Appraisal of data-driven techniques for predicting short-term streamflow in tropical catchment.
Water science and technology : a journal of the International Association on Water Pollution Research(2023)
摘要
Short-term streamflow prediction is essential for managing flood early warning and water resources systems. Although numerical models are widely used for this purpose, they require various types of data and experience to operate the model and often tedious calibration processes. Under the digital revolution, the application of data-driven approaches to predict streamflow has increased in recent decades. In this work, multiple linear regression (MLR) and random forest (RF) models with three different input combinations are developed and assessed for multi-step ahead short-term streamflow predictions, using 14 years of hydrological datasets from the Kulim River catchment, Malaysia. Introducing more precedent streamflow events as predictor improves the performance of these data-driven models, especially in predicting peak streamflow during the high-flow event. The RF model (Nash-Sutcliffe efficiency (NSE): 0.599-0.962) outperforms the MLR model (NSE: 0.584-0.963) in terms of overall prediction accuracy. However, with the increasing lead-time length, the models' overall prediction accuracy on the arrival time and magnitude of peak streamflow decrease. These findings demonstrate the potential of decision tree-based models, such as RF, for short-term streamflow prediction and offer insights into enhancing the accuracy of these data-driven models.
更多查看译文
关键词
climate change, data-driven models, Kulim River, multiple linear regression, random forest, short-term streamflow prediction
AI 理解论文
溯源树
样例
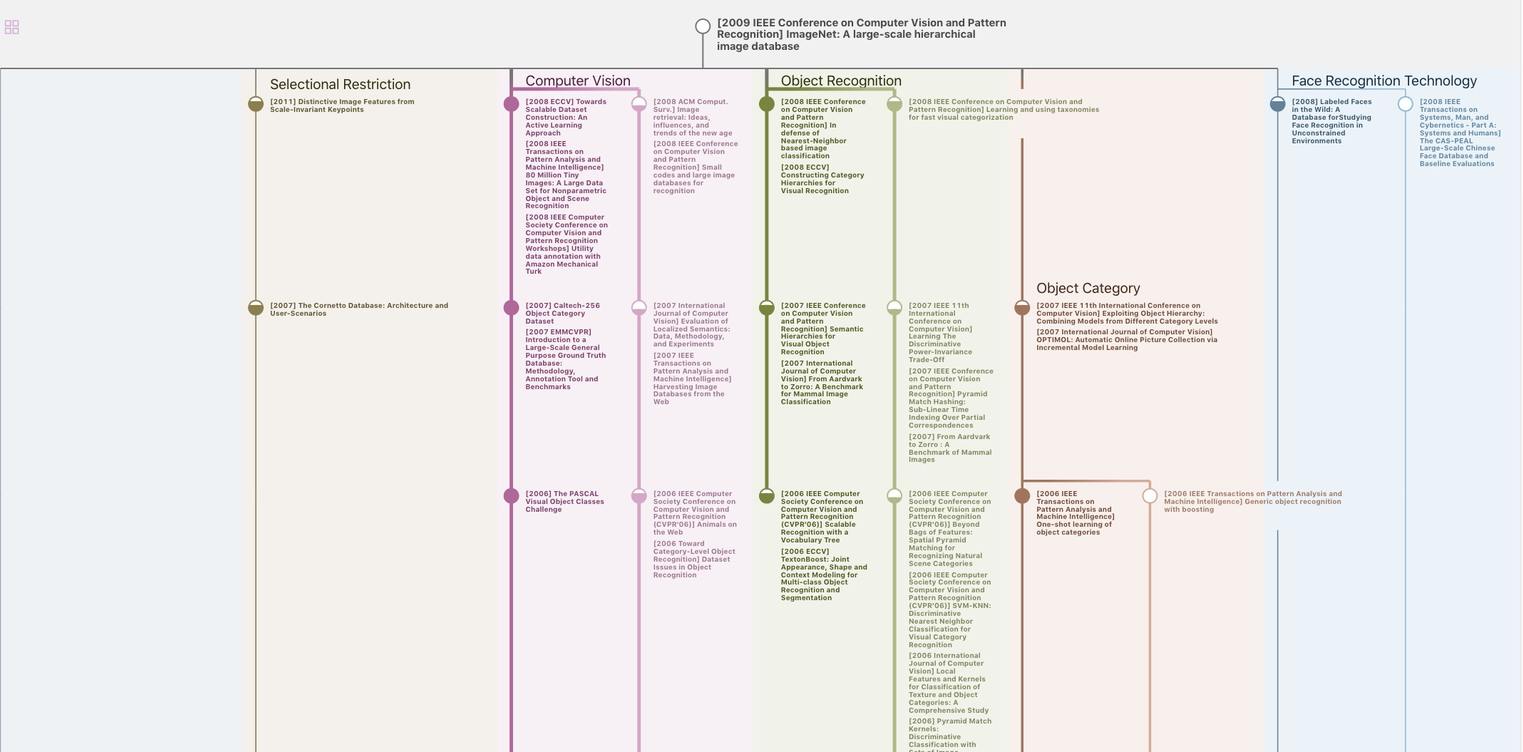
生成溯源树,研究论文发展脉络
Chat Paper
正在生成论文摘要