouichai, Nityam Rathi, Diego Aguilar Palacios, Alex You, Erick Remer, Jihad Kaouk, Samuel Haywood, Venkatesh Kirshnamurthi, Steven Campbell, Nikolaos Papanikolopoulos, and Christopher Weight Nour AbdallahNour Abdallah More articles by this author , Tarik BenidirTarik Benidir More articles by this author , Nicholas HellerNicholas Heller More articles by this author , Andrew WoodAndrew Wood More articles by this author , Fabian IsenseeFabian Isensee More articles by this author , Resha TejpaulResha Tejpaul More articles by this author , Chalairat Suk-OuichaiChalairat Suk-Ouichai More articles by this author , Nityam RathiNityam Rathi More articles by this author , Diego Aguilar PalaciosDiego Aguilar Palacios More articles by this author , Alex YouAlex You More articles by this author , Erick RemerErick Remer More articles by this author , Jihad KaoukJihad Kaouk More articles by this author , Samuel HaywoodSamuel Haywood More articles by this author , Venkatesh KirshnamurthiVenkatesh Kirshnamurthi More articles by this author , Steven CampbellSteven Campbell More articles by this author , Nikolaos PapanikolopoulosNikolaos Papanikolopoulos More articles by this author , and Christopher WeightChristopher Weight More articles by this author View All Author Informationhttps://doi.org/10.1097/JU.0000000000003353.06AboutPDF ToolsAdd to favoritesDownload CitationsTrack CitationsPermissionsReprints ShareFacebookLinked InTwitterEmail Abstract INTRODUCTION AND OBJECTIVE: The American Urologic Association recommends estimating postoperative (postop) glomerular filtration rate (GFR) in patients with renal mass to prioritize partial (PN) over radical nephrectomy (RN) when postop GFR<45 mL/min/1.73m2. Validated models based on clinical equations or renal volumes from hand or semi-automated segmentations are quite accurate but have limited uptake in clinical practice. We aimed to develop an artificial intelligence (AI)-GFR prediction calculated automatically on a preoperative (preop) computed tomography (CT) scan to predict a postop GFR as accurately as a validated clinical model. METHODS: Three hundred patients having PN or RN for renal tumor from the KiTS21 challenge were analyzed. We excluded 7 patients having bilateral tumors. Preop GFR was the closest recorded value, and postop GFR was ≥90 days. Split-renal function (SRF) was determined in a fully automated way from preop CT and our deep learning segmentation model. We programmed the algorithm to estimate postop GFR as 1.24×preop GFR×contralateral SRF for RN and 89% of preop GFR for PN. We compared AI-predicted GFR to a validated clinical model (GFR=35+preop GFR (x0.65)-18 (if RN) -age (x0.25)+3 (if tumor size>7 cm)-2 (if diabetes)). We compared AI and clinical model estimations of GFR to the measured postop GFR using correlation coefficients (R) and compared their ability to predict postop GFR<45 using logistic regression and areas under the curves (AUC). RESULTS: In 293 patients, median age was 60 years ((IQR) 51-68), 40.6% were female, and 62.1% had PN. Median tumor size was 4.2 (2.6-6.1), and 91.8% of the tumors were malignant, of which 35.1% were high-grade, 25.6% high-stage, and 21.8% had necrosis. When comparing measured postop GFR, the correlation coefficients were 0.75 and 0.77 for AI and clinical models, respectively. For the prediction of postop GFR<45, the AI and clinical models performed similarly (AUC 0.89 and 0.9, respectively). CONCLUSIONS: Our study introduces a fully automated prediction of postop GFR based on CT and baseline GFR with comparable predictive accuracy to validated clinical prediction models. These AI-based predictions can be implemented for decision-making without clinical details, clinician time, or measurements needed. Source of Funding: None © 2023 by American Urological Association Education and Research, Inc.FiguresReferencesRelatedDetails Volume 209Issue Supplement 4April 2023Page: e1120 Advertisement Copyright & Permissions© 2023 by American Urological Association Education and Research, Inc.MetricsAuthor Information Nour Abdallah More articles by this author Tarik Benidir More articles by this author Nicholas Heller More articles by this author Andrew Wood More articles by this author Fabian Isensee More articles by this author Resha Tejpaul More articles by this author Chalairat Suk-Ouichai More articles by this author Nityam Rathi More articles by this author Diego Aguilar Palacios More articles by this author Alex You More articles by this author Erick Remer More articles by this author Jihad Kaouk More articles by this author Samuel Haywood More articles by this author Venkatesh Kirshnamurthi More articles by this author Steven Campbell More articles by this author Nikolaos Papanikolopoulos More articles by this author Christopher Weight More articles by this author Expand All Advertisement PDF downloadLoading ...
