De novo generation of dual-target ligands for the treatment of SARS-CoV-2 using deep learning, virtual screening, and molecular dynamic simulations
JOURNAL OF BIOMOLECULAR STRUCTURE & DYNAMICS(2024)
摘要
De novo generation of molecules with the necessary features offers a promising opportunity for artificial intelligence, such as deep generative approaches. However, creating novel compounds having biological activities toward two distinct targets continues to be a very challenging task. In this study, we develop a unique computational framework for the de novo synthesis of bioactive compounds directed at two predetermined therapeutic targets. This framework is referred to as the dual-target ligand generative network. Our approach uses a stochastic policy to explore chemical spaces called a sequence-based simple molecular input line entry system (SMILES) generator. The steps in the high-level workflow would be to gather and prepare the training data for both targets' molecules, build a neural network model and train it to make molecules, create new molecules using generative AI, and then virtually screen the newly validated molecules against the SARS-CoV-2 PLpro and 3CLpro drug targets. Results shows that novel molecules generated have higher binding affinity with both targets than the conventional drug i.e. Remdesivir being used for the treatment of SARS-CoV-2.Communicated by Ramaswamy H. Sarma
更多查看译文
关键词
SARS-CoV-2,PLpro,3CLpro,SMILES,de novo generation,deep generative approaches
AI 理解论文
溯源树
样例
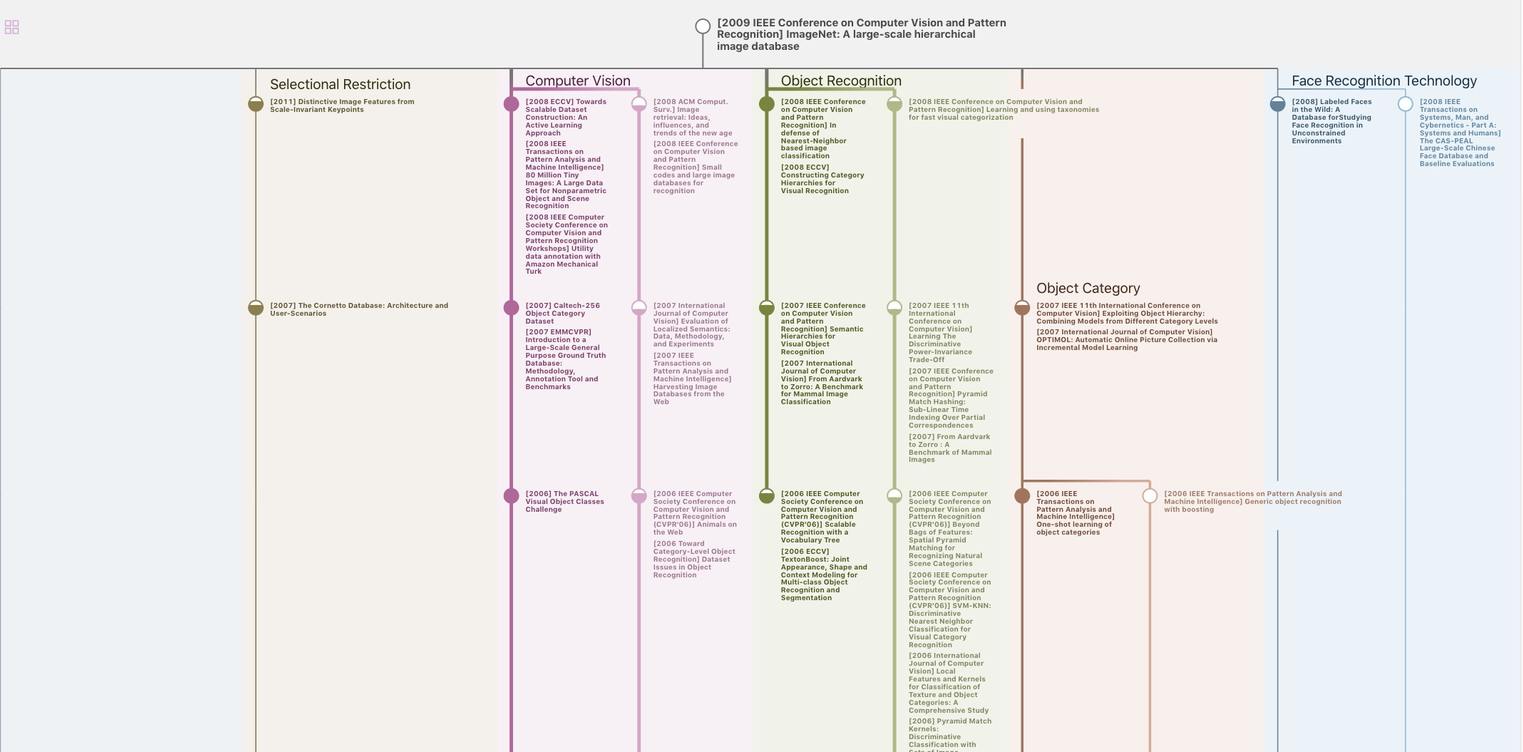
生成溯源树,研究论文发展脉络
Chat Paper
正在生成论文摘要