更多查看译文
关键词
renal surgery,validated clinical models,models predict,ai-generated,post-operative
AI 理解论文
溯源树
样例
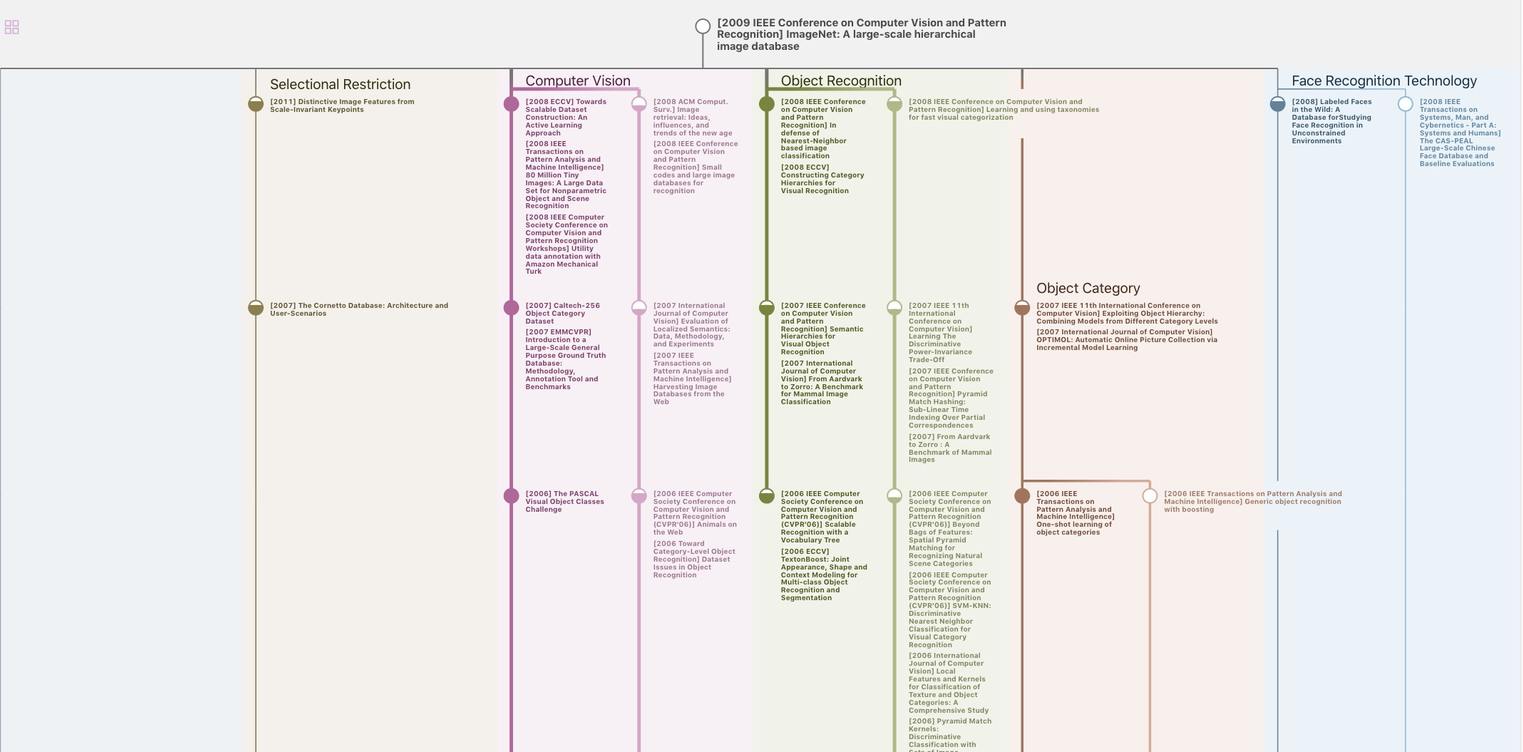
生成溯源树,研究论文发展脉络
Chat Paper
正在生成论文摘要